Data-Driven Distance Metrics for Kriging-Short-Term Urban Traffic State Prediction
IEEE TRANSACTIONS ON INTELLIGENT TRANSPORTATION SYSTEMS(2023)
摘要
Estimating traffic flow states at unmeasured urban locations provides a cost-efficient solution for many ITS applications. In this work, a geostatistical framework, kriging is extended in such a way that it can both estimate and predict traffic volume and speed at various unobserved locations, in real-time. In the paper, different distance metrics for kriging are evaluated. Then, a new, data-driven one is formulated, capturing the similarity of measurement sites. Then, with multidimensional scaling the distances are transformed into a hyperspace, where the kriging algorithm can be used. As a next step, temporal dependency is injected into the estimator via extending the hyperspace with an extra dimension, enabling for short horizon traffic flow prediction. Additionally, a temporal correction is proposed to compensate for minor changes in traffic flow patterns. Numerical results suggest that the spatio-temporal prediction can make more accurate predictions compared to other distance metric-based kriging algorithms. Additionally, compared to deep learning, the results are on par while the algorithm is more resilient against traffic pattern changes.
更多查看译文
关键词
Prediction algorithms, Measurement, Detectors, Kernel, Deep learning, Correlation, Neural networks, Kriging, spatio-temporal prediction, distance metric, traffic flow prediction
AI 理解论文
溯源树
样例
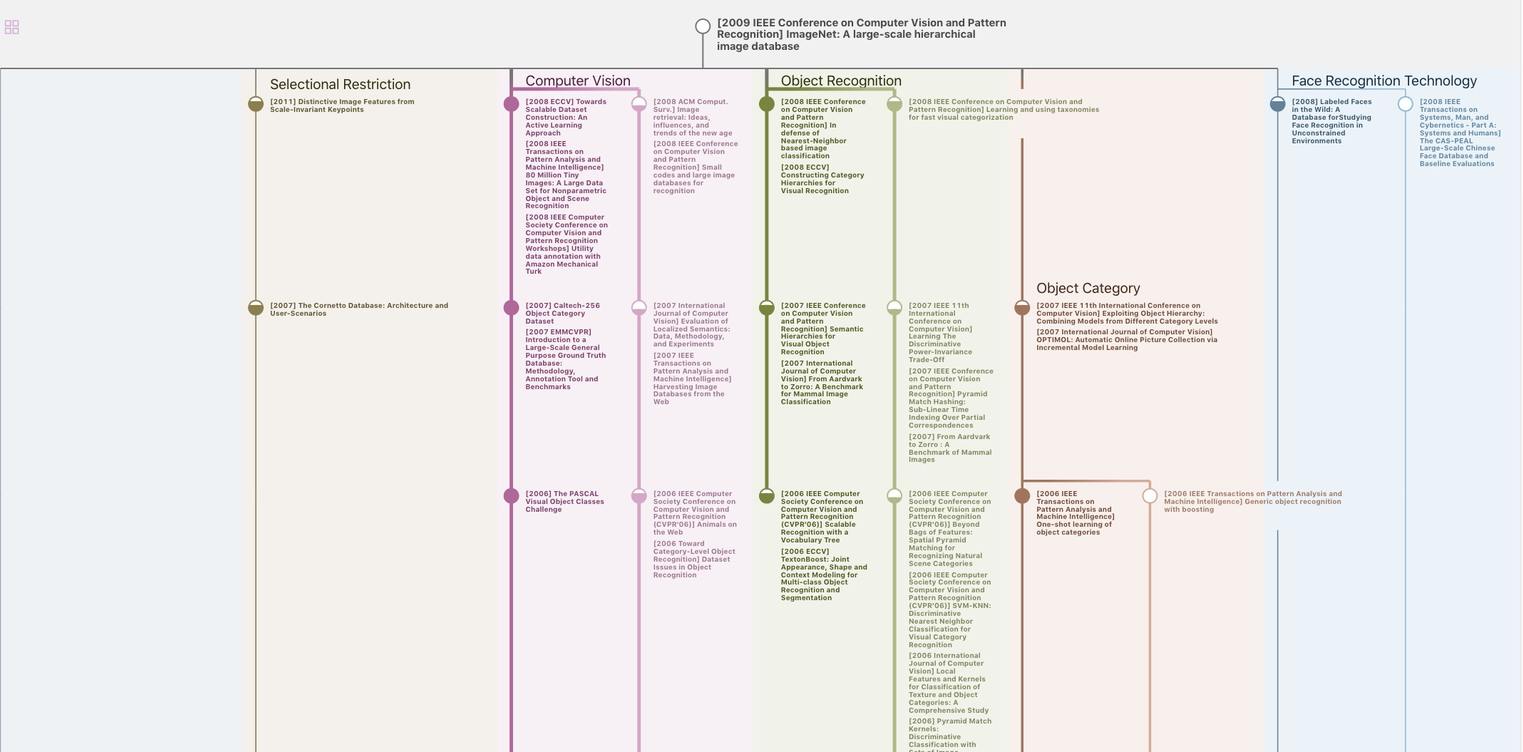
生成溯源树,研究论文发展脉络
Chat Paper
正在生成论文摘要