Terrain Classification of Hyperspectral Remote Sensing Images Based on SC-KSDA
ADVANCES IN NATURAL COMPUTATION, FUZZY SYSTEMS AND KNOWLEDGE DISCOVERY, ICNC-FSKD 2022(2023)
摘要
To solve the problem of "same object but different spectrum" of hyperspectral remote sensing images and further extract more effective nonlinear separability features, in this paper, spectral clustering and subclass discriminant analysis (SC-SDA) is generalized to the kernel space, spectral clustering and kernel subclass discriminant analysis (SC-KSDA) is proposed. The SC-SDA method can automatically distinguish the number of clusters for the same class of ground objects that can be divided, and divide them into subclasses, and calculate the final feature subspace by subclass discriminant analysis (SDA). In this paper, the results of subclass division of various classes are obtained based on the SC-SDA method, and then the criterion function on data separability and kernel parameters is designed to map the data divided by subclasses into high-dimensional kernel space. Finally, each subclass is treated as a separate class, and linear discriminant analysis (LDA) method is used to extract nonlinear separable features in kernel space. The experimental results of the three measured hyperspectral remote sensing images using the minimum distance classifier show that proposed SC-KSDA can effectively improve accuracy of feature classification.
更多查看译文
关键词
Hyperspectral remote sensing images,Feature extraction,Kernel subclass discriminant analysis
AI 理解论文
溯源树
样例
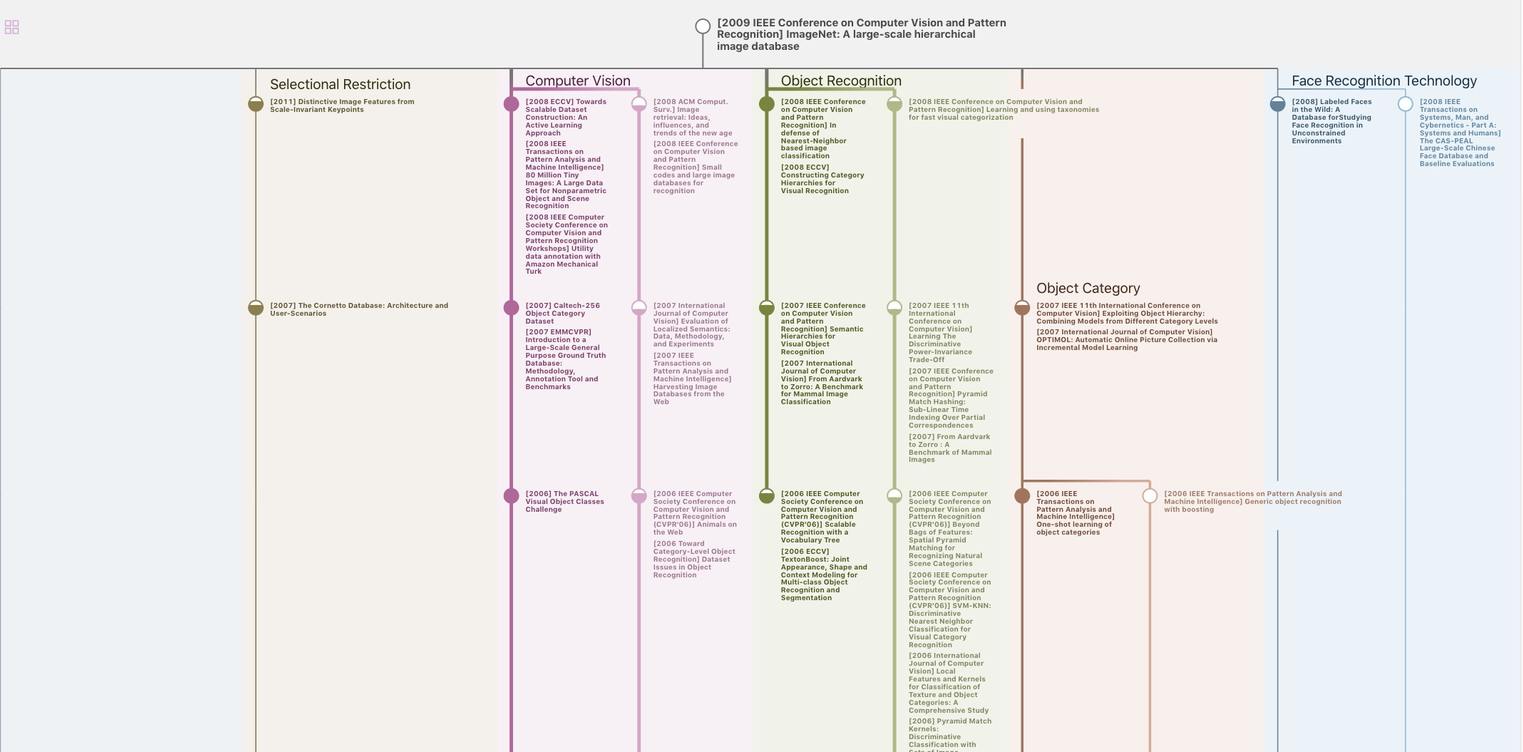
生成溯源树,研究论文发展脉络
Chat Paper
正在生成论文摘要