The Value of Randomized Strategies in Distributionally Robust Risk-Averse Network Interdiction Problems
INFORMS JOURNAL ON COMPUTING(2023)
摘要
Conditional value at risk (CVaR) is widely used to account for the preferences of a risk-averse agent in extreme loss scenarios. To study the effectiveness of randomization in interdiction problems with an interdictor that is both risk- and ambiguity-averse, we introduce a distributionally robust maximum flow network interdiction problem in which the interdictor randomizes over the feasible interdiction plans in order to minimize the worst case CVaR of the maximum flow with respect to both the unknown distribution of the capacity of the arcs and the interdictor's own randomized strategy. Using the size of the uncertainty set, we control the degree of conservatism in the model and reformulate the interdictor's distributionally robust optimization problem as a bilinear optimization problem. For solving this problem to any given optimality level, we devise a spatial branch-and-bound algorithm that uses the McCormick inequalities and reduced reformulation linearization technique to obtain a convex relaxation of the problem. We also develop a column-generation algorithm to identify the optimal support of the convex relaxation, which is then used in the coordinate descent algorithm to determine the upper bounds. The efficiency and convergence of the spatial branch-and-bound algorithm is established in numerical experiments. Further, our numerical experiments show that randomized strategies can have significantly better performance than optimal deterministic ones.
更多查看译文
关键词
network interdiction,conditional value at risk,distributionally robust optimization,spatial branch and bound,column generation
AI 理解论文
溯源树
样例
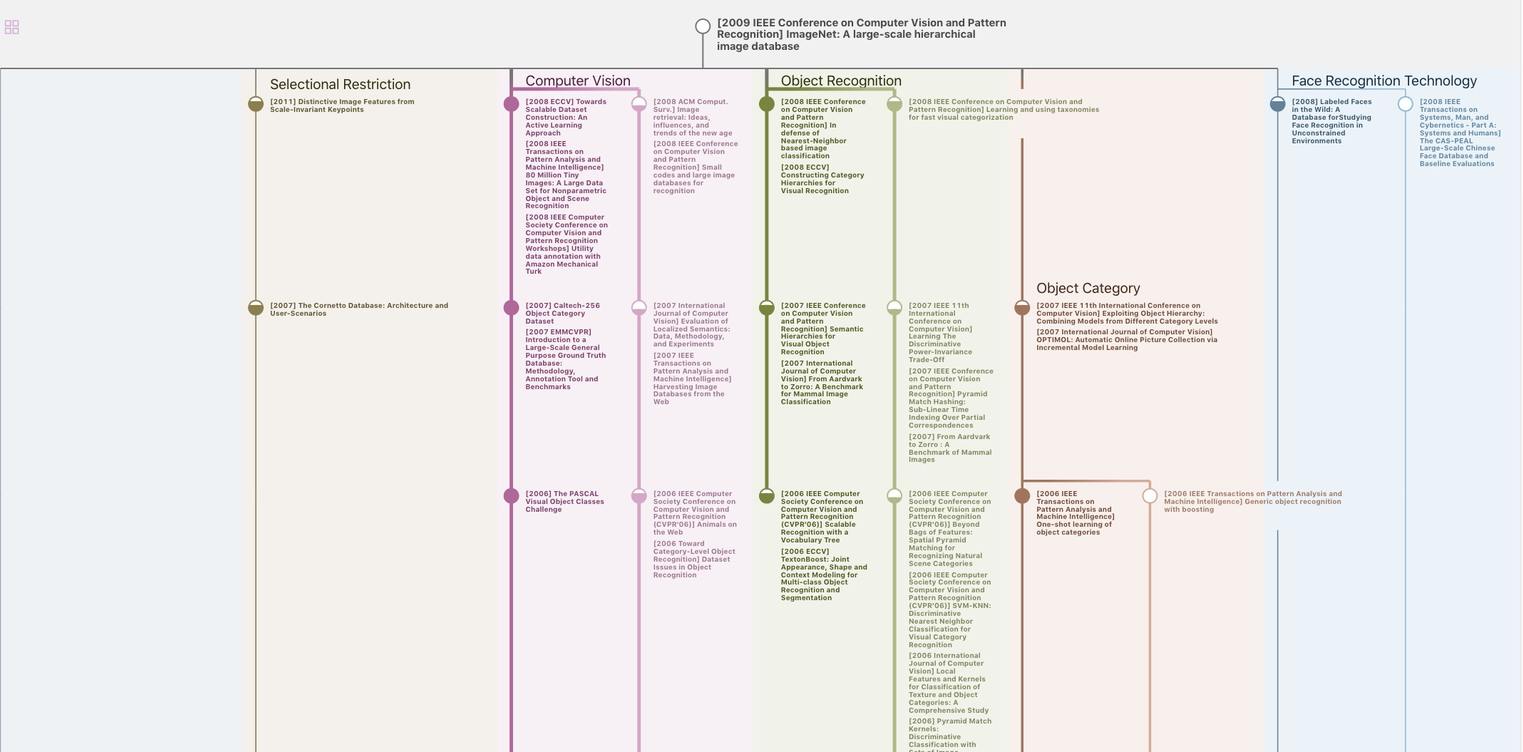
生成溯源树,研究论文发展脉络
Chat Paper
正在生成论文摘要