Trans-Lighter: A light-weight federated learning-based architecture for Remaining Useful Lifetime prediction
COMPUTERS IN INDUSTRY(2023)
摘要
Predictive maintenance (PdM) plays an important role in industrial manufacturing. One of the most fundamen-tal ideas underlying many PdM solutions is to estimate Remaining Useful Life (RUL) of machines. Recently, advanced deep learning models like convolutional neural network (CNN) and long short-term memory (LSTM) have been widely used for RUL prediction. However, these models also have certain limitations because of the difficulty in dealing with long-term dependencies in time series data. In this study, we propose a novel model based on transformer networks to overcome this difficulty. Rather than using the full structure of a transformer model, we exploit only the encoder combined with a linear layer. The Bayesian Optimization algorithm is applied to find optimal hyperparameters for the encoder. Experiments on widely used turbofan engine datasets show that our proposed method significantly outperforms the state-of-the-art RUL prediction methods by up to 25% in terms of predicting remaining usable life. We also provide a solution for the problem of preserving the privacy and security of data in smart manufacturing by designing a Federated Learning-based architecture for RUL using the proposed transformer-based model.
更多查看译文
关键词
Remaining Useful Lifetime,Federated learning,Bayesian optimization,Transformer
AI 理解论文
溯源树
样例
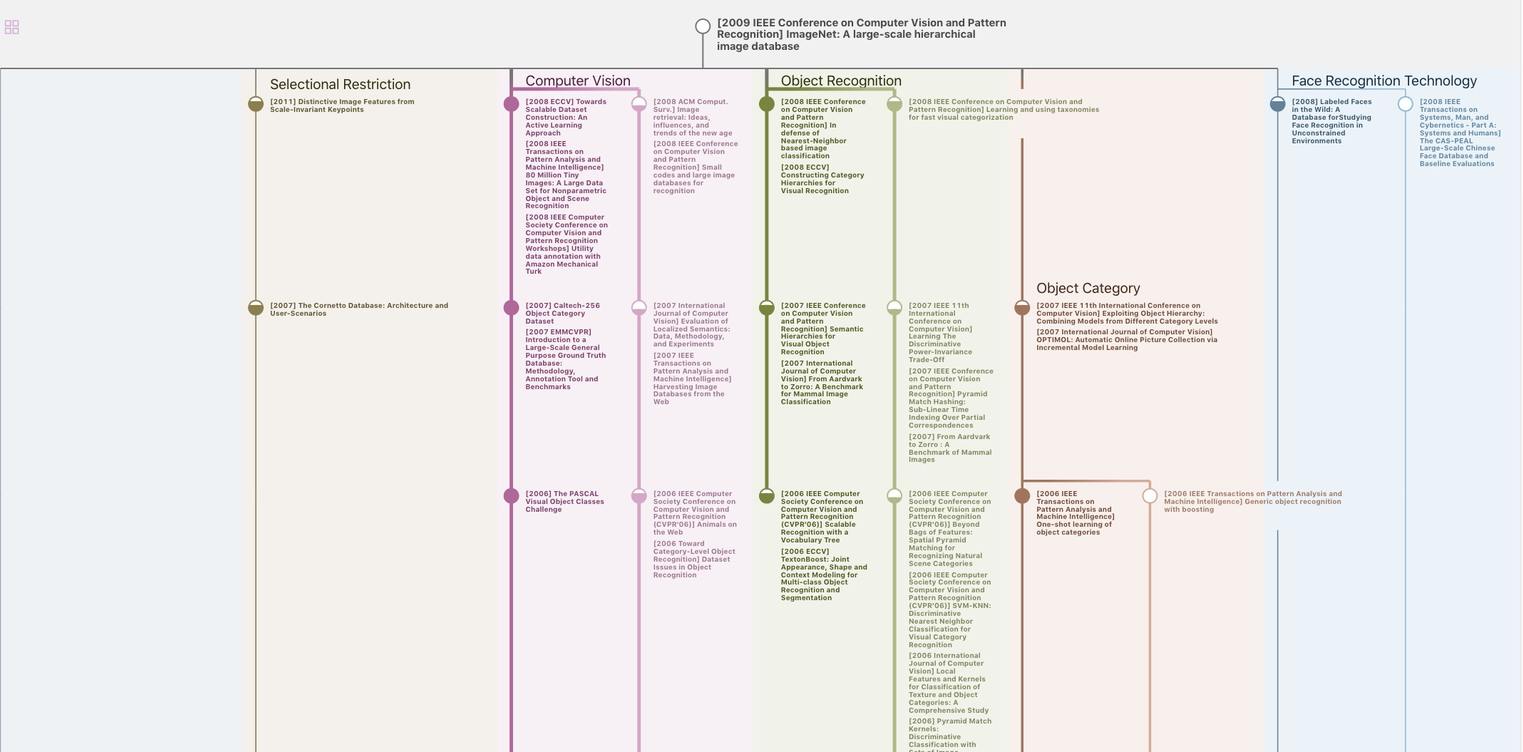
生成溯源树,研究论文发展脉络
Chat Paper
正在生成论文摘要