Online Control of the Raw Water System of a High-Sediment River Based on Deep Reinforcement Learning
WATER(2023)
摘要
Water supply systems that use rivers with high sedimentation levels may experience issues such as reservoir siltation. The suspended sediment concentration (SSC) of rivers experiences interannual variation and high nonlinearity due to its close relationship with meteorological factors, which increase the mismatch between the river water source and urban water demand. The raw water system scheduling problem is expressed as a reservoir and pump station control problem that involves real-time SSC changes. To lower the SSC of the water intake and lower the pumping station's energy consumption, a deep reinforcement learning (DRL) model based on SSC prediction was developed. The framework consists of a DRL model, a hydraulic model for simulating the raw water system, and a neural network for predicting river SSC. The framework was tested using data from a Yellow River water withdrawal pumping station in China with an average capacity of 400,000 m(3)/d. The strategy created in this study can reduce the system energy consumption per unit of water withdrawal by 8.33% and the average annual water withdrawal SSC by 37.01%, when compared to manual strategy. Meanwhile, the deep reinforcement learning algorithm had good response robustness to uncertain imperfect predictive data.
更多查看译文
关键词
water intake pumping station,storage reservoir,deep reinforcement learning,predictive online control,artificial neural network
AI 理解论文
溯源树
样例
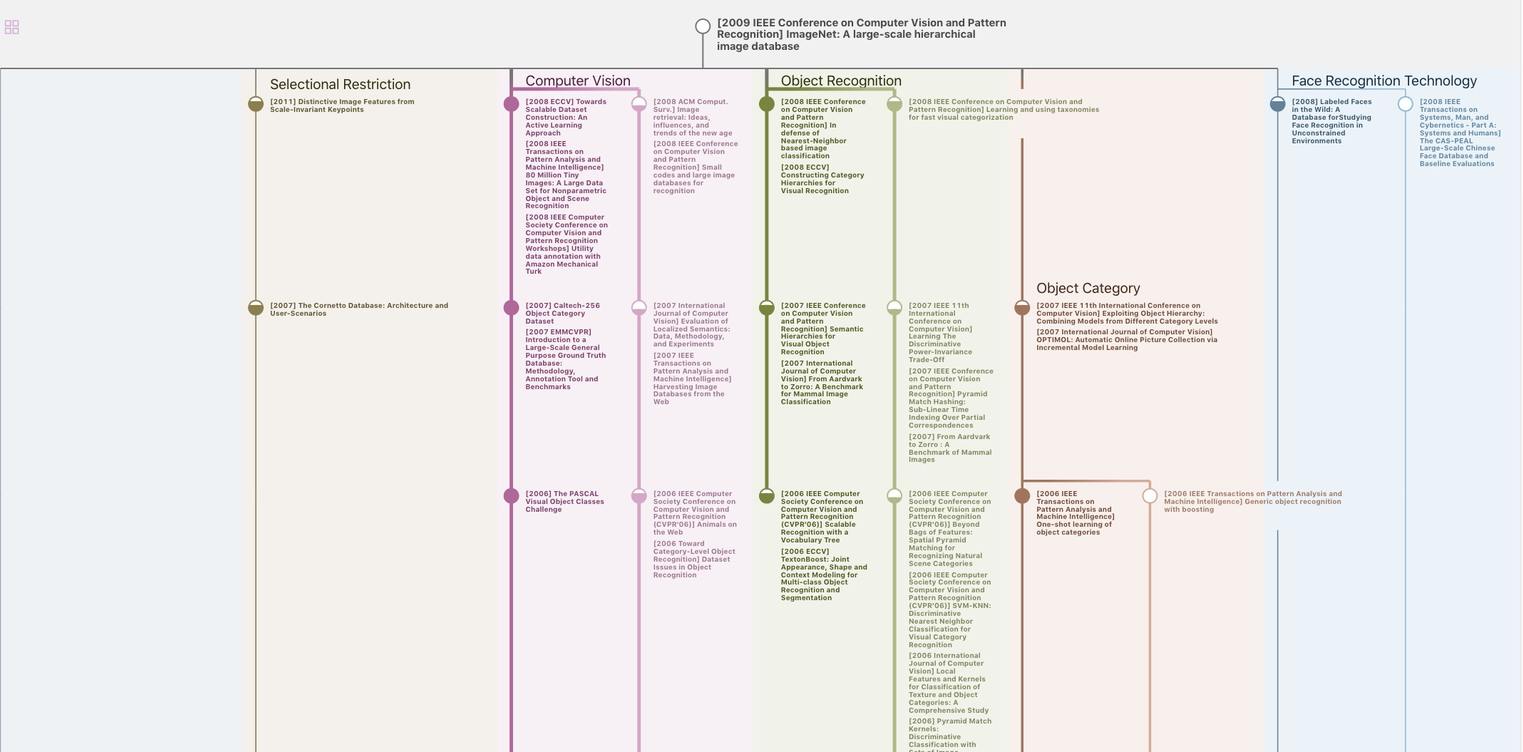
生成溯源树,研究论文发展脉络
Chat Paper
正在生成论文摘要