Time series KSTAR PF superconducting coil temperature forecasting using recurrent transformer model
FUSION ENGINEERING AND DESIGN(2023)
摘要
In this paper, we propose a KSTAR PF superconducting coil temperature forecasting model using a recurrent transformer model to protect the PF coil during operation. We developed a Recurrent Transformer model (RTransformer) that recurrently forecasts future time points using current time input data and latent variables from previous time steps based on the perceiver autoregressive model. The RTransformer model is compu-tationally and memory efficient, requiring lower overhead than the Transformer model as it only computes forecasting points using a subset of input sequence data instead of the entire sequence of time windows. The RTransformer model was trained using the KSTAR PF coil temperature dataset obtained from the PF coil monitoring system. We compared the performance of the proposed model with Long-Short-Term memory (LSTM), Transformer model, and TransformerXL, based on R2 score, Mean Absolute Error (MAE), Root Mean Squared Error (RMSE), and Mean Absolute Percentage Error (MAPE). We also compared the inference time for each model. The experimental results showed that the proposed model outperformed other deep learning models, with lower error rates and similar inference times to the Transformer model. For most performance metrics, our proposed model scored among the top 1 and top 2 scoring models.
更多查看译文
关键词
coil temperature forecasting,time series kstar pf,transformer
AI 理解论文
溯源树
样例
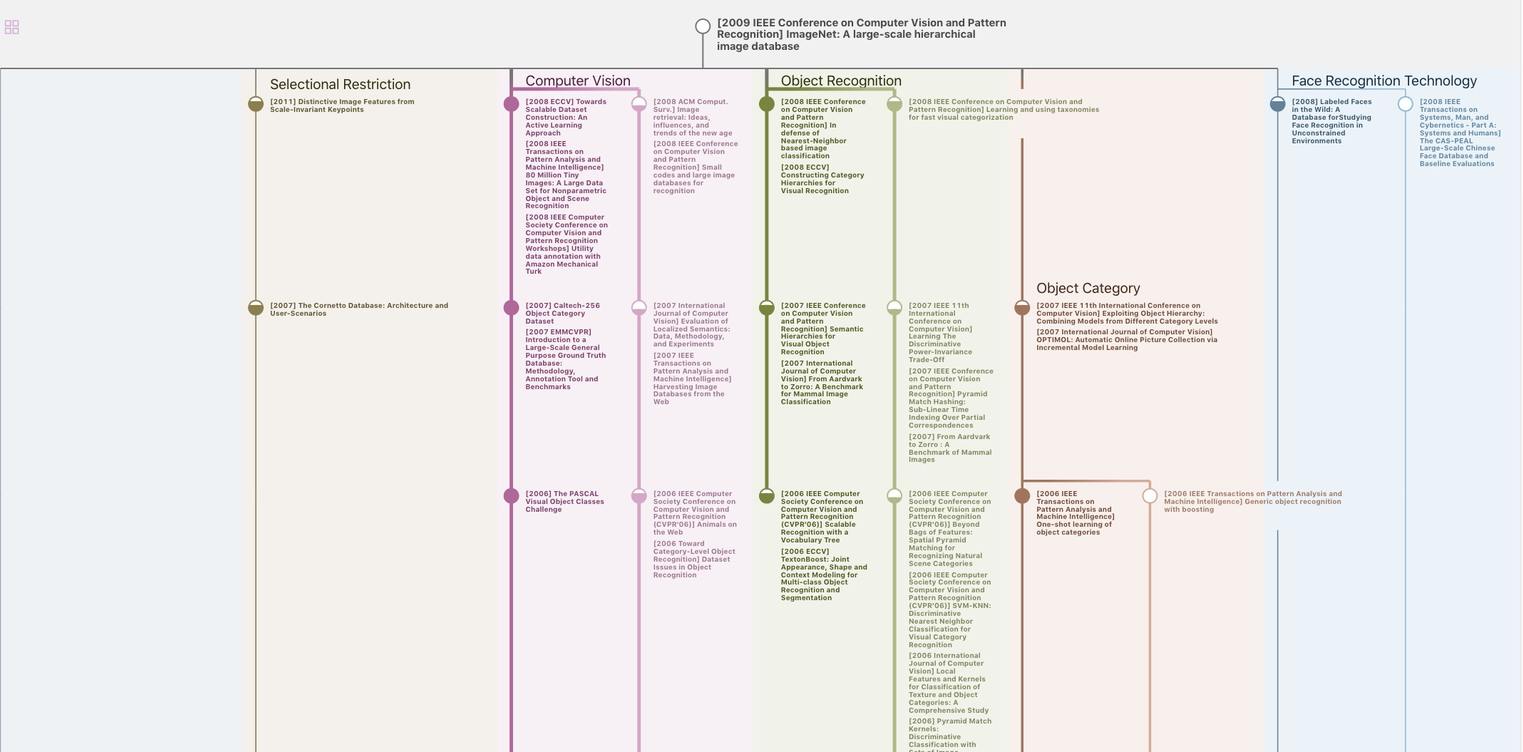
生成溯源树,研究论文发展脉络
Chat Paper
正在生成论文摘要