Adaptive genetic algorithm-based particle herding scheme for mitigating particle impoverishment
MEASUREMENT(2023)
摘要
Particle filters (PFs) estimate the true parameter state from samples of states, called particles, that are drawn to construct an approximated posterior probability density function (PDF). Each drawn particle has its own probability of being the true state called importance weight. Both particle degeneracy and impoverishment are important problems found in generic PFs that cause particles to become trapped at local maxima. Genetic algorithms (GAs) were applied to herd the drawn particles to discover new high-likelihood state values in order to reshape the approximated posterior PDF and to enhance the accuracy of state estimation, while the particle diversity is maintained. We calculated the threshold weight for classifying particles as low-weight particles and high-weight particles. Each parent pair consists of a high-weight particle and a low-weight particle. High -weight particles are always kept unchanged. After each new offspring particle is found using GA operations, we must check its weight to ensure that it is actually superior to its low-weight parent. Otherwise, this offspring must not be allowed to replace its low-weight parent and must be destroyed. To ensure that the number of high-weight particles is adequate, some offspring particles evolve to be the new high-weight parent particles to produce high-weight offspring with further promoted diversity. Results showed that our approach significantly improves posterior PDF approximation and reduces errors in state estimation.
更多查看译文
关键词
Genetic algorithms (GAs),Particle degeneracy,Particle diversity,Particle filtering (PF),Particle herding,Particle impoverishment,Posterior PDF,Probability density function (PDF),Signal processing
AI 理解论文
溯源树
样例
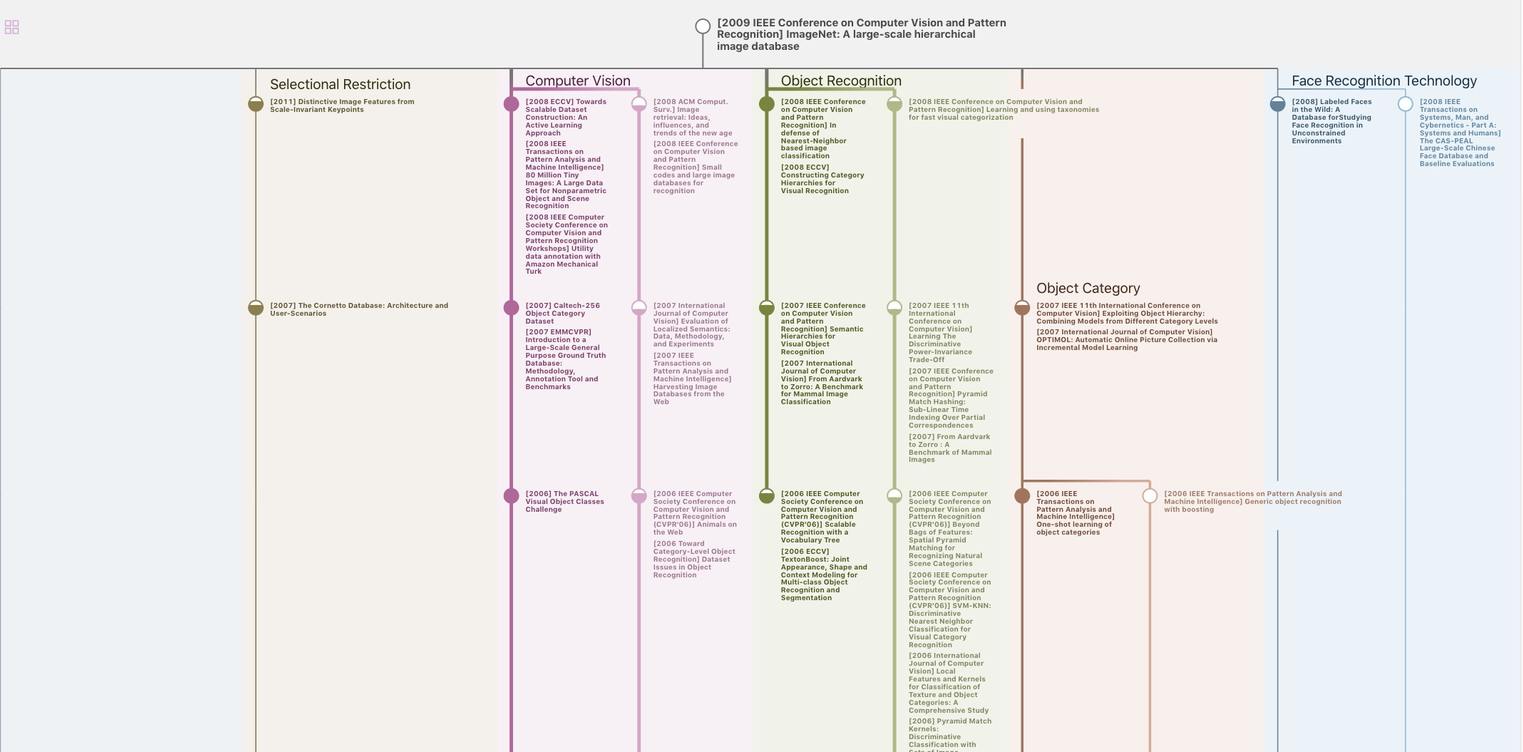
生成溯源树,研究论文发展脉络
Chat Paper
正在生成论文摘要