Predicting the NOx emissions of low heat value gas rich-quench-lean combustor via three integrated learning algorithms with Bayesian optimization
ENERGY(2023)
摘要
With the increased attention to low heat value gas fuels in recent years, research on NOx emissions from the combustors of low heat value gas fuels is necessary. This study introduces integrated learning algorithms to the NOx pollution emission prediction from gas turbine combustors. The combustor is a self-designed low heat value gas rich-quench-lean combustor and 92 sets of emission data have been obtained using simulation methods. The simulation data has been experimentally verified. The effect of four control parameters on NOx emissions has been investigated: inlet air temperature, inlet air mass flow rate, swirler installation angle, and combustor lean burn zone length. Pearson correlation coefficients show that four control parameters have very low correlations. The three integrated learning algorithms used in this study are the random forest regression algorithm, the extreme gradient boosting algorithm, and the natural gradient boosting algorithm. The hyperparameters of the three integrated learning algorithms are optimised using the Bayesian optimization algorithm. Three integrated learning algorithms are utilized to predict pollutant emission characteristics and the results show that NGboost provides the best predictions and Random Forest the worst, with NGboost suffering from overfitting problems. The results of the algorithm predictions were analyzed to understand the impact of different control parameters on the NOx emissions. A feature importance evaluation shows that the influence of inlet air temperature on NOx emissions far outweighs the other three parameters.
更多查看译文
关键词
Low heat value gas,RQL combustor,Integrated learning algorithm,NOx emission,Prediction
AI 理解论文
溯源树
样例
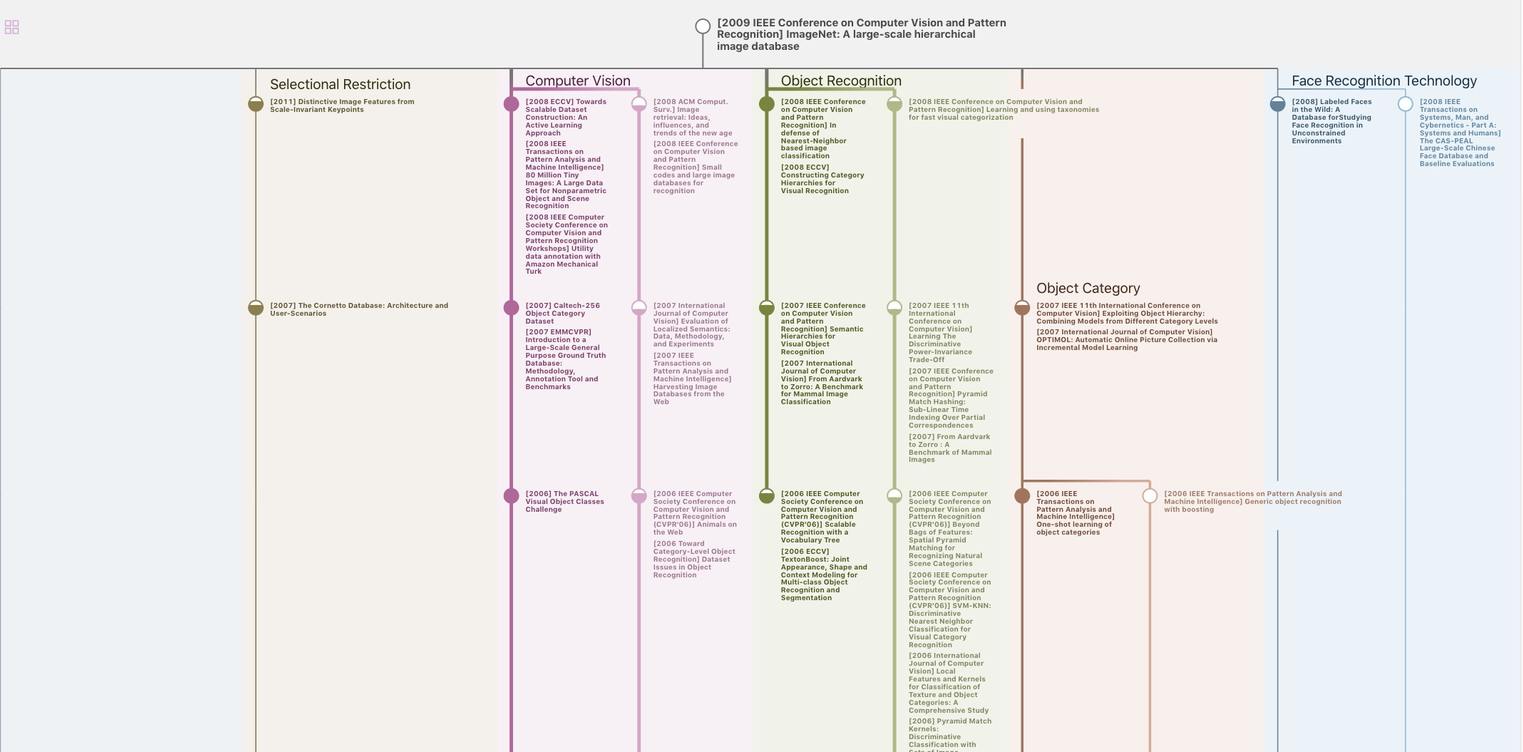
生成溯源树,研究论文发展脉络
Chat Paper
正在生成论文摘要