Monocular Road Scene Bird's Eye View Prediction via Big Kernel-Size Encoder and Spatial-Channel Transform Module
IEEE TRANSACTIONS ON INTELLIGENT TRANSPORTATION SYSTEMS(2023)
摘要
A detailed representation of the surrounding road scene is crucial for an autonomous driving system. yellow The camera-based Bird's Eye View map has been a popular solution to present the surrounding information, due to its low cost and rich spatial context information. Most of the existing methods predict the BEV map based on the depth-estimation or the trivial homography method, which may cause the error propagation and the absence of content. To overcome these drawbacks, we propose a novel end-to-end framework that employs the front monocular image to predict the road layout and vehicle occupancy. In particular, to capture the long-range feature, we redesign a CNN encoder with a large kernel size to extract the image features. For reducing the big difference between the front image features and the top-down features, we propose a novel Spatial-Channel projection module to convert the front map into the top-down space. Additionally, concerning the correlation between front view and top-down view, we propose the Dual Cross-view Transformer module to refine the top-down view feature maps and strengthen the transformation. Extensive evaluations on the KITTI and Argoverse datasets present that the proposed model achieves the state-of-the-art results for both datasets. Furthermore, the proposed model runs in 37 FPS on a single GPU, demonstrating the generation of a real-time BEV map. The code will be published at https://github.com/raozhongyu/BEV_LKA.
更多查看译文
关键词
Transformers,Feature extraction,Kernel,Transforms,Task analysis,Roads,Predictive models,Autonomous vehicles,bird's eye view map,Index Terms,deep learning
AI 理解论文
溯源树
样例
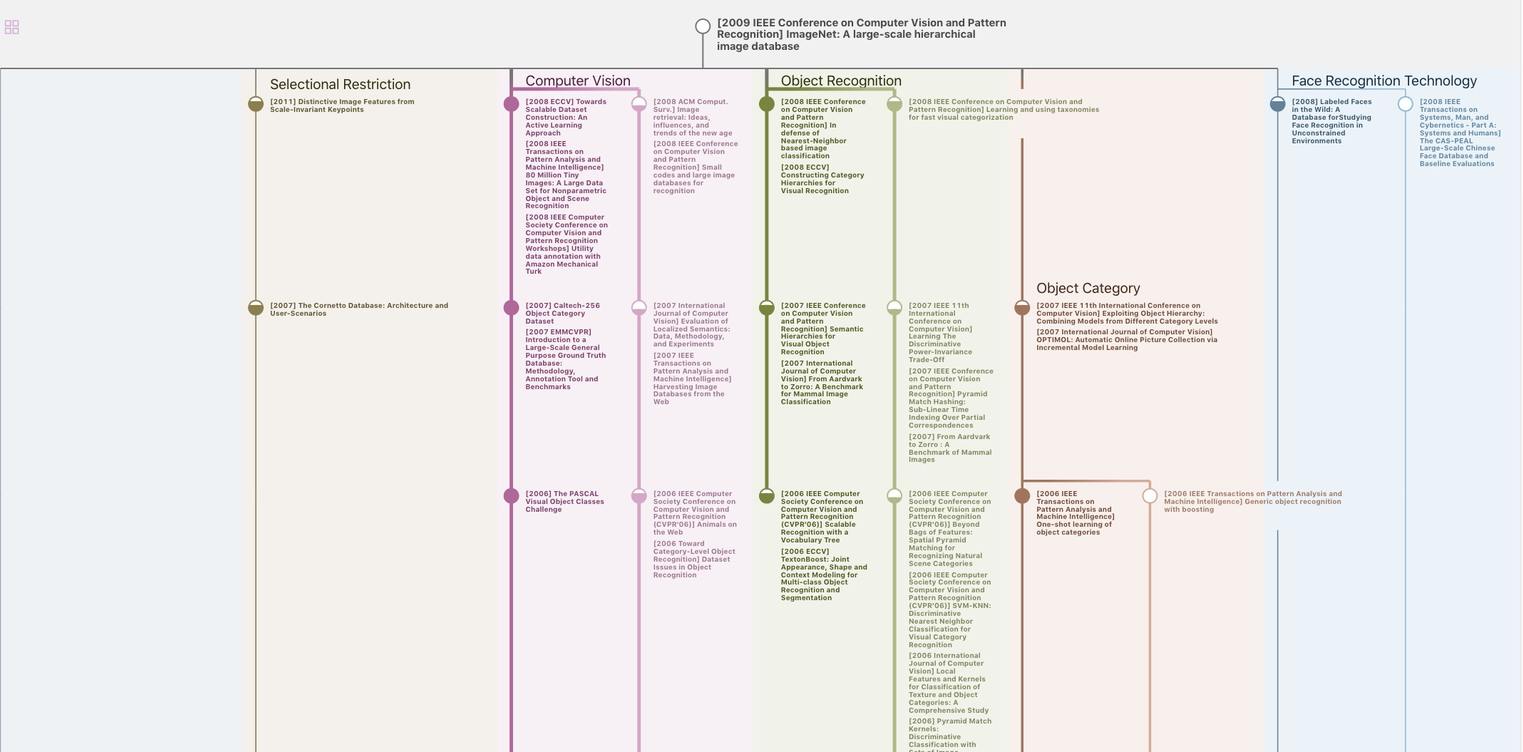
生成溯源树,研究论文发展脉络
Chat Paper
正在生成论文摘要