Hypergraph and cross-attention-based unsupervised domain adaptation framework for cross-domain myocardial infarction localization
INFORMATION SCIENCES(2023)
摘要
Solving individual differences between subjects is critical for the promotion of electrocardiogram (ECG) classification algorithms in the intelligent health monitoring industry. Popular inter-subject-based solutions usually require the manual labeling of heartbeats and frequent updating of the model for new subjects. To track these problems, we propose a hypergraph and cross-attention-based unsupervised domain adaptation (HGCA-UDA) framework for the myocardial infarction localization. Specifically, we first build a hypergraph-based dual-channel network, that can simultaneously learn specific feature representations from an ECG lead and disease category levels for samples from different domains. We then design a cross-attention module to align cross-domain locally similar samples. Subsequently, a domain alignment strategy based on the Wasserstein distance is proposed to align the global edge feature distribution. Finally, a pseudo-label generation scheme is proposed to further align fine-grained category information. We conduct extensive experiments on two public benchmark datasets (the Physikalisch-Technische Bundesanstalt (PTB) and PTB_XL database), and the results show that the proposed HGCR-UDA (with unlabeled patients) achieves comparable results compared with state-of-the-art inter-patient-based methods (with labeled patients) and has excellent applications prospects in the field of intelligent health monitoring.
更多查看译文
关键词
Hypergraph,Unsupervised domain adaptation,Patient individual differences,Myocardial infarction,Electrocardiogram
AI 理解论文
溯源树
样例
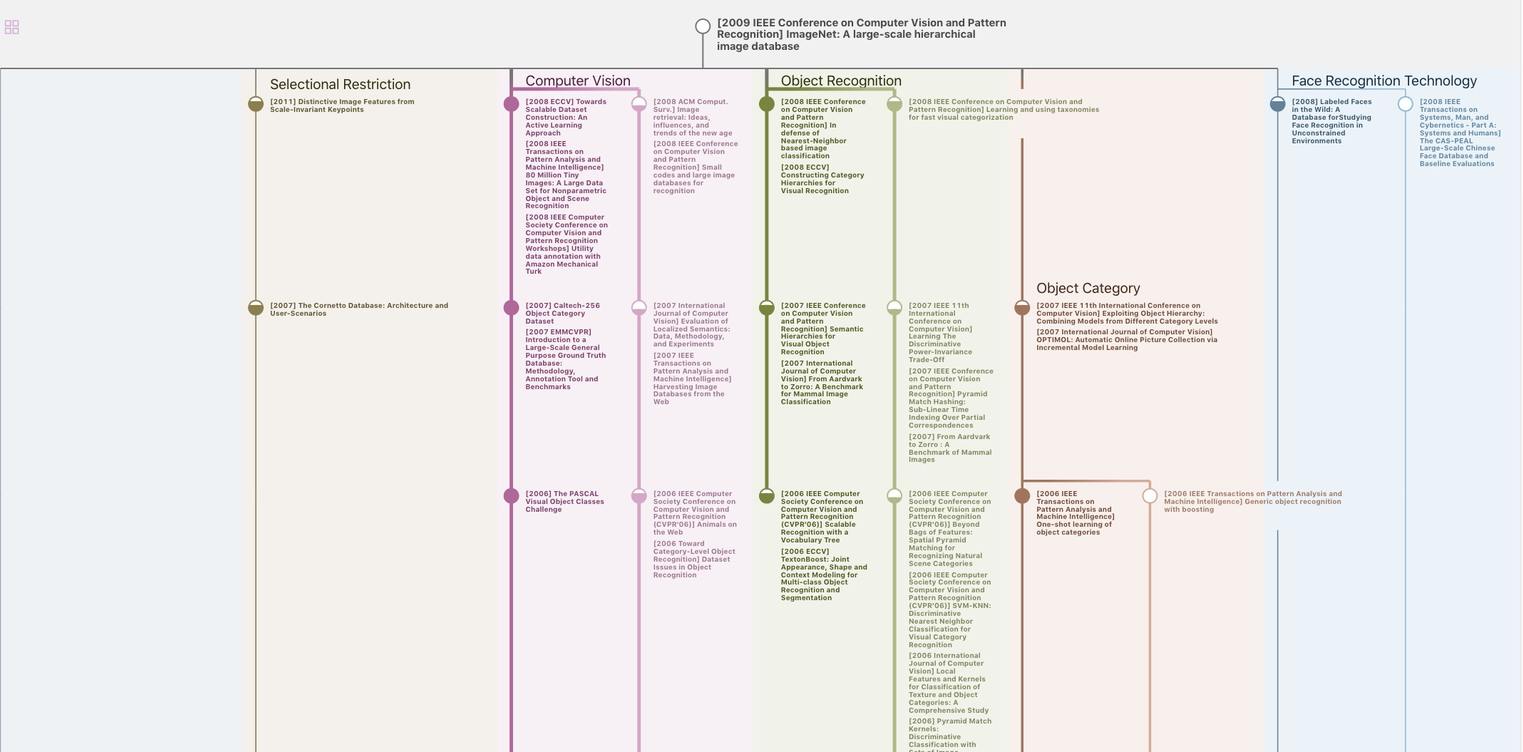
生成溯源树,研究论文发展脉络
Chat Paper
正在生成论文摘要