Lambing event detection using deep learning from accelerometer data
COMPUTERS AND ELECTRONICS IN AGRICULTURE(2023)
摘要
Newborn lamb mortality is a major economic and welfare concern for the sheep industry globally, which can potentially be reduced through automated monitoring of lambing difficulties and quantifying time of birth. This study investigated the identification of lambing ewes' behaviours during labour and post-partum licking, by applying deep learning algorithms to halter-mounted accelerometer sensor data. 101 lambing ewes from two experiments and 29 non-pregnant ewes from three other experiments, were fitted with the sensors. Ground truth behaviour labels, 5 s long, were obtained based on video recordings of the study sheep. Classification using a Long Short-Term Memory (LSTM) model, was performed for different ethograms: labour behaviours, phases of labour, licking only, and labour and licking in the context of broader grazing behaviours. The model was fine-tuned with data of six sheep where the first birth fell within the observation period. A combined grazing and lambing behaviour ethogram achieved the best performance (accuracy: 81%) with recall of 0.85 for the licking behaviour. Fine-tuning increased performance further (average accuracy: 86.3%), with a best case recall of 0.88 for labour and 0.94 for licking. The licking only ethogram demonstrated strong recall for licking (0.9) when the epoch length was increased to 60 s. Isolation of the labour and licking behaviours achieved 84.8% accuracy, with a weighted F1-score of 0.85, demonstrating the ability to separate the labour phases. The study presents a strong foundation for the development of systems to detect lambing events and prolonged labour.
更多查看译文
关键词
On-animal sensors,Parturition,Machine learning,Sheep,Behaviour classification
AI 理解论文
溯源树
样例
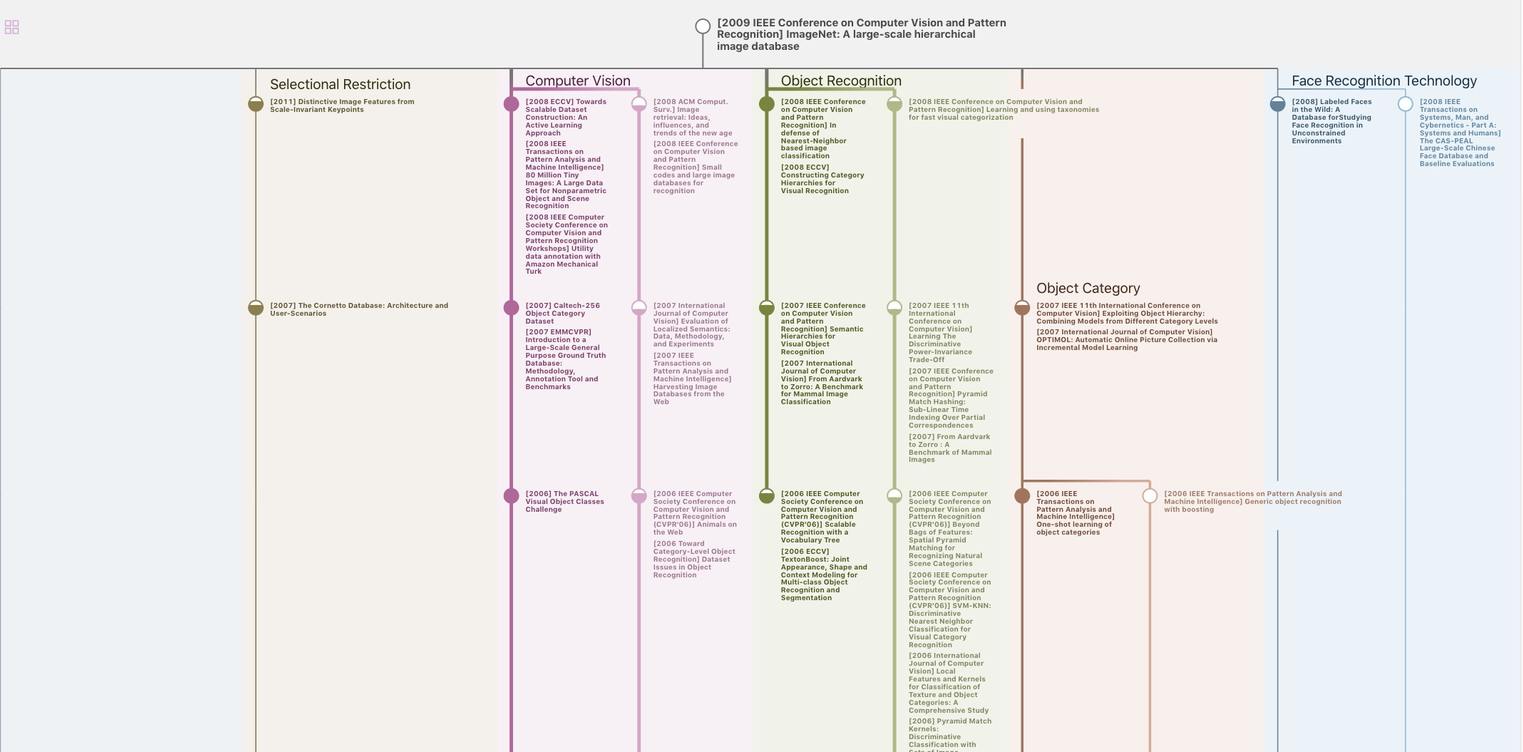
生成溯源树,研究论文发展脉络
Chat Paper
正在生成论文摘要