Event-triggered reconfigurable reinforcement learning motion-planning approach for mobile robot in unknown dynamic environments
ENGINEERING APPLICATIONS OF ARTIFICIAL INTELLIGENCE(2023)
摘要
Deep reinforcement learning (DRL) is an essential technique for autonomous motion planning of mobile robots in dynamic and uncertain environments. In attempting to acquire a satisfactory DRL-based motion planning strategy, the mobile robots encountered several difficulties, including poor convergence, insufficient sample information, and low learning efficiency. These problems not only consume plenty of training time, but also bring a negative impact on motion planning performance. One promising research direction is to provide a more effective network framework for DRL-based policies. Along this line of thinking, our paper presents a novel DRL-based motion planning approach called Reconfigurable Structure of Deep Deterministic Policy Gradient (RS-DDPG) for mobile robots. To account for the poor convergence, the proposed approach first introduces an event-triggered reconfigurable actor-critic network framework for motion policy that adaptively changes its network structure to suppress the overestimation of action value. Then, the time convergence of the motion policy can be enhanced based on the value actions with minor valuation deviation. Afterwards, an adaptive reward mechanism is designed for reconfigurable networks to compensate for the lack of sample information. To deal with the problem of low learning efficiency, we developed a sample pretreatment method for the experience samples, which employs three novel techniques to improve the sample utilization, including a double experience memory buffer, a variable proportional sampling principle, and a similarity judgment mechanism. In extensive experiments, the proposed method outperforms the compared approaches.
更多查看译文
关键词
Mobile robot,Reinforcement learning,Actor–critic,Reconfigurable structure,Sample pretreatment,Event-triggered
AI 理解论文
溯源树
样例
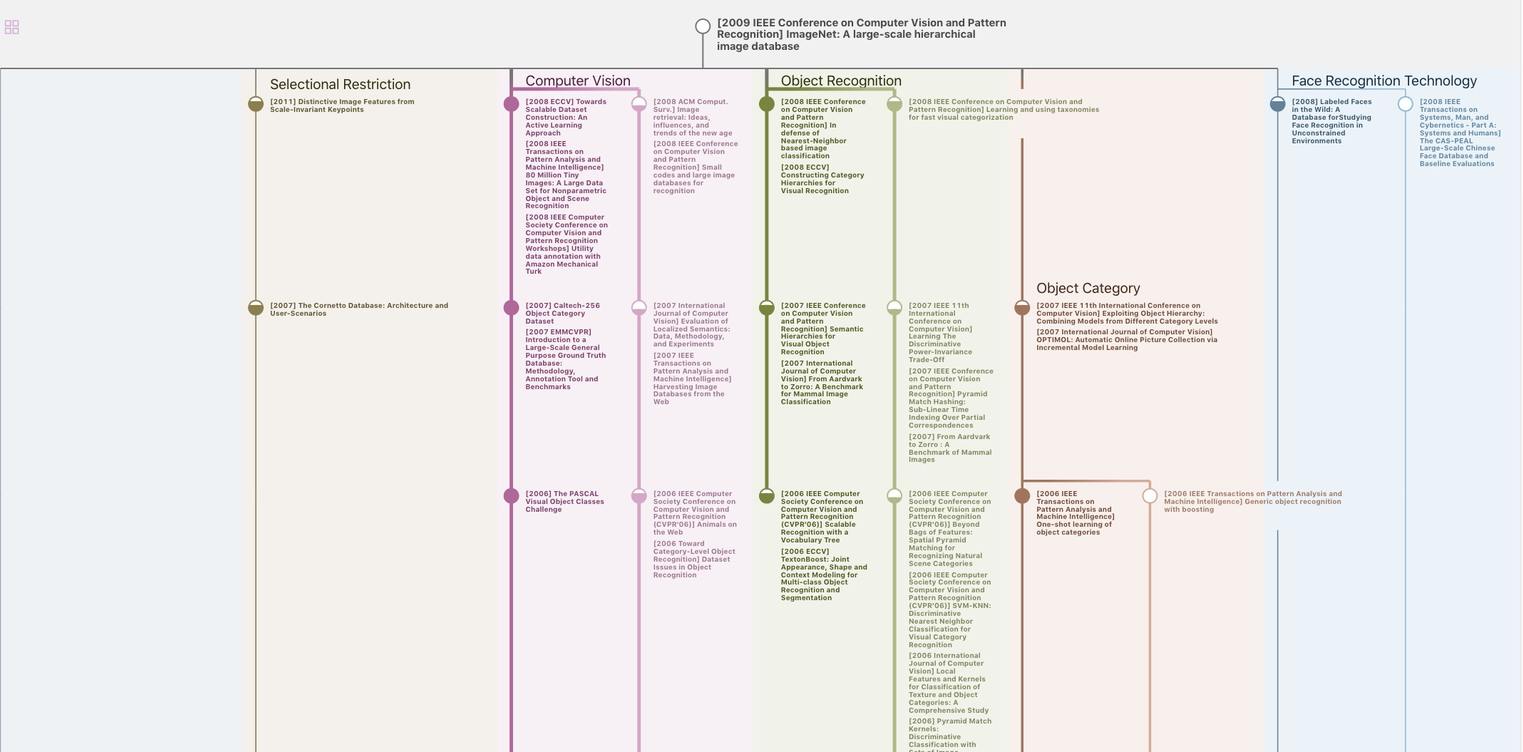
生成溯源树,研究论文发展脉络
Chat Paper
正在生成论文摘要