Improved Speech Emotion Recognition Using Channel-wise Global Head Pooling (CwGHP)
CIRCUITS SYSTEMS AND SIGNAL PROCESSING(2023)
摘要
A multihead attention-based convolutional neural network (CNN) architecture known as channel-wise global head pooling is proposed to improve the classification accuracy of speech emotion recognition. A time-frequency kernel is used in two-dimensional convolution to emphasize both the scales in mel-frequency-cepstral-coefficients. Following the CNN encoder, a multihead attention network is optimized to learn salient discriminating characteristics of audio samples on the three emotional speech datasets, including the interactive emotional dyadic motion capture in English, the Berlin emotional speech dataset in the German language, and Ryerson audio-visual database of emotional speech and song in North American English. The proposed model’s robustness is demonstrated in these diverse language datasets. A chunk-level classification approach is utilized for model training with source labels for each segment. While performing the model evaluation, an aggregation of emotions is applied to achieve the emotional sample classification. The classification accuracy is improved to 84.89% and 82.87% unweighted accuracy (UA) and weighted accuracy (WA) on the IEMOCAP dataset. It is the state-of-the-art performance on this speech corpus compared to (79.34% of WA and 77.54% of UA) using only audio modality; the proposed method achieved a UA improvement of more than 7%. Furthermore, it validated the model on two other datasets via a series of experiments that yielded acceptable results. The model is investigated using WA and UA. Additionally, statistical parameters, including precision, recall and F 1-score, are also used to estimate the effectiveness of each emotion class.
更多查看译文
关键词
Speech emotion recognition,Multihead attention,Convolutional neural network,MFCC,Adaptive pooling
AI 理解论文
溯源树
样例
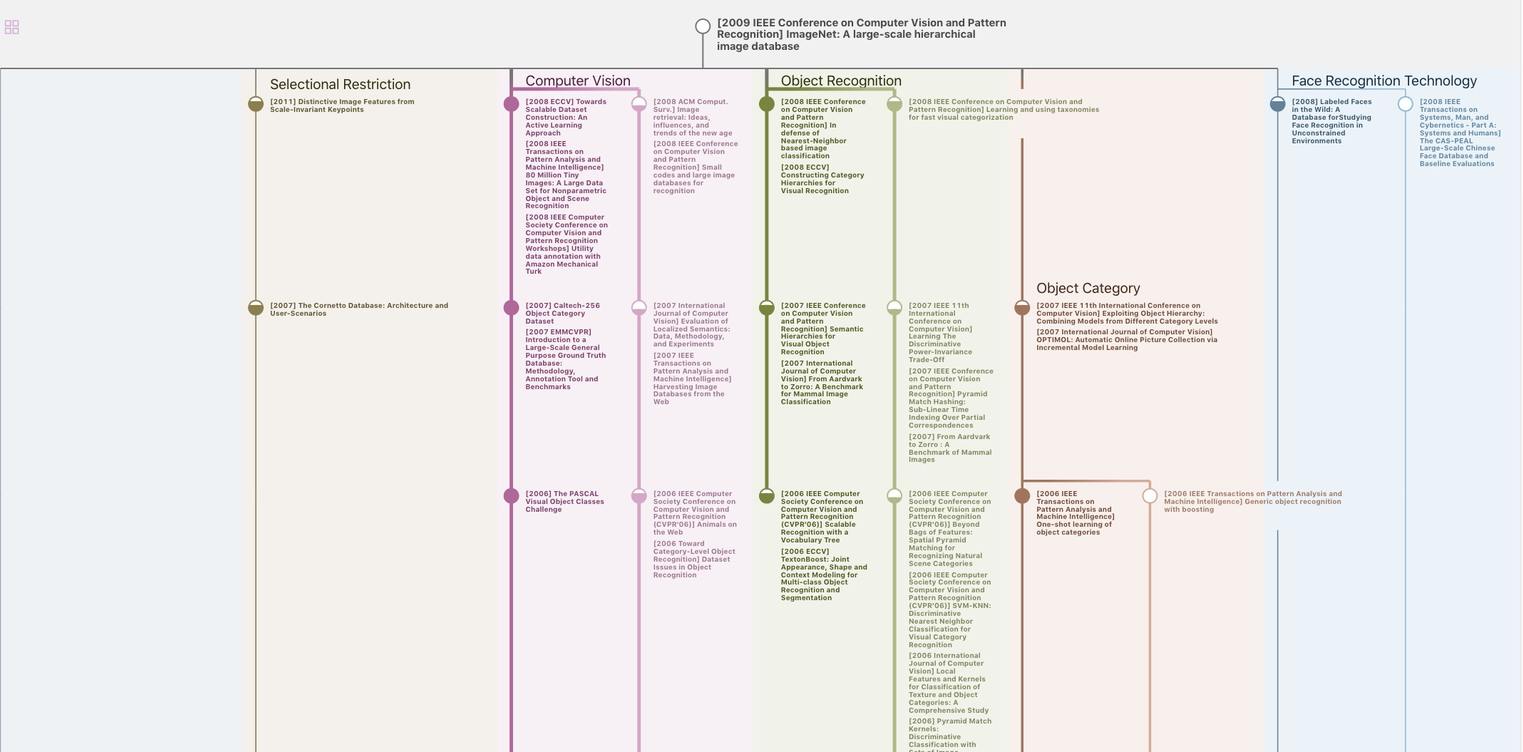
生成溯源树,研究论文发展脉络
Chat Paper
正在生成论文摘要