Three-dimensional seismic data reconstruction via partial sum of tensor nuclear norm minimization
JOURNAL OF GEOPHYSICS AND ENGINEERING(2023)
摘要
Rank-reduction has become a hotspot in seismic data reconstruction research in recent years. Traditional rank-reduction methods generally use the nuclear norm as a convex proxy for rank, but these methods overly penalize large singular values, leading to reconstruction results that deviate from the optimal solution. In this paper, we propose a tensor robust principal component analysis model with minimization of the partial sum of tensor nuclear norm for three-dimensional (3D) reconstruction of seismic data. The partial sum of tensor nuclear norm minimizes only the partial singular values and can approximate the rank function more accurately. TRPCA can accurately recover the 3D tensor corrupted by sparse errors, improving the accuracy of seismic data reconstruction. The experimental results of the simulated data and real data show that the reconstruction effect of the proposed method on the 3D seismic data is better than the compared methods.
更多查看译文
关键词
rank reduction, partial sum of tensor nuclear norm, tensor robust principal component analysis, 3D seismic data reconstruction
AI 理解论文
溯源树
样例
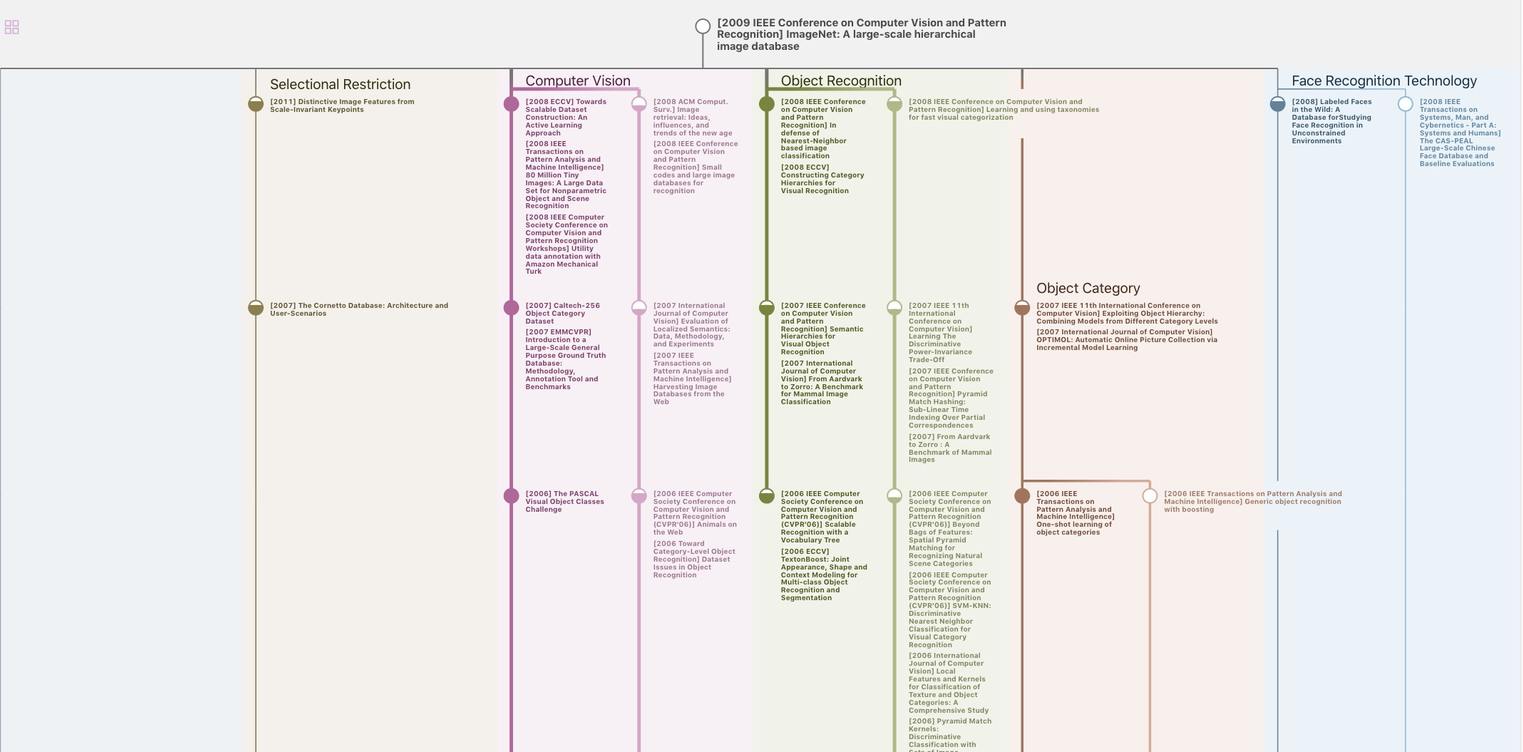
生成溯源树,研究论文发展脉络
Chat Paper
正在生成论文摘要