A novel automatic acne detection and severity quantification scheme using deep learning
BIOMEDICAL SIGNAL PROCESSING AND CONTROL(2023)
摘要
Accurate detection and severity quantification of acne are of great significance in the precise treatment of patients. Due to the similar appearance of acne with close severity, it is challenging for dermatologists to grade acne accurately and efficiently. This study aims to propose an accurate and efficient scheme based on deep learning (DL) to assist dermatologists in acne detection and severity quantification. The proposed frame consists of two steps: the Localization deep learning (Localization-DL) model and the Class segmentation (ClassSeg) model. The first model uses the distilled lightweight convolution network as the backbone and extracts multi-scale features through a pyramid pooling module for facial region localization and distinction. The second model is a unified framework that combines a Class module to distinguish background and facial skin sub-images and a segmentation (Seg) module to perform segmentation for different classes to obtain lesion masks. The facial skin segmentation branch of the ClassSeg model is built based on a high-resolution network (HRNet) and modified by mask-aware attention, shuffle attention, and conditional channel weight block. The experiments show that the two models achieve promising results and demonstrate effectiveness in lesion detection compared to other methods. The proposed scheme shows excellent results in acne severity quantification and yields a comparable performance with dermatologists (accuracy: 0.9091 for ours, 0.9301 for SDerms, 0.8741 for IDerms, and 0.7483 for JDerms). The assessment performance also outperforms the existing approaches. This work opens new avenues for acne severity quantification and provides valuable diagnosis evidence for dermatologists in clinical practice.
更多查看译文
关键词
Deep learning (DL),Acne lesion,Severity quantification,Segmentation
AI 理解论文
溯源树
样例
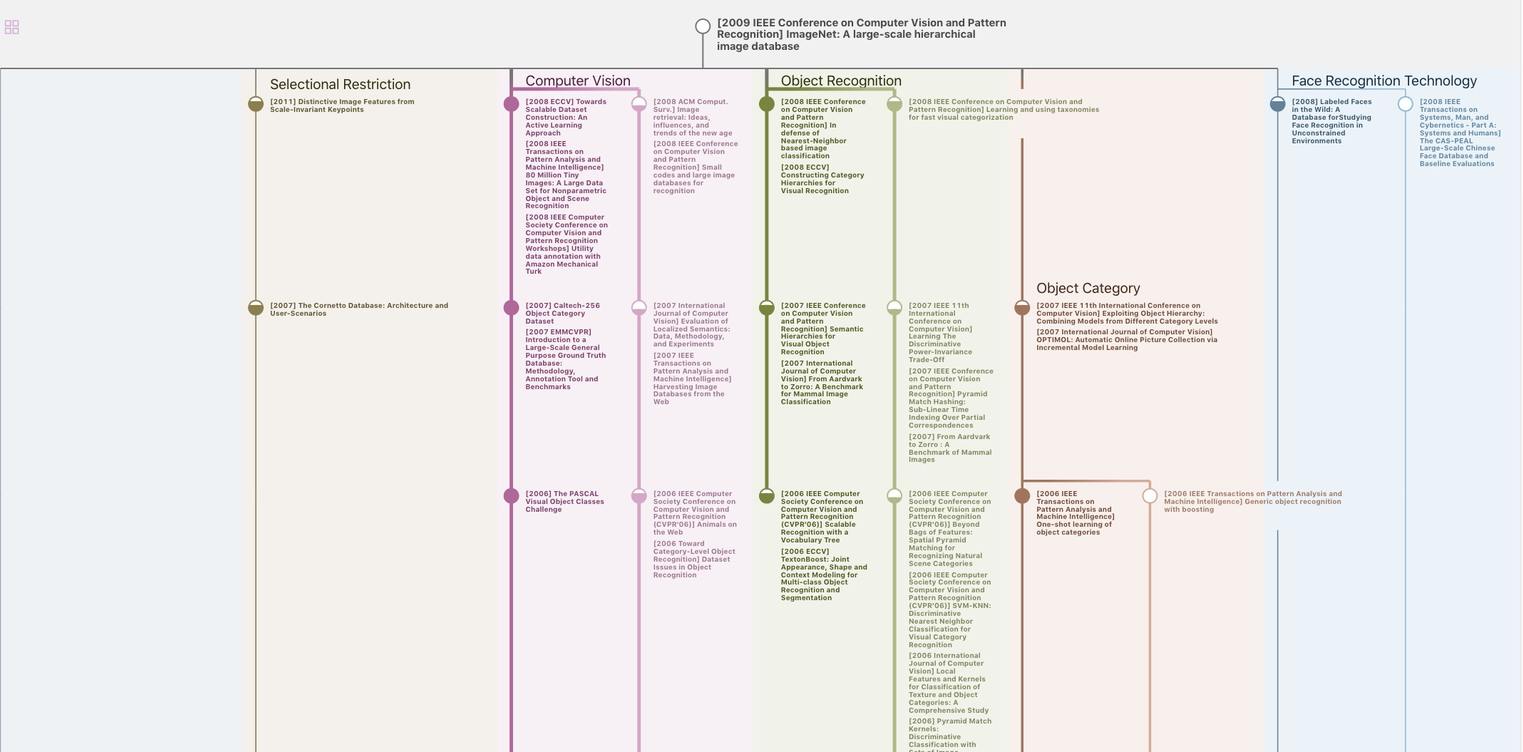
生成溯源树,研究论文发展脉络
Chat Paper
正在生成论文摘要