Optimizing Cascaded Control of Mechatronic Systems through Constrained Residual Reinforcement Learning
MACHINES(2023)
摘要
Cascaded control structures are prevalent in industrial systems with many disturbances to obtain stable control but are cumbersome and challenging to tune. In this work, we propose cascaded constrained residual reinforcement learning (RL), an intuitive method that allows to improve the performance of a cascaded control structure while maintaining safe operation at all times. We draw inspiration from the constrained residual RL framework, in which a constrained reinforcement learning agent learns corrective adaptations to a base controller's output to increase optimality. We first revisit the interplay between the residual agent and the baseline controller and subsequently extend this to the cascaded case. We analyze the differences and challenges this structure brings and derive some principle insights from this into the stability and operation of the cascaded residual architecture. Next, we propose a novel actor structure to enable efficient learning under the cascaded setting. We show that the standard algorithm is suboptimal for application to cascaded control structures and validate our method on a high-fidelity simulator of a dual motor drivetrain, resulting in a performance improvement of 14.7% on average, with only a minor decrease in performance occurring during the training phase. We study the different principles constituting the method and examine and validate their contribution to the algorithm's performance under the considered cascaded control structure.
更多查看译文
关键词
mechatronics,motion control,cascaded control,reinforcement learning (RL),uncertain systems
AI 理解论文
溯源树
样例
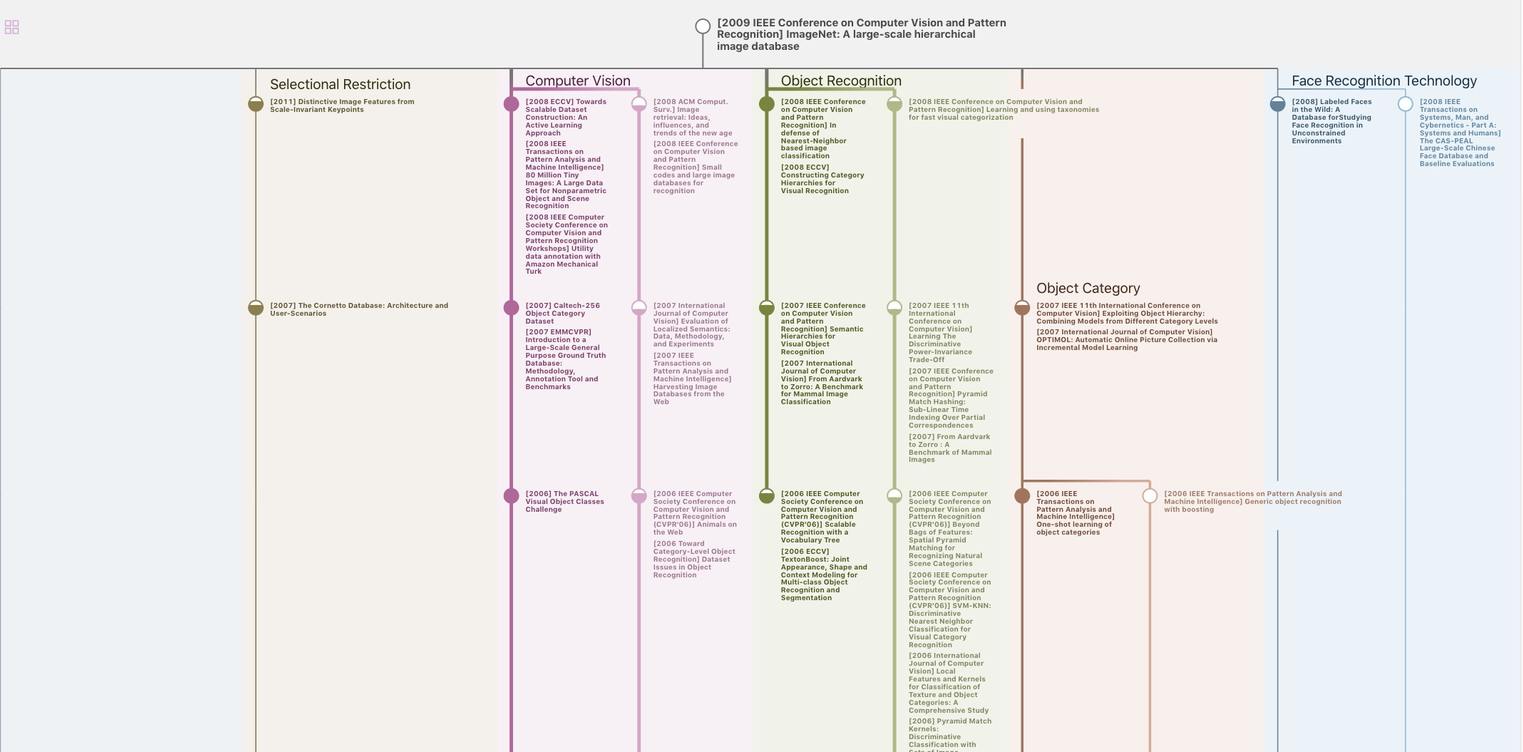
生成溯源树,研究论文发展脉络
Chat Paper
正在生成论文摘要