Impact of class imbalance in VeReMi dataset for misbehavior detection in autonomous vehicles
SOFT COMPUTING(2023)
摘要
Class imbalance is one of the common problems faced by the researchers in their learning or analyzing datasets. The learning precision and accuracy using the real-time datasets and simulated datasets vary at large scale. The learning conducted by simulated datasets may not be matching with the learning carried using real-time generated datasets; moreover, the learning vector deviates away for the real-time datasets. Similar kind of issue is observed in the VeReMi dataset that is used extensively to train the intelligent models for detecting misbehavior nodes in autonomous vehicles network. In this research, the learning impact of an intelligent model is evaluated, which is trained by the VeReMi dataset, treated for avoiding class imbalance and resampled based on well-known class imbalance techniques such as SMOTE and DSSTE, and observe the variations in the model’s performance, whose primary target is to identify behavioral anomalies in autonomous vehicles and systems. The work aims to evidently show how imbalanced the VeReMi dataset is and its impact on training intelligent models to classify the attacks.
更多查看译文
关键词
Class imbalance,Autonomous vehicles,SMOTE,DSSTE,VeReMi
AI 理解论文
溯源树
样例
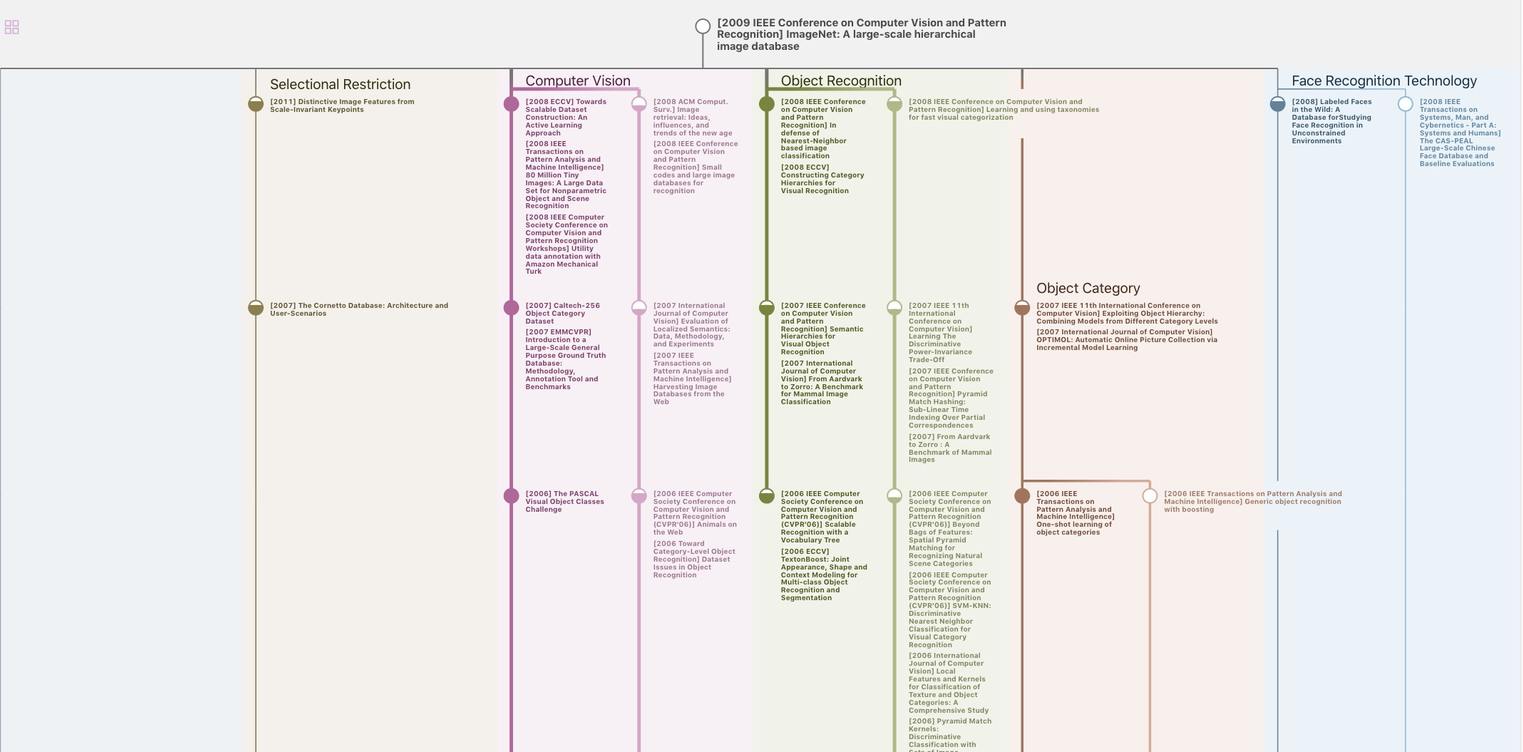
生成溯源树,研究论文发展脉络
Chat Paper
正在生成论文摘要