Creating and validating a scholarly knowledge graph using natural language processing and microtask crowdsourcing
INTERNATIONAL JOURNAL ON DIGITAL LIBRARIES(2023)
摘要
Due to the growing number of scholarly publications, finding relevant articles becomes increasingly difficult. Scholarly knowledge graphs can be used to organize the scholarly knowledge presented within those publications and represent them in machine-readable formats. Natural language processing (NLP) provides scalable methods to automatically extract knowledge from articles and populate scholarly knowledge graphs. However, NLP extraction is generally not sufficiently accurate and, thus, fails to generate high granularity quality data. In this work, we present TinyGenius, a methodology to validate NLP-extracted scholarly knowledge statements using microtasks performed with crowdsourcing. TinyGenius is employed to populate a paper-centric knowledge graph, using five distinct NLP methods. We extend our previous work of the TinyGenius methodology in various ways. Specifically, we discuss the NLP tasks in more detail and include an explanation of the data model. Moreover, we present a user evaluation where participants validate the generated NLP statements. The results indicate that employing microtasks for statement validation is a promising approach despite the varying participant agreement for different microtasks.
更多查看译文
关键词
Crowdsourcing microtasks,Knowledge graph validation,Scholarly knowledge graphs,User interface evaluation
AI 理解论文
溯源树
样例
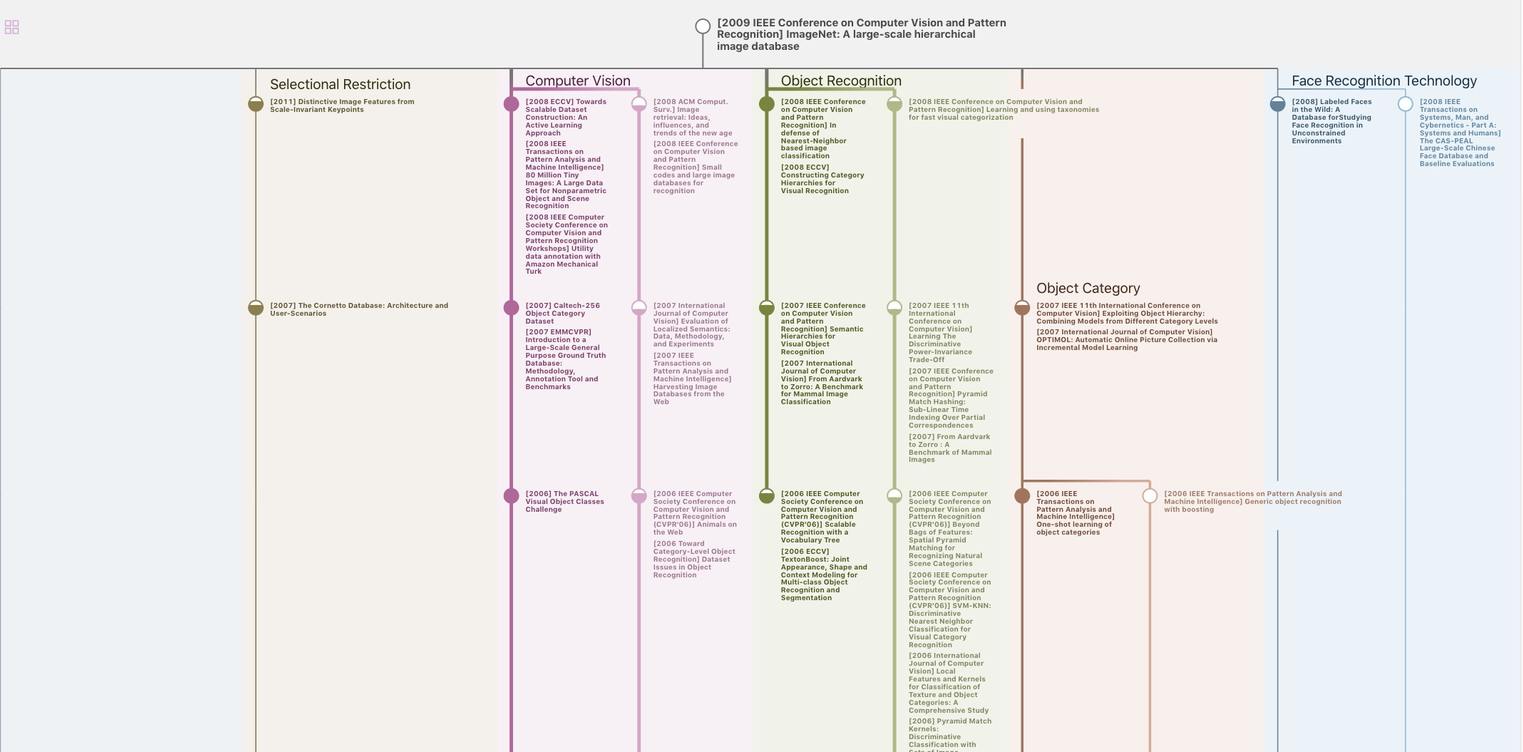
生成溯源树,研究论文发展脉络
Chat Paper
正在生成论文摘要