Multiview Feature Selection With Information Complementarity and Consensus for Fault Diagnosis
IEEE TRANSACTIONS ON SYSTEMS MAN CYBERNETICS-SYSTEMS(2023)
摘要
Feature selection plays a critical role in data-driven fault diagnosis. Existing works often perform feature selection on a single overcomplete feature pool that is collected from various feature extraction approaches, such as signal processing and deep learning. However, simple concatenation of these "multiview" features may neglect the inherent properties and cross-feature correlations, often leading to ineffectiveness in feature selection for fault diagnosis. Therefore, a multiview feature selection with information complementarity and consensus (MFSICC) is proposed in this article to efficiently select relevant features for fault diagnosis. In particular, both complementary and consensus properties that are shared among the multiview features are incorporated based on a structured sparsity learning model. Besides, an iterative algorithm is proposed to solve the MFSICC problem with theoretically approved convergence. Experiments on bearing and gearbox fault diagnosis with real-world datasets validate the effectiveness of MFSICC. Important model parameters and empirical convergence are also discussed to facilitate practical use.
更多查看译文
关键词
fault diagnosis,selection,information complementarity
AI 理解论文
溯源树
样例
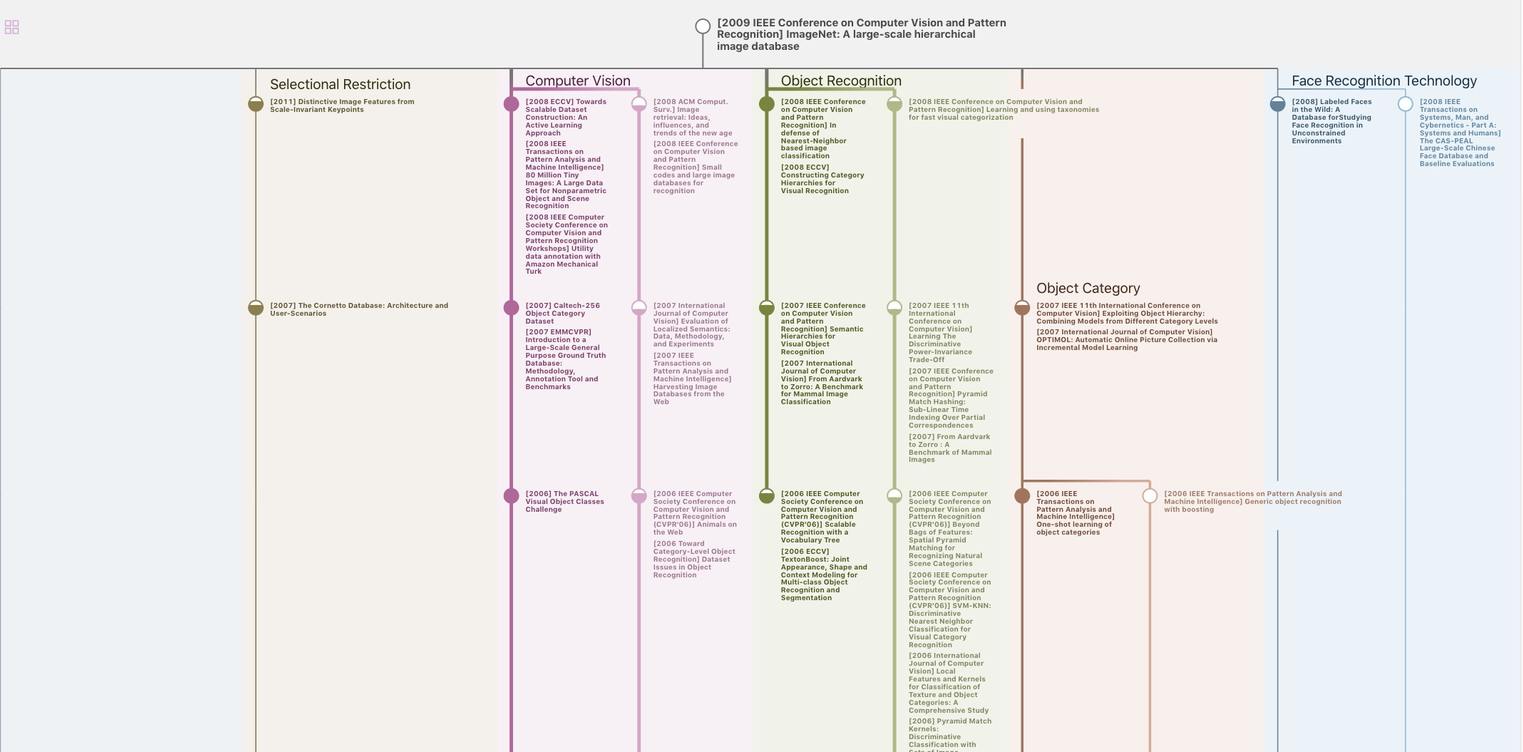
生成溯源树,研究论文发展脉络
Chat Paper
正在生成论文摘要