Surrogate Modeling of High-Speed Links Based on GNN and RNN for Signal Integrity Applications
IEEE TRANSACTIONS ON MICROWAVE THEORY AND TECHNIQUES(2023)
摘要
High-speed link consisting of drivers and interconnects is essential for high-speed data transmission. In this article, a surrogate modeling technique based on graph neural network (GNN) and recurrent neural network (RNN) is proposed for signal integrity (SI) analysis of high-speed links with variable physical parameters and variable topologies. First, GNN extracts global features that can fully characterize components of the high-speed link from their topologies and physical parameters. Second, RNN takes the extracted global features and the excitation waveforms as inputs to predict the response waveforms. Finally, the well-trained GNN -RNN surrogate models of components of the high-speed link are cascaded as the entire surrogate model of the high-speed link. Numerical examples of the dri- ver model, the interconnect model, and the entire high-speed link model are provided for validation. It is shown that the proposed GNN -RNN surrogate models achieve low mean squared errors (MSEs), mean absolute errors (MAEs), and high efficiency.
更多查看译文
关键词
Integrated circuit modeling,Numerical models,Topology,Graph neural networks,Predictive models,Feature extraction,Network topology,Graph neural network (GNN),high-speed links,recurrent neural network (RNN),signal integrity (SI),surrogate model
AI 理解论文
溯源树
样例
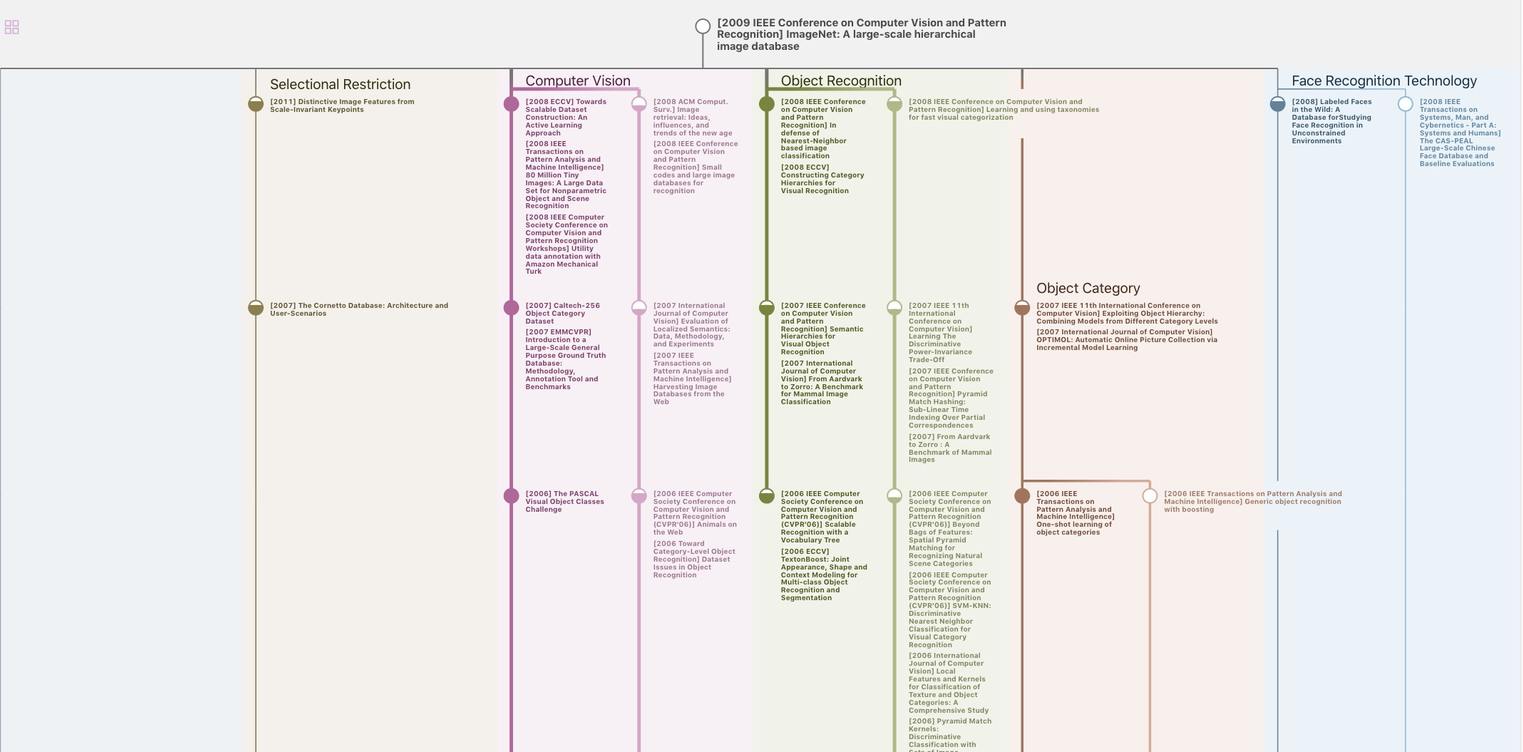
生成溯源树,研究论文发展脉络
Chat Paper
正在生成论文摘要