Scenario Generation for Financial Data with a Machine Learning Approach Based on Realized Volatility and Copulas
COMPUTATIONAL ECONOMICS(2023)
摘要
Portfolio optimisation is a core problem in quantitative finance and scenario generation techniques play a crucial role in simulating the future behaviour of the assets that can be used in allocation strategies. In the literature, there are different approaches to generating scenarios, from historical observations to models that predict the volatility of assets. In this paper, we propose a new methodology to generate one-day-ahead discrete scenarios, which are then used as input in choosing the portfolio that optimises the conditional value at risk (CVaR). Our approach uses machine learning supervised algorithms as forecasting models to predict the realized variance and intraday Kendall correlation of assets. With the predictions, we use an EVT-Copula approach to simulate the multivariate probability distribution of the assets. Our computational experiments suggest that our approach could produce more accurate volatility and correlation forecasts, and lower risk portfolios than traditional literature baselines.
更多查看译文
关键词
Machine learning,Supervised learning,Realized volatility,Portfolio optimisation
AI 理解论文
溯源树
样例
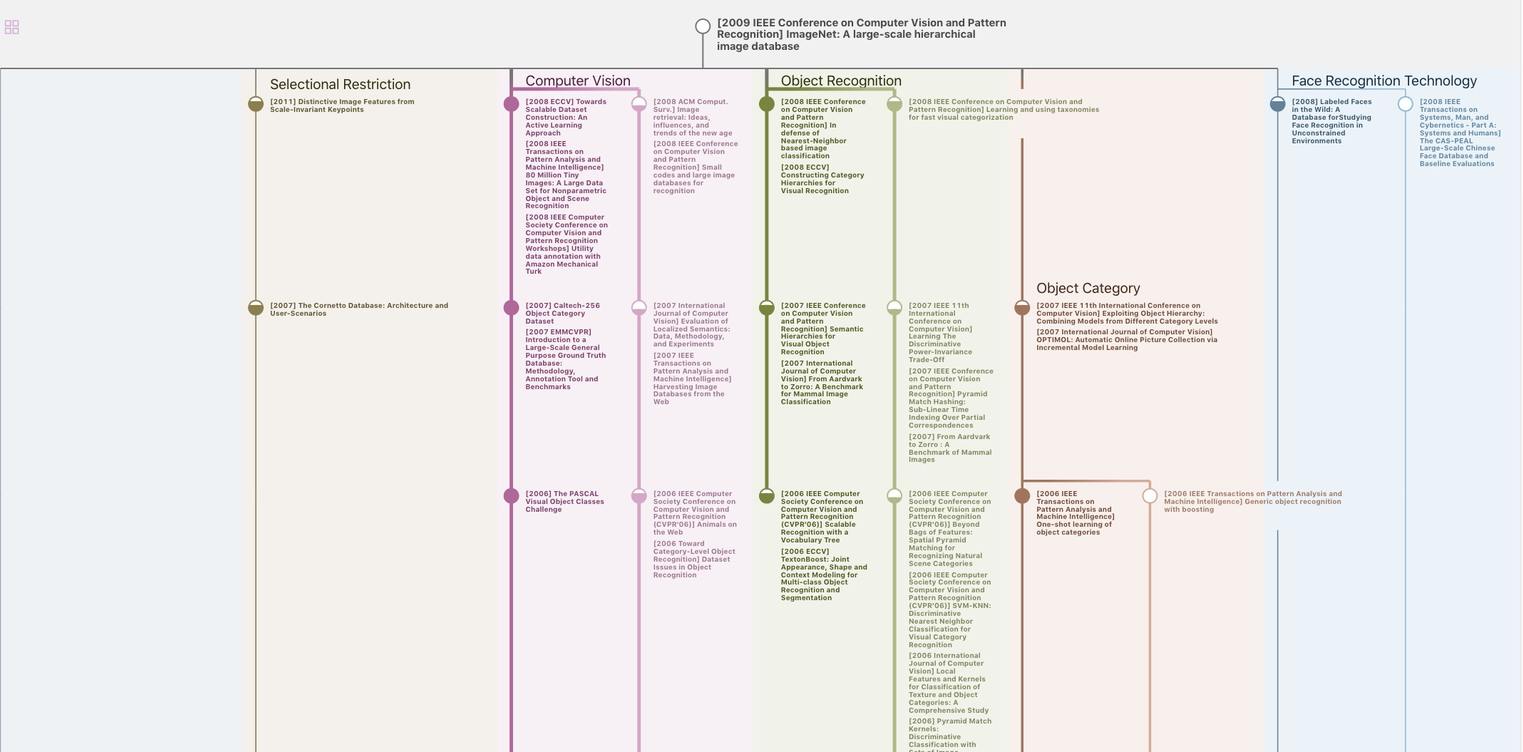
生成溯源树,研究论文发展脉络
Chat Paper
正在生成论文摘要