The influence of physical and algorithmic factors on simulated far-field waveforms and source-time functions of underground explosions using unsupervised machine learning
GEOPHYSICAL JOURNAL INTERNATIONAL(2023)
摘要
Characterizing explosion sources and differentiating between earthquake and underground explosions using distributed seismic networks becomes non-trivial when explosions are detonated in cavities or heterogeneous ground material. Moreover, there is little understanding of how changes in subsurface physical properties affect the far-field waveforms we record and use to infer information about the source. Simulations of underground explosions and the resultant ground motions can be a powerful tool to systematically explore how different subsurface properties affect far-field waveform features, but there are added variables that arise from how we choose to model the explosions that can confound interpretation. To assess how both subsurface properties and algorithmic choices affect the seismic wavefield and the estimated source functions, we ran a series of 2-D axisymmetric non-linear numerical explosion experiments and wave propagation simulations that explore a wide array of parameters. We then inverted the synthetic far-field waveform data using a linear inversion scheme to estimate source-time functions (STFs) for each simulation case. We applied principal component analysis (PCA), an unsupervised machine learning method, to both the far-field waveforms and STFs to identify the most important factors that control variance in the waveform data and differences between cases. For the far-field waveforms, the largest variance occurs in the shallower radial receiver channels in the 0-50 Hz frequency band. For the STFs, both peak amplitude and rise times across different frequencies contribute to the variance. We find that the ground equation of state (i.e. lithology and rheology) and the explosion emplacement conditions (i.e. tamped versus cavity) have the greatest effect on the variance of the far-field waveforms and STFs, with the ground yield strength and fracture pressure being secondary factors. Differences in the PCA results between the far-field waveforms and STFs could possibly be due to near-field non-linearities of the source that are not accounted for in the estimation of STFs and could be associated with yield strength, fracture pressure, cavity radius and cavity shape parameters. Other algorithmic parameters are found to be less important and cause less variance in both the far-field waveforms and STFs, meaning algorithmic choices in how we model explosions are less important, which is encouraging for the further use of explosion simulations to study how physical Earth properties affect seismic waveform features and estimated STFs.
更多查看译文
关键词
Machine learning,Computational seismology,Waveform inversion,Seismology
AI 理解论文
溯源树
样例
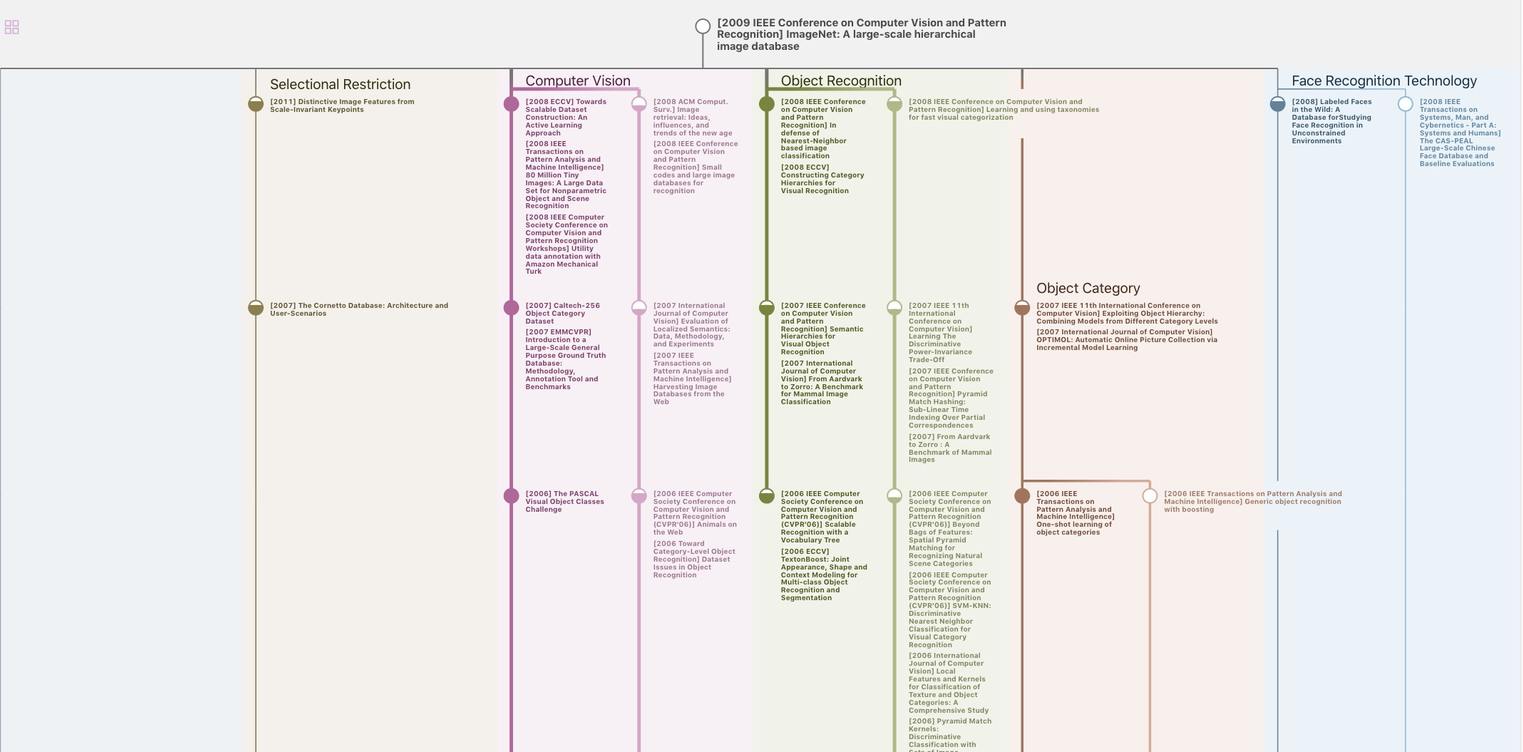
生成溯源树,研究论文发展脉络
Chat Paper
正在生成论文摘要