Differentiate Xp11.2 Translocation Renal Cell Carcinoma from Computed Tomography Images and Clinical Data with ResNet-18 CNN and XGBoost
CMES-COMPUTER MODELING IN ENGINEERING & SCIENCES(2023)
摘要
This study aims to apply ResNet-18 convolutional neural network (CNN) and XGBoost to preoperative computed tomography (CT) images and clinical data for distinguishing Xp11.2 translocation renal cell carcinoma (Xp11.2 tRCC) from common subtypes of renal cell carcinoma (RCC) in order to provide patients with individualized treatment plans. Data from 45 patients with Xp11.2 tRCC from January 2007 to December 2021 are collected. Clear cell RCC (ccRCC), papillary RCC (pRCC), or chromophobe RCC (chRCC) can be detected from each patient. CT images are acquired in the following three phases: unenhanced, corticomedullary, and nephrographic. A unified framework is proposed for the classification of renal masses. In this framework, ResNet-18 CNN is employed to classify renal cancers with CT images, while XGBoost is adopted with clinical data. Experiments demonstrate that, if applying ResNet-18 CNN or XGBoost singly, the latter outperforms the former, while the framework integrating both technologies performs similarly or better than urologists. Especially, the possibility of misclassifying Xp11.2 tRCC, pRCC, and chRCC as ccRCC by the proposed framework is much lower than urologists.
更多查看译文
关键词
computed tomography images,carcinoma
AI 理解论文
溯源树
样例
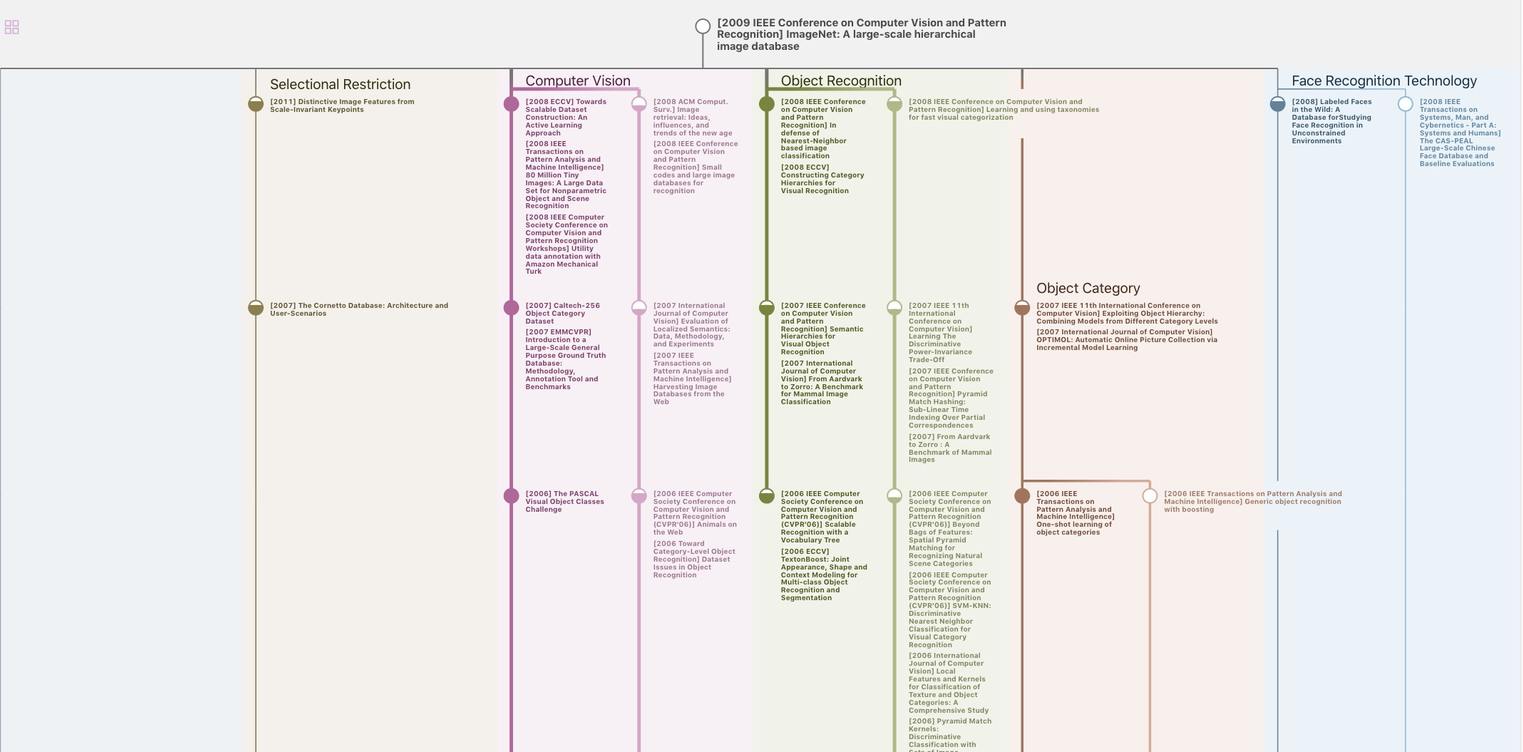
生成溯源树,研究论文发展脉络
Chat Paper
正在生成论文摘要