An Improved Low-Rank Matrix Fitting Method Based on Weighted L-1,L-p Norm Minimization for Matrix Completion
INTERNATIONAL JOURNAL OF PATTERN RECOGNITION AND ARTIFICIAL INTELLIGENCE(2023)
摘要
Low-rank matrix completion, which aims to recover a matrix with many missing values, has attracted much attention in many fields of computer science. A low-rank matrix fitting (LMaFit) method has been proposed for fast matrix completion recently. However, this method cannot converge accurately on matrices of real-world images. For improving the accuracy of LMaFit method, an improved low-rank matrix fitting (ILMF) method based on the weighted L-1,L-p norm minimization is proposed in this paper, where the L-1,L-p norm is the summation of the p-power eth 0 < p < 2 THORN of L-1 norms of rows in a matrix. In the proposed method, i.e. the ILMF method, the incomplete matrix that may be corrupted by noises is decomposed into the summation of a low-rank matrix and a noise matrix at first. Then, a weighted L-1,L-p norm minimization problem is solved by using an alternating direction method for improving the accuracy of matrix completion. Experimental results on real-world images show that the ILMF method has much better performances in terms of both the convergence accuracy and convergence speed than the compared methods.
更多查看译文
关键词
Low rank,L-1,L-p norm,matrix completion,QR decomposition
AI 理解论文
溯源树
样例
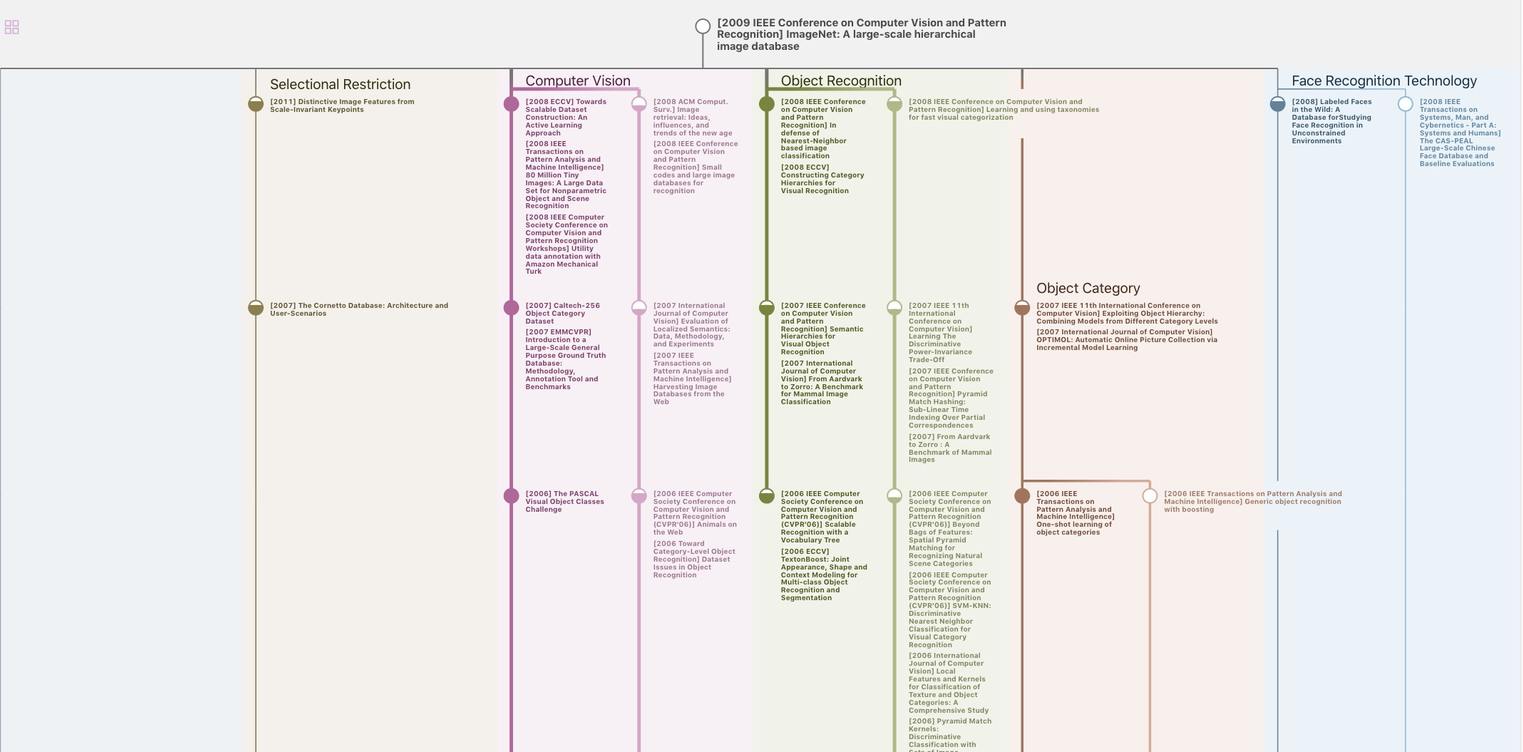
生成溯源树,研究论文发展脉络
Chat Paper
正在生成论文摘要