Extraction of Interconnect Parasitic Capacitance Matrix Based on Deep Neural Network
ELECTRONICS(2023)
摘要
Interconnect parasitic capacitance extraction is crucial in analyzing VLSI circuits' delay and crosstalk. This paper uses the deep neural network (DNN) to predict the parasitic capacitance matrix of a two-dimensional pattern. To save the DNN training time, the neural network's output includes only coupling capacitances in the matrix, and total capacitances are obtained by summing corresponding predicted coupling capacitances. In this way, we can obtain coupling and total capacitances simultaneously using a single neural network. Moreover, we introduce a mirror flip method to augment the datasets computed by the finite element method (FEM), which doubles the dataset size and reduces data preparation efforts. Then, we compare the prediction accuracy of DNN with another neural network ResNet. The result shows that DNN performs better in this case. Moreover, to verify our method's efficiency, the total capacitances calculated from the trained DNN are compared with the network (named DNN-2) that takes the total capacitance as an extra output. The results show that the prediction accuracy of the two methods is very close, indicating that our method is reliable and can save the training workload for the total capacitance. Finally, a solving efficiency comparison shows that the average computation time of the trained DNN for one case is not more than 2% of that of FEM.
更多查看译文
关键词
interconnect wire,parasitic capacitance matrix,data augmentation,DNN,ResNet
AI 理解论文
溯源树
样例
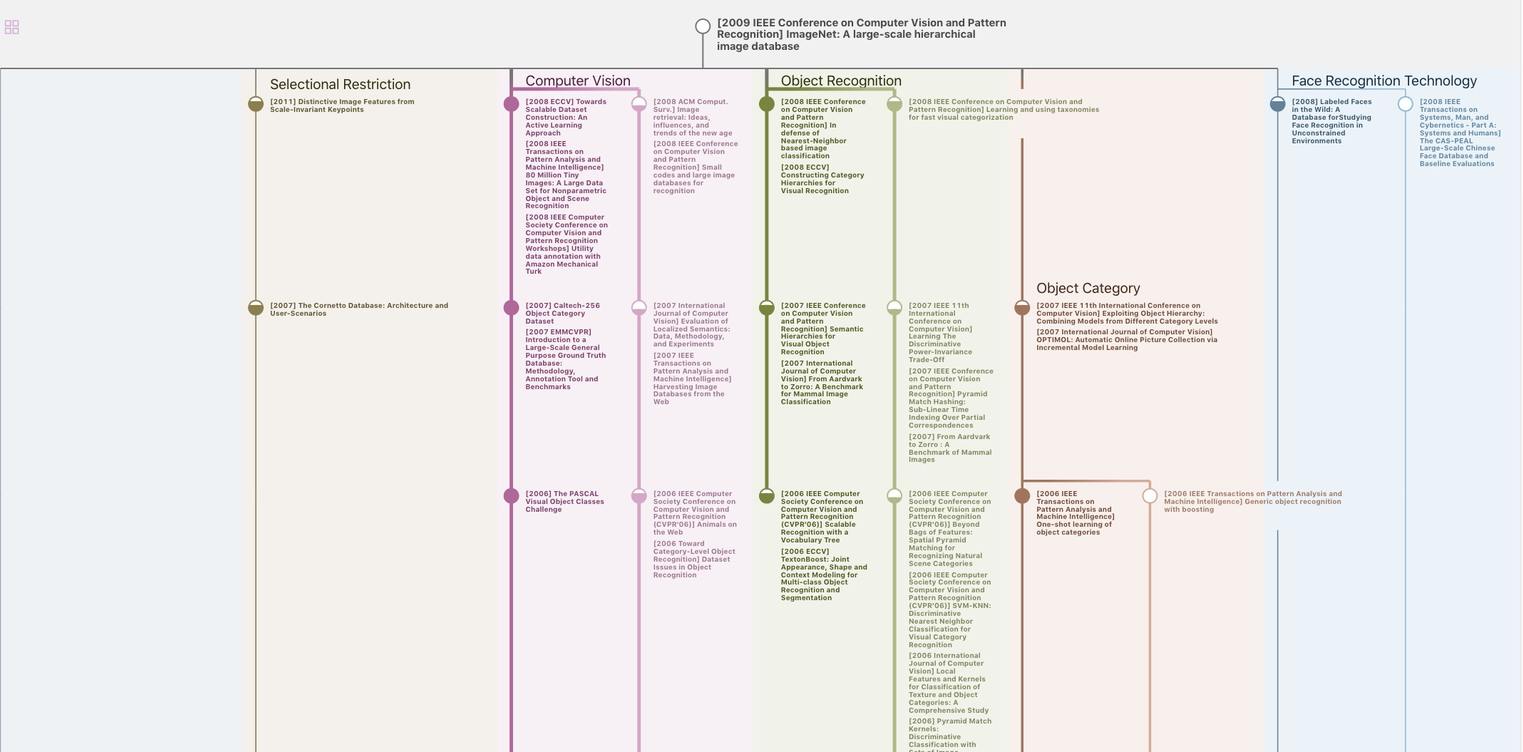
生成溯源树,研究论文发展脉络
Chat Paper
正在生成论文摘要