Balanced incremental deep reinforcement learning based on variational autoencoder data augmentation for customer credit scoring
ENGINEERING APPLICATIONS OF ARTIFICIAL INTELLIGENCE(2023)
摘要
Incremental deep reinforcement learning has been successfully applied to different fields in the real-world. However, training deep reinforcement learning models with the data set generated in a certain stage may lead to catastrophic forgetting of trained models, that is, when training models on the new data set, the performance of models will be seriously degraded on the old data set. Therefore, we construct the balanced incremental deep Q-network based on variational autoencoder data augmentation (BIDQN-VADA) model for customer credit scoring. First, the original training set is processed by the random undersampling method to obtain the balanced training set, in which the initial deep Q-network model is trained. Then, the balanced training subset with a fixed number of samples is selected from the original training set, and these samples are augmented by the variational autoencoder data augmentation technology to obtain the balanced augmented training subset. Finally, the balanced training subset and balanced augmented training subset are stored in data stream cache according to the first-in first-out principle for incrementally updating parameters of the deep Q-network model. In order to verify the performance of the model, we conduct a series of experiments on eight real-world customer credit scoring data sets. The experimental results show that the BIDQN-VADA model can achieve the best customer credit scoring performance by combining the balance, incremental and data augmentation process at the same time. More importantly, the performance of the BIDQN-VADA model is significantly better than the other seven classification models.
更多查看译文
关键词
Incremental deep reinforcement learning,Data augmentation,Customer credit scoring,Variational autoencoder,Deep Q-network
AI 理解论文
溯源树
样例
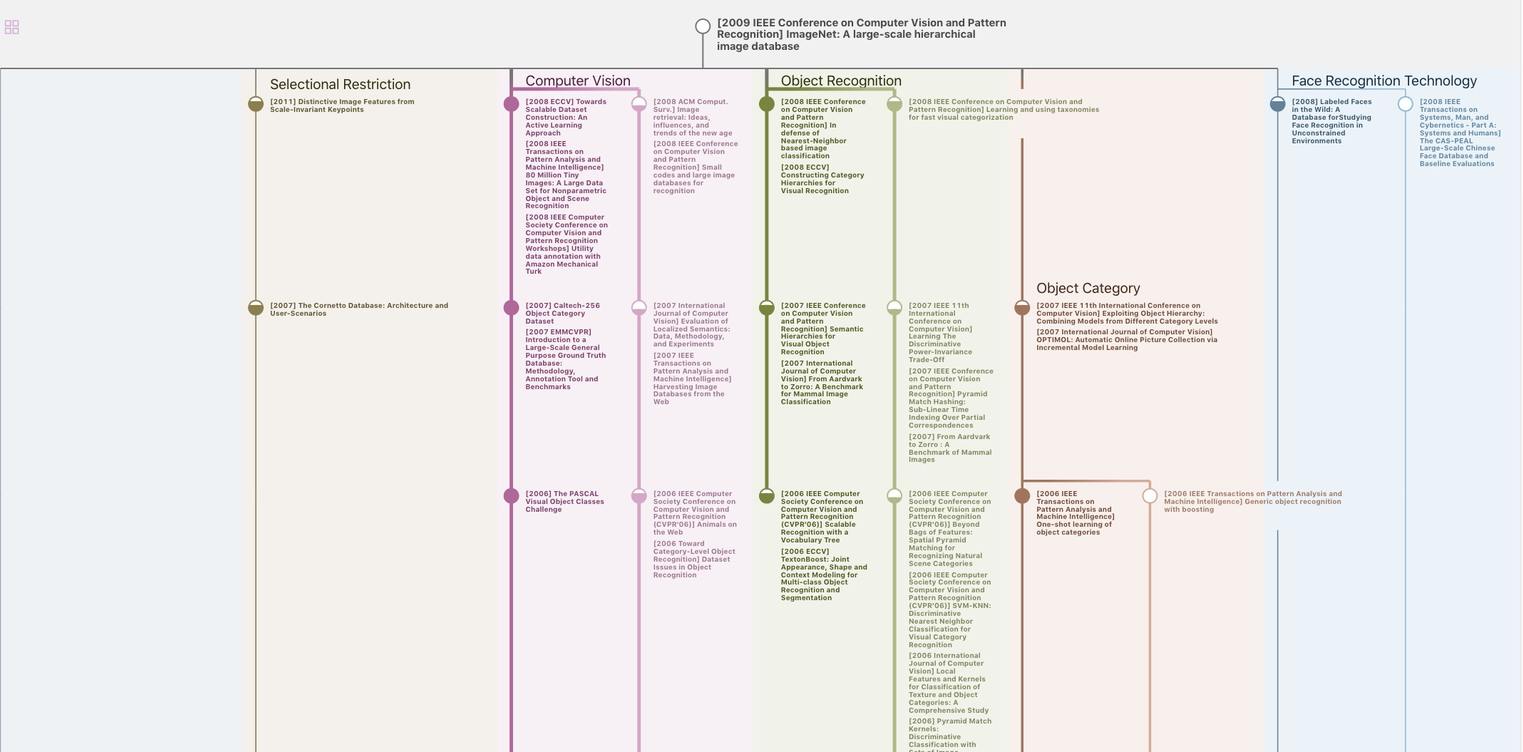
生成溯源树,研究论文发展脉络
Chat Paper
正在生成论文摘要