A multi-stage model for bird's eye view prediction based on stereo-matching model and RGB-D semantic segmentation
IET INTELLIGENT TRANSPORT SYSTEMS(2023)
摘要
Bird's-Eye-View (BEV) map is a powerful and detailed scene representation for intelligent vehicles that provides both the location and semantic information about nearby objects from a top-down perspective. BEV map generation is a complex multi-stage task, and the existing methods typically perform poorly for distant scenes. Thus, the authors introduce a novel multi-stage model that infers to obtain more accurate BEV map. First, the authors propose the Adaptive Aggregation with Stereo Mixture Density (AA-SMD) model, which is an improved stereo matching model that eliminates bleeding artefacts and provides more accurate depth estimation. Next, the authors employ the RGB-Depth (RGB-D) semantic segmentation model to improve the semantic segmentation performance and connectivity of their model. The depth map and semantic segmentation maps are then combined to create an incomplete BEV map. Finally, the authors propose a Multi Strip Pooling Unet (MSP-Unet) model with a hierarchical multi-scale (HMS) attention and strip pooling (SP) module to improve prediction with BEV generation. The authors evaluate their model with a Car Learn to Act (CARLA)-generated synthetic dataset. The experiment results demonstrate that the authors' model generates a highly accurate representation of the surrounding environment achieving a state-of-the-art result of 61.50% Mean Intersection-over-Union (MIoU) across eight classes.
更多查看译文
关键词
autonomous driving,image recognition,learning (artificial intelligence)
AI 理解论文
溯源树
样例
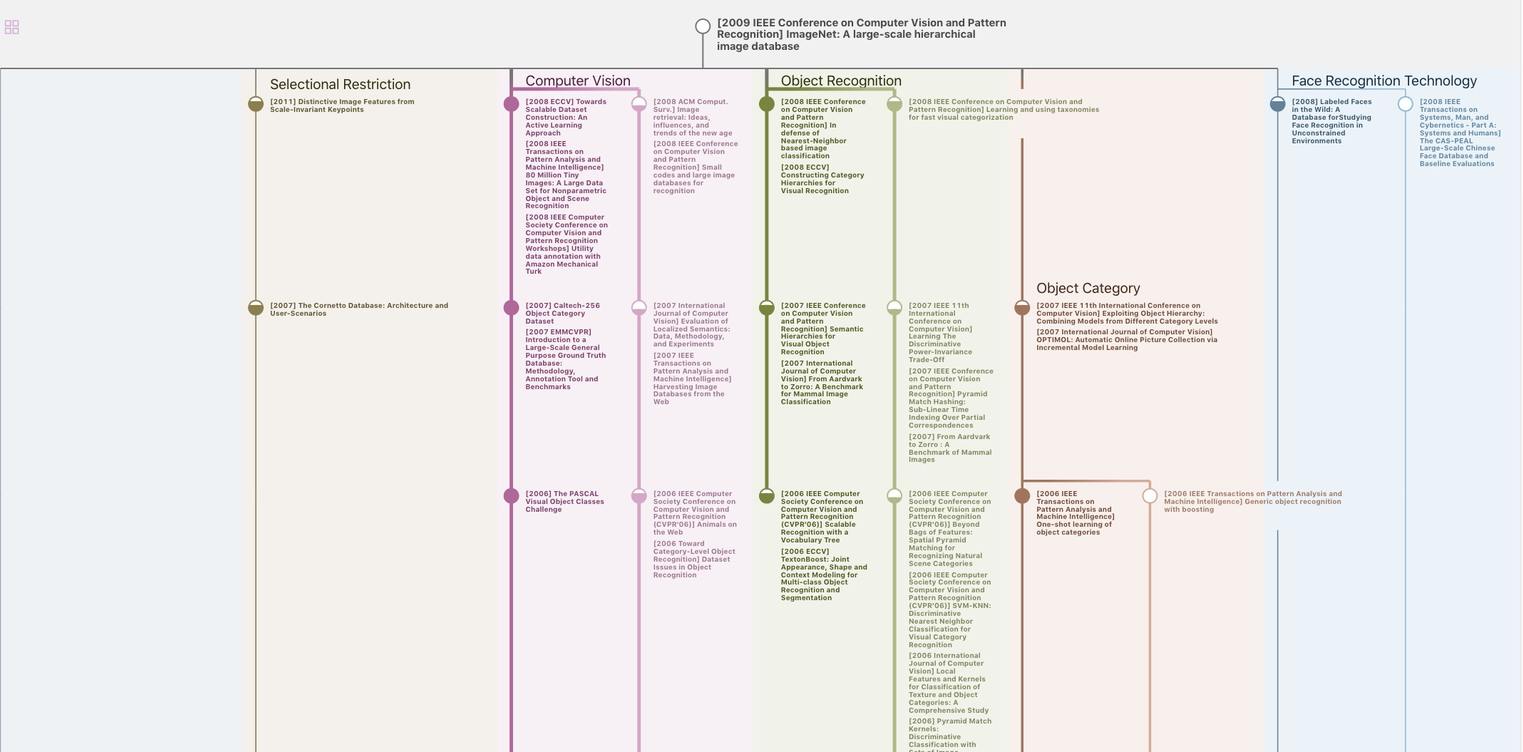
生成溯源树,研究论文发展脉络
Chat Paper
正在生成论文摘要