Decentralized federated learning with privacy-preserving for recommendation systems
ENTERPRISE INFORMATION SYSTEMS(2023)
摘要
Hyperautomation can automate complex business processes, reduce human intervention and improve business operational efficiency. Recommendation systems (RS) facilitate hyperautomation greatly. However, these systems require a large amount of user data to train their machine learning (ML) models and hence user data privacy has received great attention. In this paper, we propose a decentralized federated learning framework with privacy-preserving for RS. In our framework, users train the private and public parameters locally but share the public parameters only. Extensive experiments verify that our approach is accurate and can well preserve privacy. This study is helpful for providing privacy preserving in hyperautomation.
更多查看译文
关键词
-hyperautomation,decentralised federated learning,matrix factorisation,recommendation systems,privacy-preserving
AI 理解论文
溯源树
样例
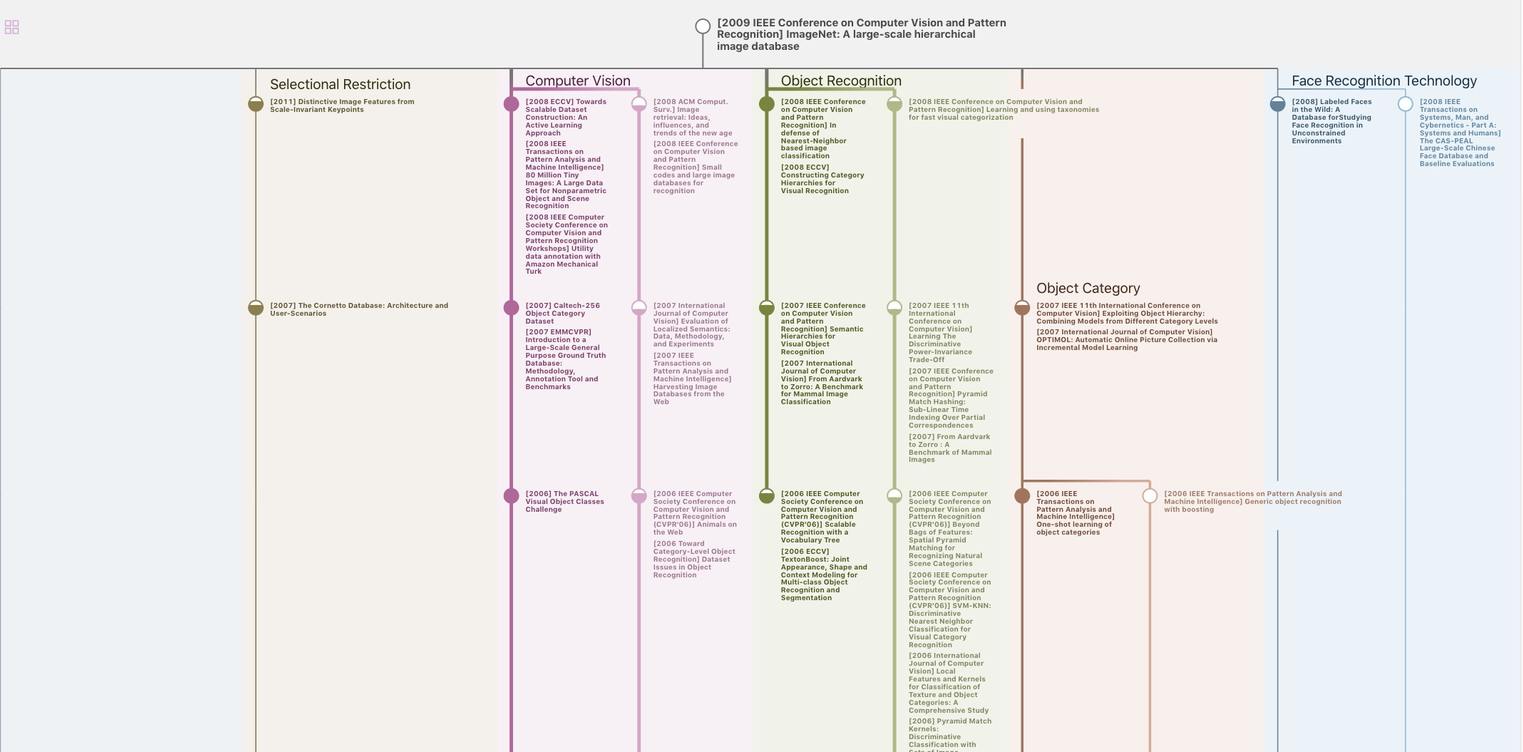
生成溯源树,研究论文发展脉络
Chat Paper
正在生成论文摘要