Adversarial Learning-Based Sentiment Analysis for Socially Implemented IoMT Systems
IEEE TRANSACTIONS ON COMPUTATIONAL SOCIAL SYSTEMS(2023)
摘要
Sentiment analysis is an important task in social computing and behavior analysis, and is a typical indicator of social health. It is a challenging mission to predict the sentiment of people in socially implemented Internet of Medical Things (IoMT) systems. The existing methods have several defects, and a typical defect is that most methods ignore the fact that there is much noise in IoMT systems and it is far not enough only to develop classification models for one type of data. In socially implemented IoMT systems, many methods treat the review text as plain text but ignore the potential knowledge structure. To solve those problems, in this article, we propose a novel solution, which is composed of adversarial learning and a hierarchical attention mechanism. We construct a hierarchical attention mechanism to learn the knowledge structure of a text. We propose to apply the attention mechanism both at the word level and sentence level, enabling us to learn the knowledge from each word and each sentence. We propose to use adversarial learning to learn new knowledge as non-random perturbations, which promotes the model's robustness. We evaluate our method on several large-scale real-world datasets, covering a wide range of cases of sentiment analysis. Experimental results demonstrate that our method achieves superior performance compared to state-of-the-art methods.
更多查看译文
关键词
Sentiment analysis,Adversarial machine learning,Task analysis,Computational modeling,Perturbation methods,Logic gates,Data models,Adversarial learning,Internet of Medical Things (IoMT) systems,sentiment analysis,social computing,social health prediction
AI 理解论文
溯源树
样例
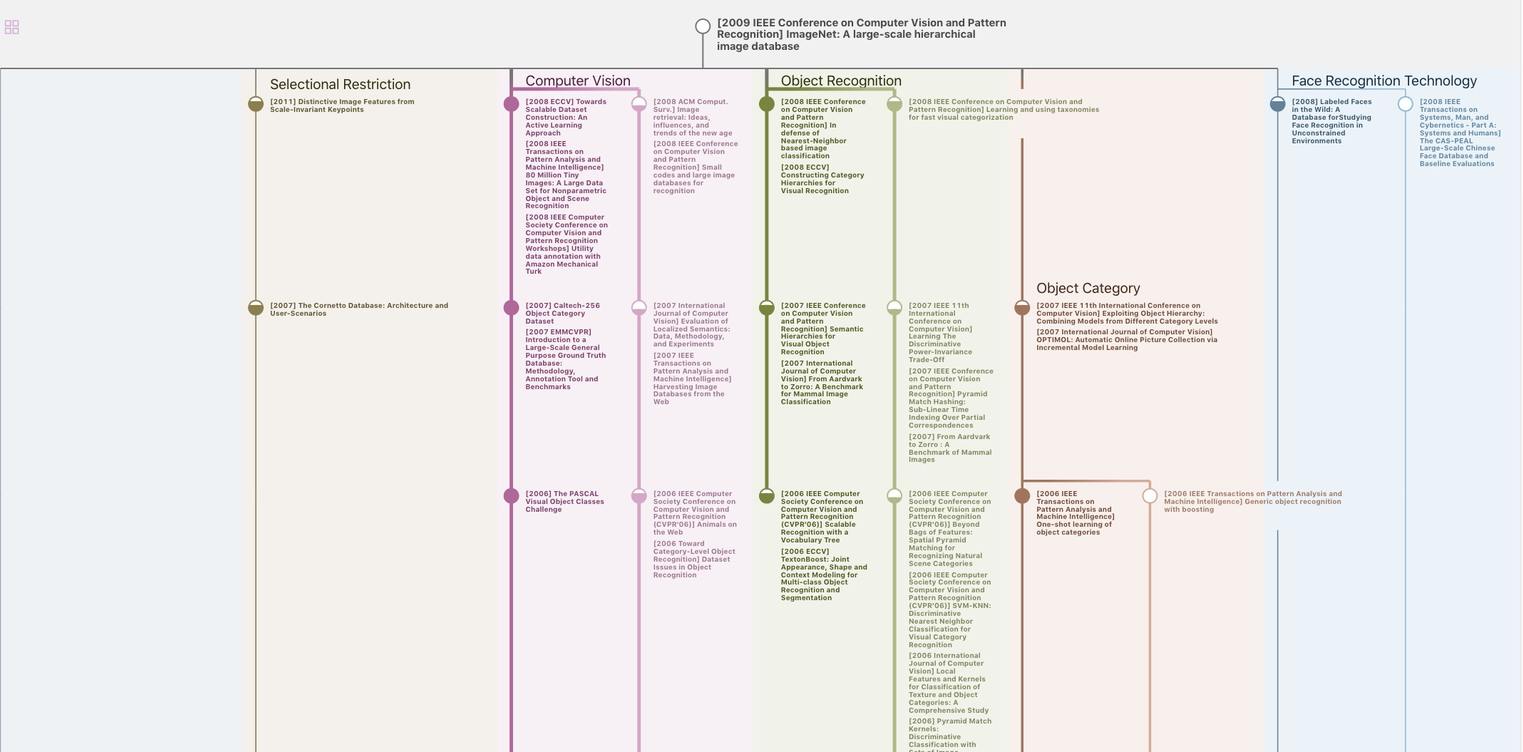
生成溯源树,研究论文发展脉络
Chat Paper
正在生成论文摘要