A surrogate-assisted evolutionary algorithm based on multi-population clustering and prediction for solving computationally expensive dynamic optimization problems
EXPERT SYSTEMS WITH APPLICATIONS(2023)
摘要
The computationally expensive dynamic optimization problems (CEDOPs) arise when the objective function of a dynamic optimization problem is prohibitively expensive, or even almost impossible, to obtain. Such problems are extremely challenging from a computational perspective, due to the combination of dynamic environments and computationally expensive objective functions. In this paper, we present a surrogate-assisted evolutionary algorithm based on multi-population clustering and prediction (SAEA/MPCP) to tackle CEDOPs. In the solution process, SAEA/MPCP employs three basic strategies. First, to improve the solution speed and the ability to interact with the environment of the algorithm, the initial population were selected using the strategy of clustering and differential prediction. Second, in order to increase the distribution and diversity of individuals and improve the solution accuracy of the algorithm, the generation of sample points for surrogate model was performed using integrated multi-population strategies. Third, we replace the two kinds of high and low confidence samples in the Co-kriging model with the generated samples from the previous strategy, which further improves the construction and optimization efficiency of the surrogate model. Finally, we set up two types of CEDOPs with different degrees of computational cost and experimentally compare them with a variety of state-of-the-art dynamic optimization algorithms (DOAs), respectively. The experiments fully demonstrate the effectiveness of SAEA/MPCP algorithm to some of the best known DOAs.
更多查看译文
关键词
Dynamic optimization problems,Evolutionary computation,Multi-population clustering and prediction,Co-kriging model
AI 理解论文
溯源树
样例
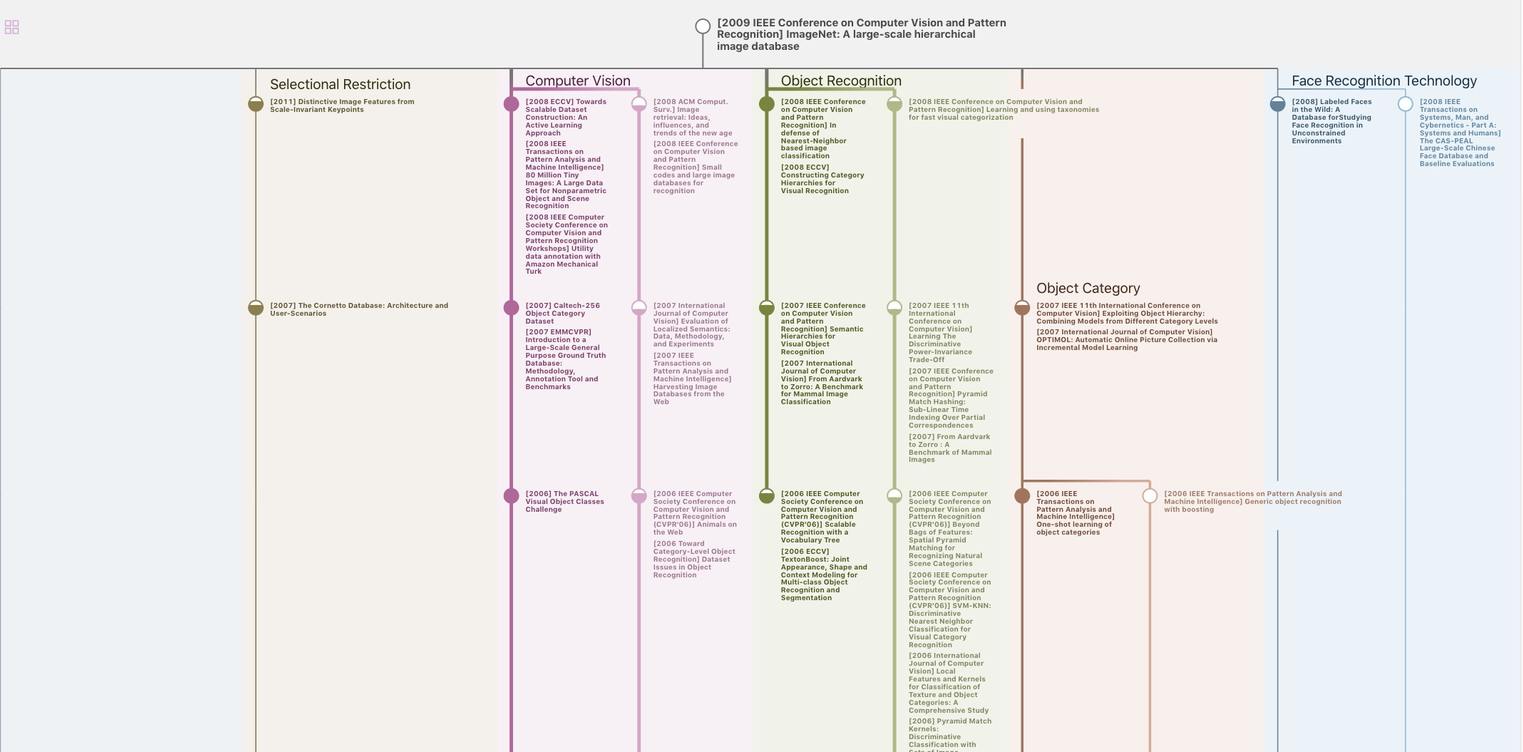
生成溯源树,研究论文发展脉络
Chat Paper
正在生成论文摘要