DCIFPN: Deformable cross-scale interaction feature pyramid network for object detection
IET IMAGE PROCESSING(2023)
摘要
Exploiting multi-scale features is one of the most effective methods to recognize objects of different scales in object detection. Since image pyramid is time-consuming, Feature Pyramid Network (FPN) becomes the most popular component used for obtaining pyramidal features. Despite its effectiveness, there still exist some intrinsic defects. In this work, it is attributed to insufficient information flow and a Deformable Cross-scale Interaction Feature Pyramid Network (DCIFPN) is proposed, which aims to promote the information transfer process with content-aware sampling and dynamic aggregation weights. More specifically, Deformable Semantic Enhancement Module (DSEM) is designed that can construct accurate information flow with dynamic aggregation weights. In addition, Deformable Spatial Refinement Module (DSRM) is proposed to enhance high-level features with low-level location details. When DCIFPN is deployed on RetinaNet and FCOS with ResNet-50, the performance is improved by 1.6 AP and 1.1 AP, respectively, on the challenging MS COCO benchmark. Apart from one-stage detectors, DCIFPN is also applicable to two-stage methods such as Faster R-CNN and Mask R-CNN. Further experiments on Pascal VOC and CrowdHuman datasets can verify the effectiveness and generalization of the method.
更多查看译文
关键词
feature extraction,object detection
AI 理解论文
溯源树
样例
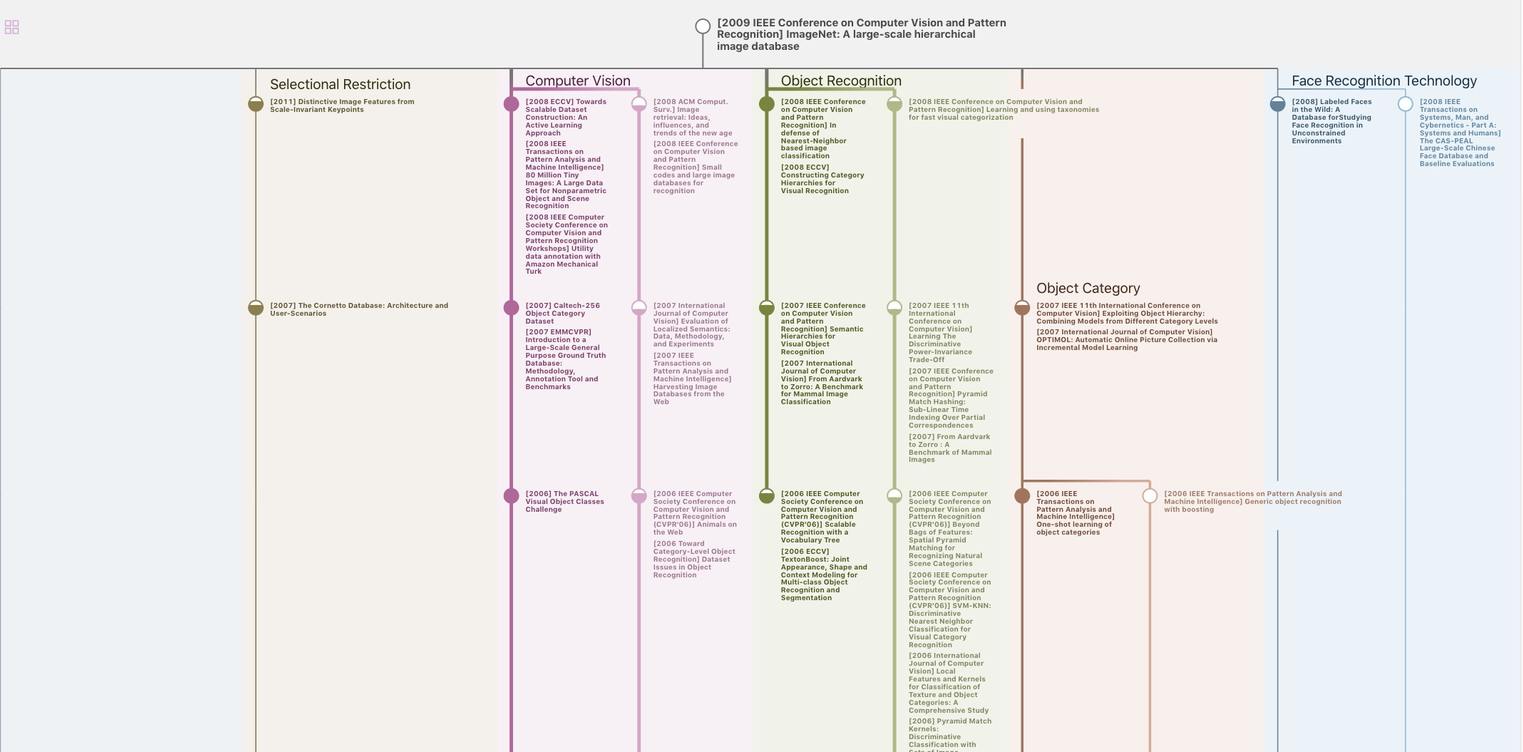
生成溯源树,研究论文发展脉络
Chat Paper
正在生成论文摘要