Shift-invariant universal adversarial attacks to avoid deep-learning-based modulation classification
INTERNATIONAL JOURNAL OF COMMUNICATION SYSTEMS(2023)
摘要
With the development of deep-learning technology, how to prevent signal modulation from being correctly classified by deep-learning-based intruders becomes a challenging issue. Adversarial attack provides an ideal solution as deep-learning models are proved to be vulnerable to intentionally designed perturbations. However, applying adversarial attacks to communication systems faces several practical problems such as shift-invariant, imperceptibility, and bandwidth compatibility. To this end, a shift-invariant universal adversarial attack approach is proposed in this work for misleading deep-learning-based modulation classifiers used by intruders. Specifically, this work first introduces a convolutional neural network (CNN)-based UAP (universal adversarial perturbation) generation model that contains an finite impulse response (FIR) filter layer to control the bandwidth of the output perturbation. Besides, this work proposes a circular shift scheme that simulates the random signal cropping in the inference phase and thus ensures the shift-invariant property of adversarial perturbations. In addition, this work designs a composite loss function that improves the imperceptibility of the adversarial perturbation in both time and frequency domains without decreasing the effectiveness of the adversarial attack. Experimental results demonstrate the effectiveness of the proposed approach, achieving about 50% accuracy drop on the target model when the perturbation-to-signal ratio (PSR) is -10 dB. Furthermore, extensive experiments are conducted to validate the shift-invariant, imperceptibility, bandwidth compatibility, and transferability of the proposed approach for modulation classification tasks.
更多查看译文
关键词
adversarial attack,deep-learning,modulation classification,wireless communication
AI 理解论文
溯源树
样例
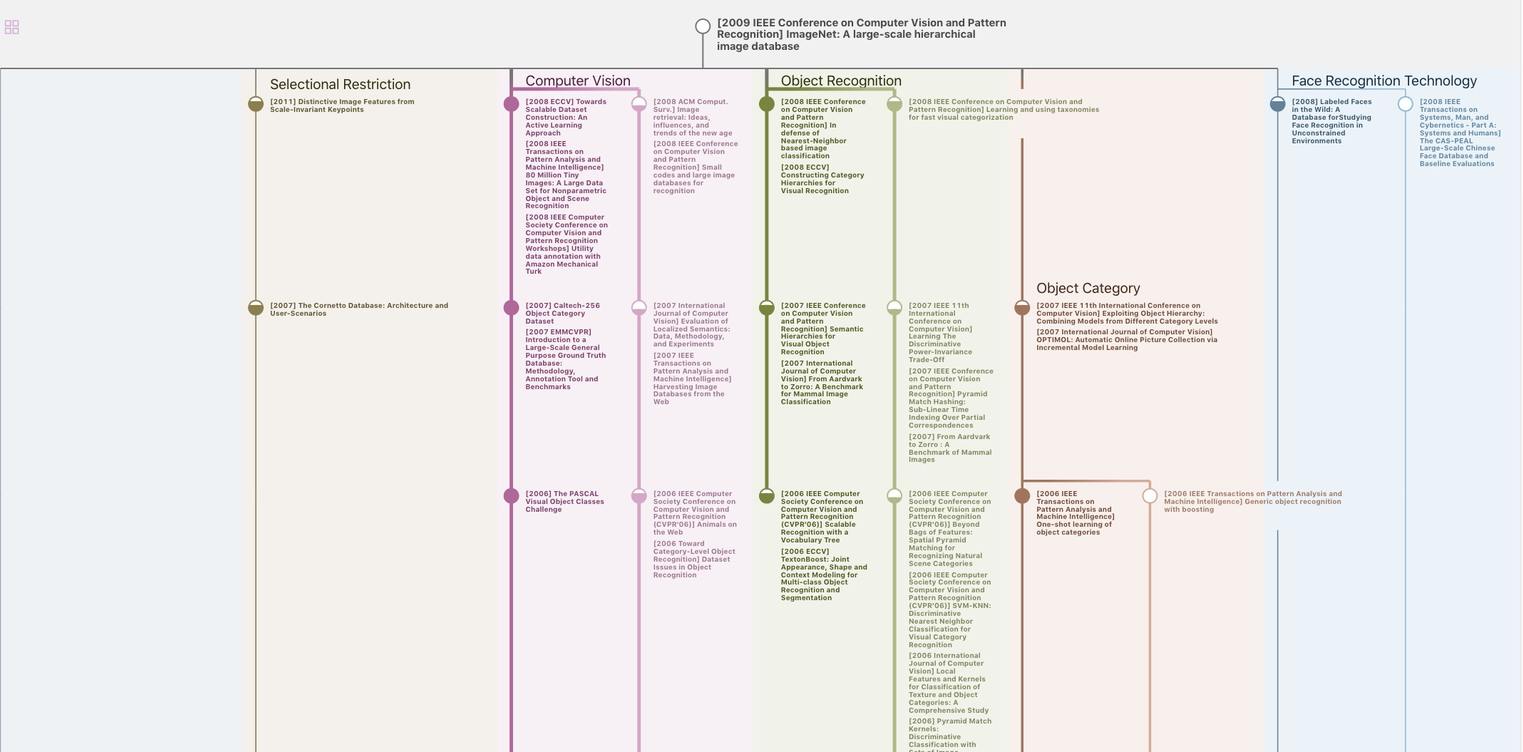
生成溯源树,研究论文发展脉络
Chat Paper
正在生成论文摘要