An Attentive Spatio-Temporal Learning-Based Network for Cardiovascular Disease Diagnosis
IEEE TRANSACTIONS ON SYSTEMS MAN CYBERNETICS-SYSTEMS(2023)
摘要
Automated diagnosis of cardiovascular diseases (CVDs) has become an imperative need for remote or in-hospital heart monitoring. This is a challenging task because of the tenuous morphological variation of the electrocardiogram (ECG) signal across different cardiac diseases. Existing works have attempted to learn the diagnostic representation by capturing the lead-specific morphological variation of a multilead ECG signal. In this work, we have developed an attentive spatio-temporal learning network (ASTLNet) that can learn better diagnostic representation by exploiting the concurrent spatio-temporal variation of a multilead ECG signal. The ASTLNet consists of two modules, i.e., spatio-temporal representation learning (STRL) module and attentive spatio-temporal aggregation (ASTA) module. The STRL module is designed to learn the multiscale spatio-temporal representation, and the ASTA module is designed to aggregate the learned representation. Experiments on the three publicly available datasets, i.e., PTB, PTB-XL, and CPSC-2018, demonstrate that the proposed model can effectively learn the spatio-temporal variation of the ECG signal and gives superior performance compared to the state-of-the-art methods.
更多查看译文
关键词
Electrocardiography,Lead,Convolutional neural networks,Solid modeling,Heart,Neural networks,Hidden Markov models,Cardiovascular disease (CVD),deep learning,electrocardiogram (ECG),LSTM,multihead criss-cross attention (MHCCA)
AI 理解论文
溯源树
样例
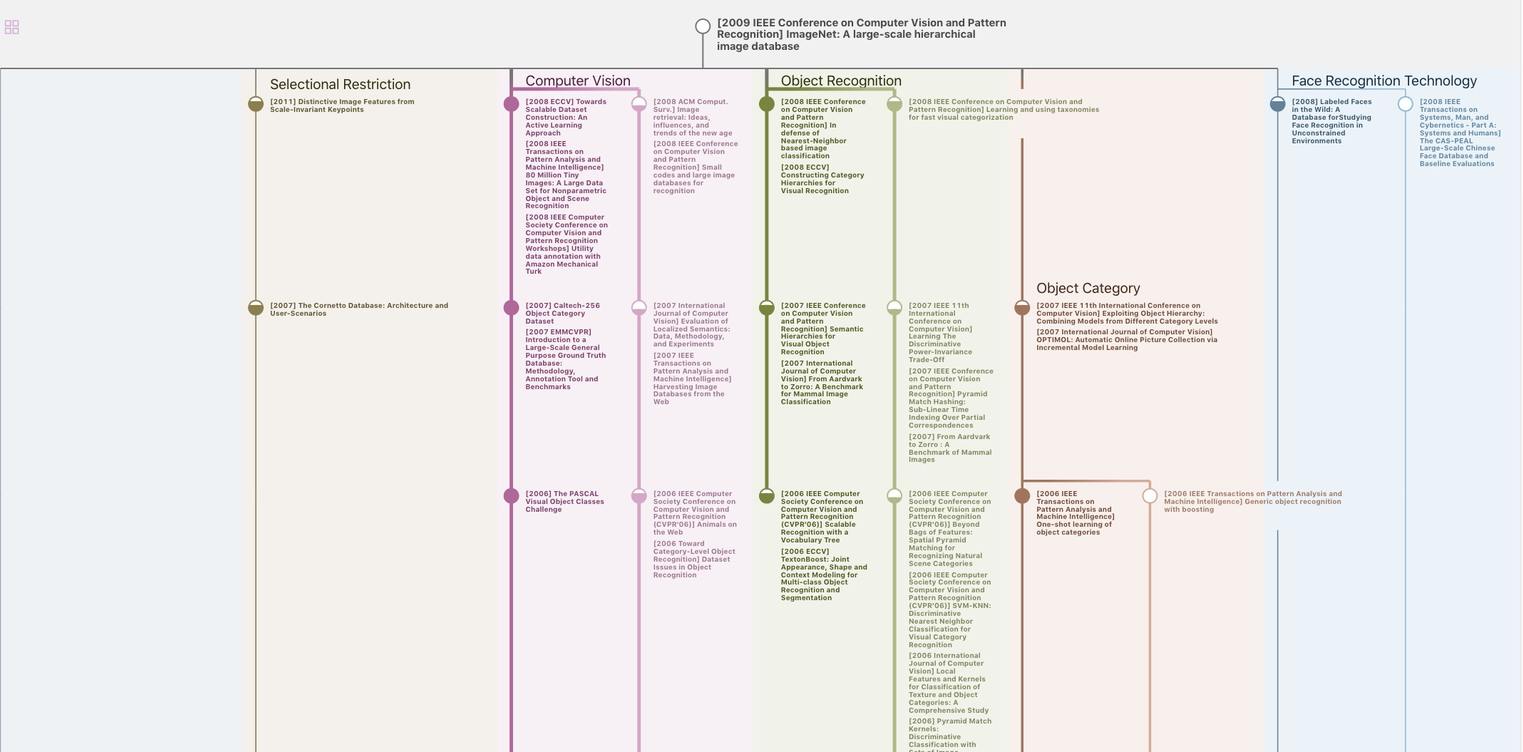
生成溯源树,研究论文发展脉络
Chat Paper
正在生成论文摘要