Gray Level Co-Occurrence Matrix, Fractal and Wavelet Analyses of Discrete Changes in Cell Nuclear Structure following Osmotic Stress: Focus on Machine Learning Methods
FRACTAL AND FRACTIONAL(2023)
摘要
In this work, we demonstrate that it is possible to create supervised machine-learning models using a support vector machine and random forest algorithms to separate yeast cells exposed to hyperosmotic stress from intact cells. We performed fractal, gray level co-occurrence matrix (GLCM), and discrete wavelet transform analyses on digital micrographs of nuclear regions of interest of a total of 2000 Saccharomyces cerevisiae cells: 1000 exposed to hyperosmotic environments and 1000 control cells. For each nucleus, we calculated values for fractal dimension, angular second moment, inverse difference moment, textural contrast, correlation feature, textural variance, and discrete wavelet coefficient energy. The support vector machine achieved an acceptable classification accuracy of 71.7% in predicting whether the cell belonged to the experimental or control group. The random forest model performed better than the support vector machine, with a classification accuracy of 79.8%. These findings can serve as a starting point for developing AI-based methods that use GLCM, fractal, and wavelet data to classify damaged and healthy cells and make predictions about various physiological and pathological phenomena associated with osmotic stress.
更多查看译文
关键词
cell,signal analysis,nucleus,stress,supervised learning
AI 理解论文
溯源树
样例
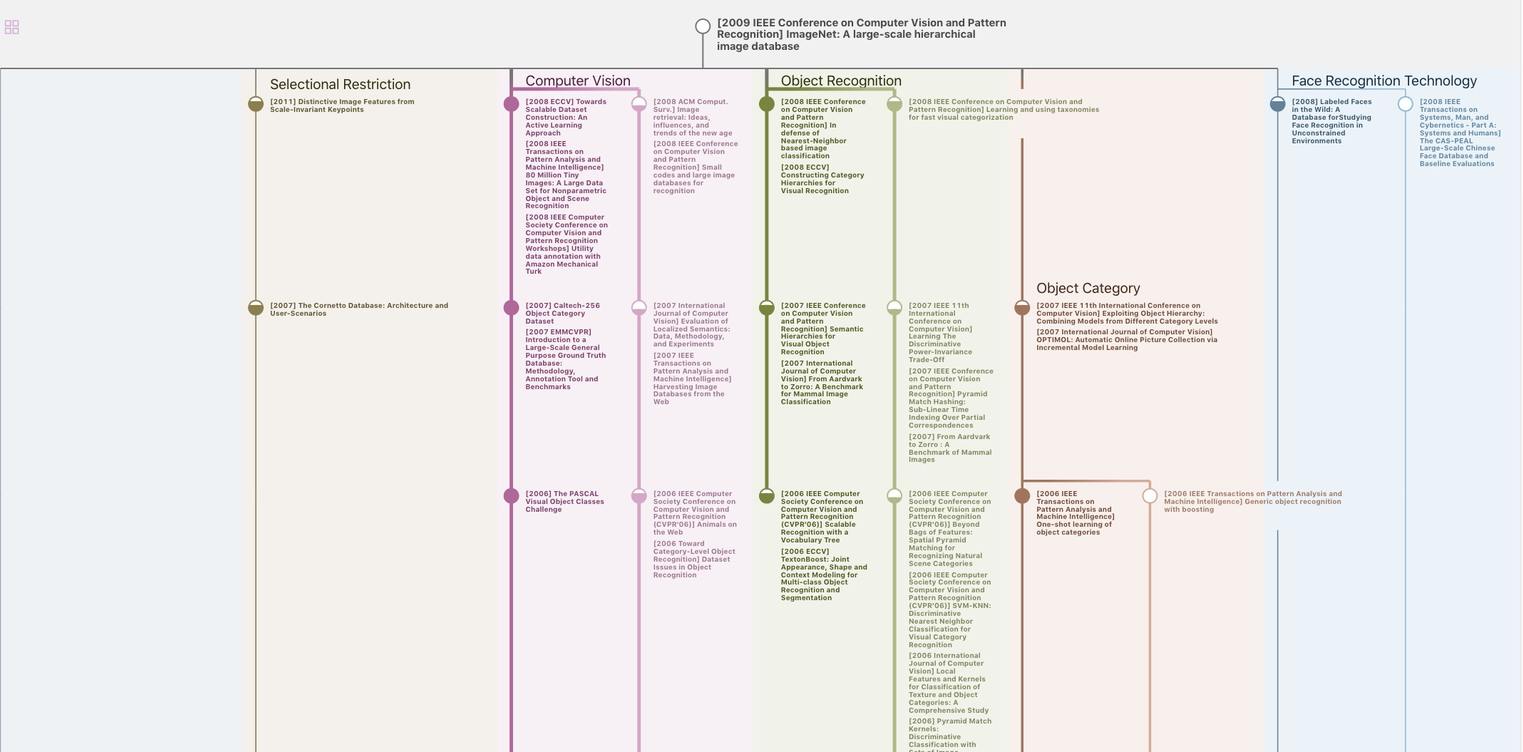
生成溯源树,研究论文发展脉络
Chat Paper
正在生成论文摘要