Design and implementation for a high-efficiency hardware accelerator to realize the learning machine for predicting OLED degradation
MICROSYSTEM TECHNOLOGIES-MICRO-AND NANOSYSTEMS-INFORMATION STORAGE AND PROCESSING SYSTEMS(2023)
摘要
A new learning machine based on neural network (NN) and its hardware accelerator are successfully built in this study for predicting the luminance decay of Organic Light Emitting Diode (OLED) displays. It is known that although OLED displays has become the mainstream in the current high-end display market, OLEDs tend to degrade in emission as used extensively for a long time. The operable voltage also rises with the usage time increasing, which causes the operating point to drift. To compensate the OLED degradation, a NN model is successfully built with favorable accuracy. Furthermore, the built NN model is implemented in FPGA hardware platform with a high-performance computing architecture, which uses registers to access inputs, integrates the multiplication, addition operations for weights and activation function into the same combinational logic circuit, and a pipeline architecture to improve maximum operation per unit time. The hardware architecture is designed via Verilog, and further verified by Xilinx Artix-7. Its operating frequency can be as high as 55.6 MHz, while resource consumption is only 1.0k LUTs, favorable as opposed to all the other past, related studies. Experiment shows that the computation of the built NN by the proposed accelerator can be completed 55.6 million times per second. In addition, the degradation prediction errors by the accelerator are as small as 2.08%, 5.51% and 4.36% for red, green and blue OLEDs, respectively, while the figure of merit, the product of computation time and area is as low as 109.86 (Time*Area), the lowest compared to all the past reported works.
更多查看译文
关键词
learning machine,hardware,degradation,high-efficiency
AI 理解论文
溯源树
样例
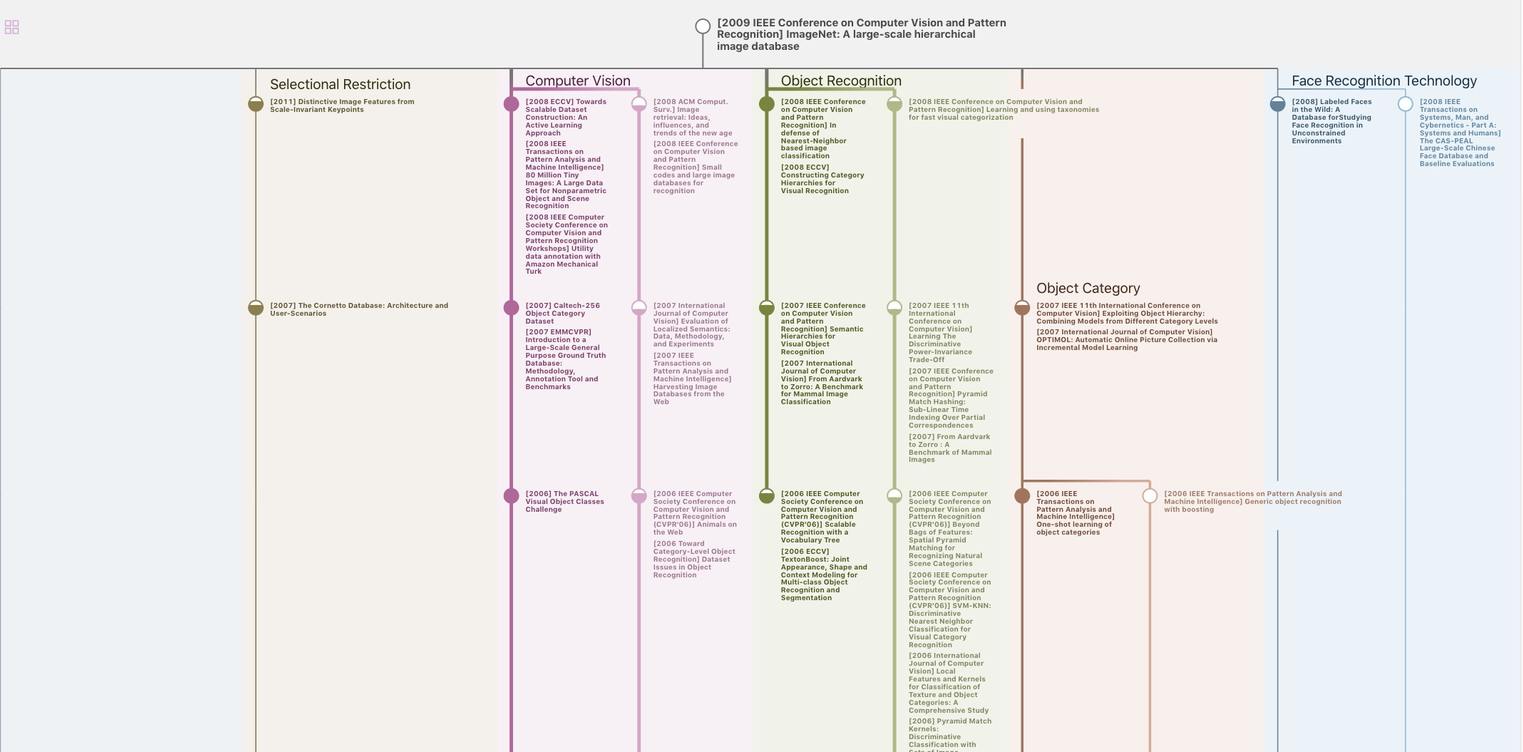
生成溯源树,研究论文发展脉络
Chat Paper
正在生成论文摘要