Enhanced neighborhood node graph neural networks for load forecasting in smart grid
INTERNATIONAL JOURNAL OF MACHINE LEARNING AND CYBERNETICS(2024)
摘要
Deep learning technology creates the condition for the optimization of the smart grid, and the big data analytical technique has the most efficient way to analyze and share the power load spatio-temporal data in the smart grid. Utilizing the graph-based method to learn the structure of load date distribution and load prediction has become hot-spot research. This paper proposes EnGAT-BiLSTM, an enhanced graph neural networks framework to realize short-term load prediction. The EnGAT-BiLSTM model aims to improve the prediction accuracy of the load and solve the sampled data sparsity in the short-term prediction. In this model, the Box-Cox transformation technology is used to denoise and obtain the effective load sampled data set; a dynamic load knowledge graph (DLKG) is designed to map the internal attribute of the various electrical load and the correlation of the external influencing factors; the graphic attention mechanism is introduced in the local network structure of graph neural network (GNN) to extract the high-quality load spatio-temporal features; the deep bi-directional long short-term memory (BiLSTM) framework is used for the lifelong learning of the load prediction. The extensive load-sampled datasets in the real world are employed to evaluate our method. The experimental results indicate that EnGAT-BiLSTM improves significantly in load prediction accuracy and has good robustness. The model will provide a valuable theoretical basis and guidance for the smart grid IoT system.
更多查看译文
关键词
Load power prediction,Lifelong learning,Graph neural network,Box-Cox transformation,Multi-attention mechanism
AI 理解论文
溯源树
样例
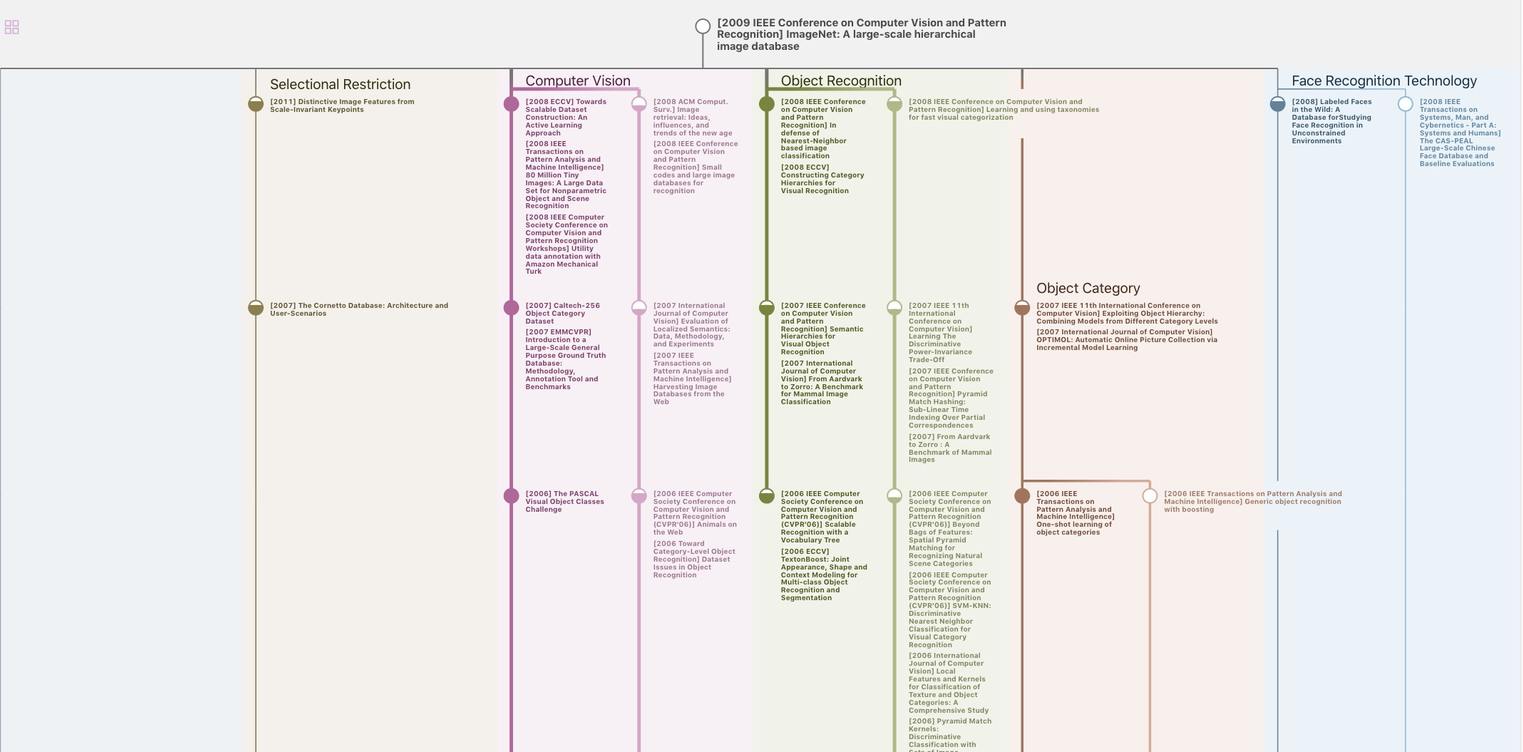
生成溯源树,研究论文发展脉络
Chat Paper
正在生成论文摘要