Recommendation model based on multi-grained interaction that fuses users’ dynamic interests
INTERNATIONAL JOURNAL OF MACHINE LEARNING AND CYBERNETICS(2023)
摘要
Users leave many reviews while participating in network activities, and these have been proven to improve the performance of recommendation systems. However, most current works in the field of rating prediction only capture preference information from the single granularity of each review. This inevitably incurs the loss of fine-grained information in reviews. In addition, people do not realize that the usefulness of reviews is also affected by the dynamics of time. User interests continuously change over time, and reviews at different stages have different meanings for user interest modeling. Therefore, we propose a new dynamic multi-granular interaction recommendation (DMIR) model. First, the model does not aggregate reviews into a single vector but rather extracts the feature information from the multi-granular fragments of each review by dilated convolution. Second, the model uses multi-granular feature information to construct the personalized fine-grained interactions between users and items. At the same time, the model assigns each user’s dynamic interest representation according to the user’s interaction with the item at different stages and obtains the corresponding user-item interactions based on the dynamic interest. Finally, we design a gating mechanism to fuse the ratings obtained by two interactions for the final rating prediction. After extensive experiments conducted on five standard datasets based on Amazon, the results show that DMIR achieves a substantial improvement over the existing state-of-the-art models in terms of rating prediction. Additionally, fusing the user’s dynamic interest and multi-granular text interactions not only improves the performance of the model but also enhances the robustness of rating prediction.
更多查看译文
关键词
Rating prediction,Multi-granularity,Multi-head attention,Dynamic modeling,Feature interaction
AI 理解论文
溯源树
样例
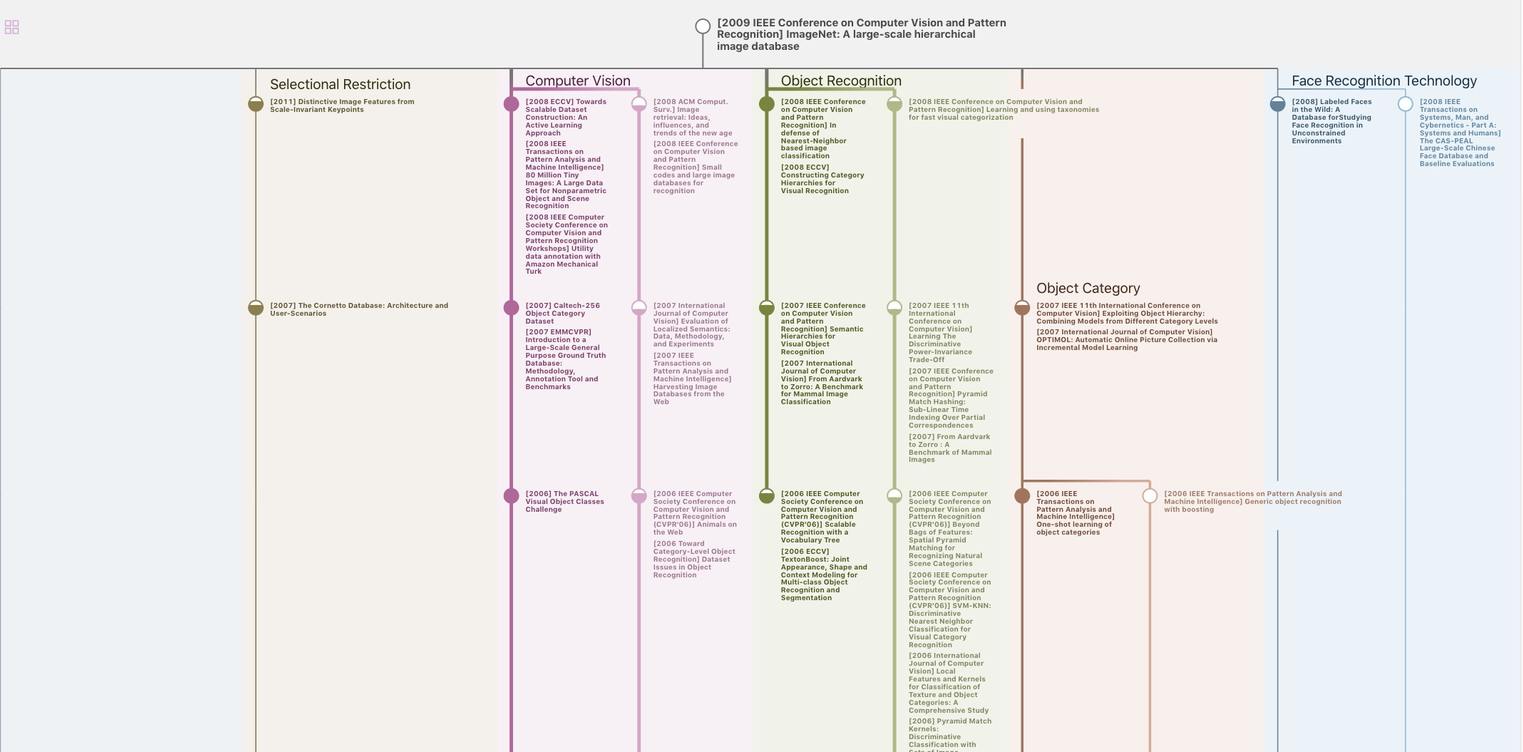
生成溯源树,研究论文发展脉络
Chat Paper
正在生成论文摘要