Graph Convolutional Neural Network Based Malware Detection in IoT-Cloud Environment
INTELLIGENT AUTOMATION AND SOFT COMPUTING(2023)
摘要
Cybersecurity has become the most significant research area in the domain of the Internet of Things (IoT) owing to the ever-increasing number of cyberattacks. The rapid penetration of Android platforms in mobile devices has made the detection of malware attacks a challenging process. Furthermore, Android malware is increasing on a daily basis. So, precise malware detection analytical techniques need a large number of hardware resources that are signifi- cantly resource-limited for mobile devices. In this research article, an optimal Graph Convolutional Neural Network-based Malware Detection and classification (OGCNN-MDC) model is introduced for an IoT-cloud environment. The pro-posed OGCNN-MDC model aims to recognize and categorize malware occur-rences in IoT-enab led cloud platforms. The presented OGCNN-MDC model has three stages in total, such as data pre-processing, malware detection and para-meter tuning. To detect and classify the malware, the GCNN model is exploited in this work. In order to enhance the overall efficiency of the GCNN model, the Group Mean-based Optimizer (GMBO) algorithm is utilized to appropriately adjust the GCNN parameters, and this phenomenon shows the novelty of the cur-rent study. A widespread experimental analysis was conducted to establish the superiority of the proposed OGCNN-MDC model. A comprehensive comparison study was conducted, and the outcomes highlighted the supreme performance of the proposed OGCNN-MDC model over other recent approaches.
更多查看译文
关键词
Cybersecurity,IoT,cloud,malware detection,graph convolution network
AI 理解论文
溯源树
样例
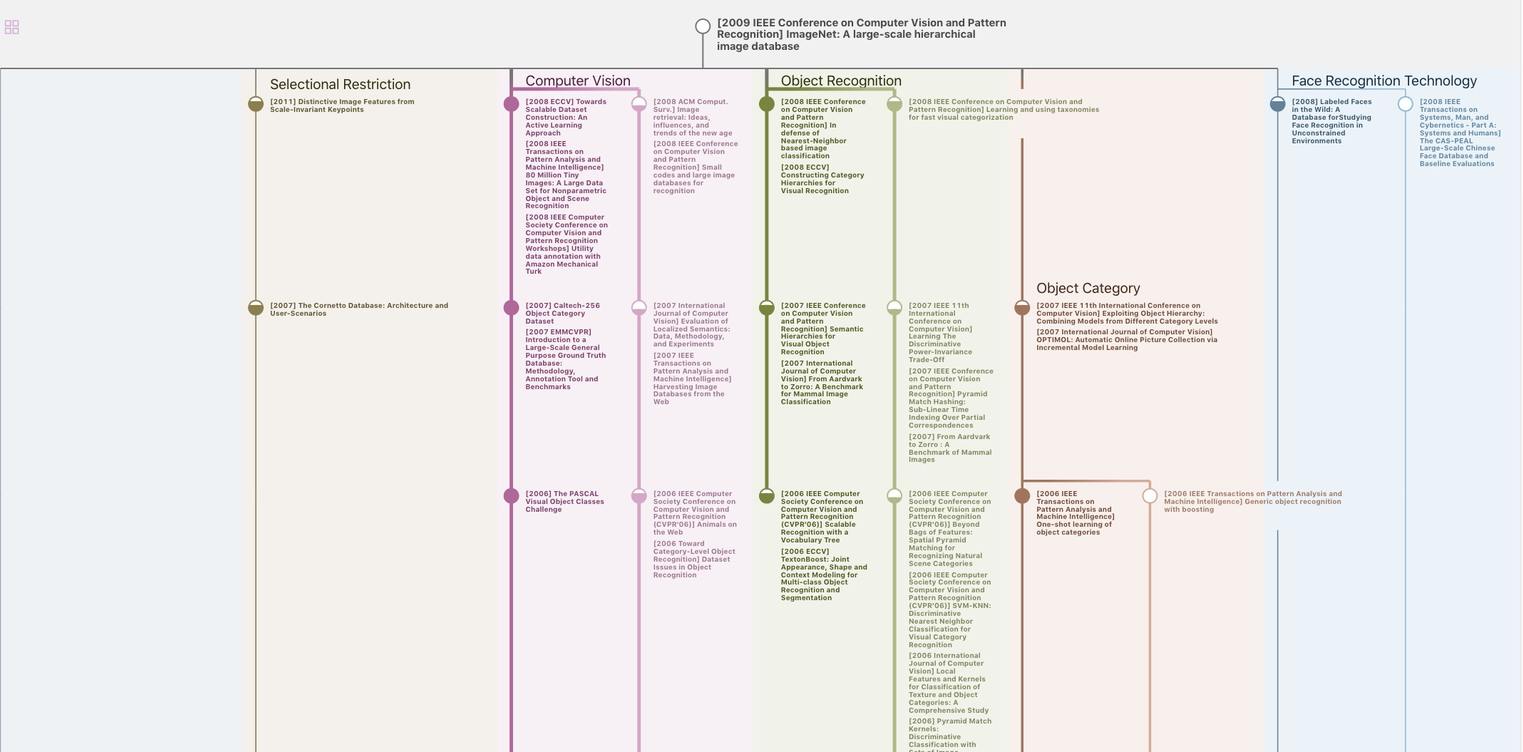
生成溯源树,研究论文发展脉络
Chat Paper
正在生成论文摘要