Remote sensing and machine learning techniques for high throughput phenotyping of late blight-resistant tomato plants in open field trials
INTERNATIONAL JOURNAL OF REMOTE SENSING(2023)
摘要
The selection of late blight resistant genotypes of tomato requires many evaluations on the field. The use of machine learning models to assess late blight severity based on images from multispectral cameras onboard of unmanned aerial vehicles (UAVs) can bring efficiency and quickly in evaluations. In this work, we use remote sensing and machine learning techniques to assess late blight resistance of tomato lines grown in open field conditions. Seventy-six tomato lines, including two resistant lines and one susceptible line, were used to quantify late blight severity. Plants were arranged according to a replicated check design in a total of 132 experimental plots. Tomato plants were artificially inoculated with a Phytophthora infestans zoospore suspension. Multispectral images were obtained using an unmanned aerial vehicle. We calculated vegetation indexes (VI) using the images, which were the basis for building the Random Forest models used to predict disease severity. Two methodologies were used to predict late blight severity: Methodology 1, which used only the images from the last day of evaluation, and Methodology 2, which used the images from four days of evaluation. For Methodology 1 and 2, determination coefficients of 0.81 and 0.93 were obtained for the test set, respectively. Methodology 2 was used to predict late blight severity of the 132 field plots. Tomato plots were sorted from lowest to highest predicted severity. Resistant plots were ranked first indicating consistency of prediction. We therefore recommend Methodology 2 as a fast and practical way to predicted late blight severity in breeding populations.
更多查看译文
关键词
high throughput phenotyping,tomato,remote sensing,plants,blight-resistant
AI 理解论文
溯源树
样例
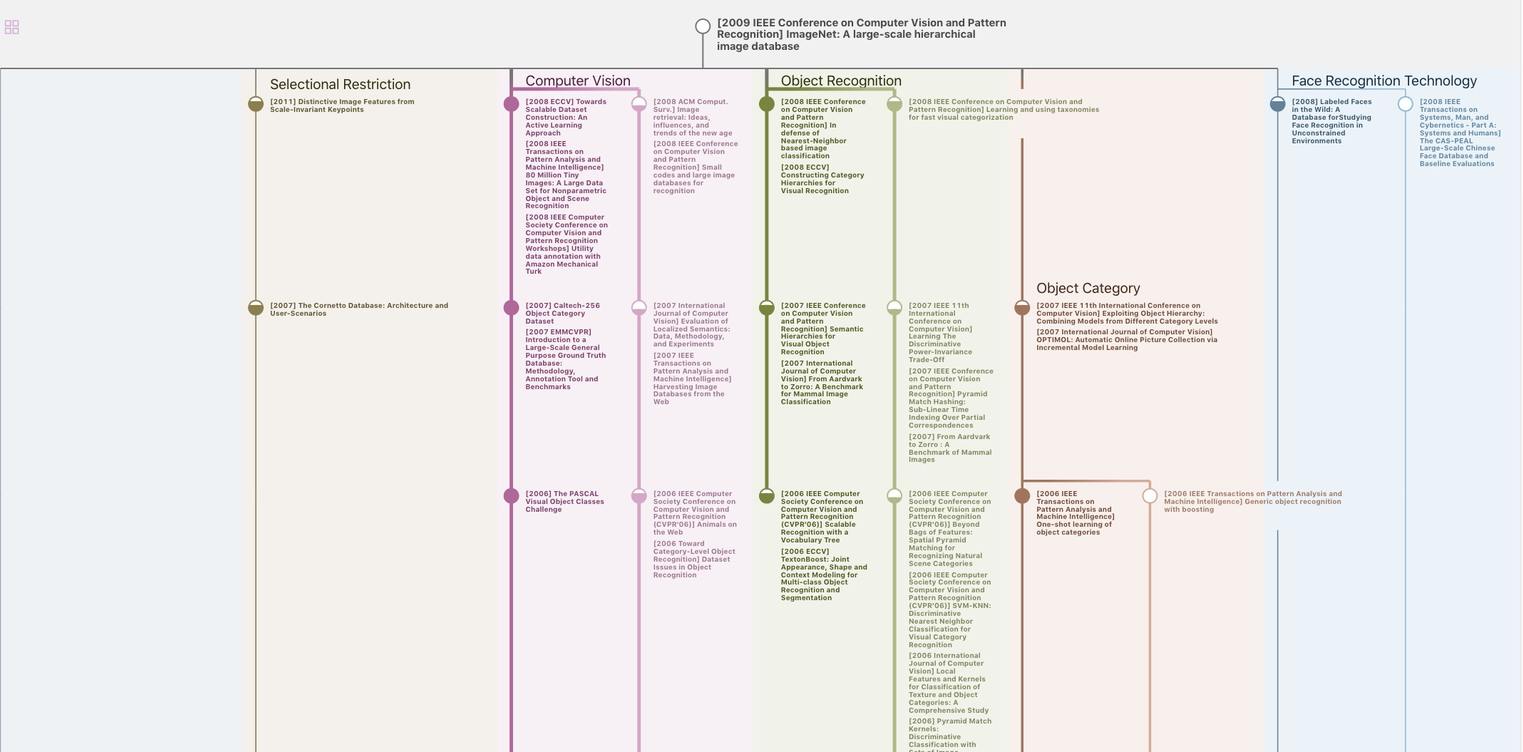
生成溯源树,研究论文发展脉络
Chat Paper
正在生成论文摘要