The role of phase transition by inception and surface reactions for the synthesis of silicon nanoparticles in a hot-wall reactor-Simulation and experiment
CHEMICAL ENGINEERING JOURNAL(2023)
摘要
A novel Eulerian particle model tailored for the application in computational fluid dynamics (CFD) is presented. The model is based on a bivariate moment method and features a full coupling with the gas-phase by kinetic formulations for inception, and activated and non-activated surface reactions. A new formulation for the particle composition accounts for the volatile content and its emission. The model is applied in the context of silicon nanopowder synthesis from monosilane, where a validation is performed with a well-established hot-wall reactor experiment from the literature. It is characterized by high precursor concentrations at moderate heating temperature of 853 K. Further, a new experiment was conducted for the comparison with the new model approach in a three-dimensional, transient simulation. The semi-industrial hot wall reactor features an unsteady complex flow field with buoyancy driven recirculation. The simulations enable new insights into the unsteady flow, mixing and nanoparticle formation process and contribute to their understanding. For the validation, residence-time dependent data for the precursor concentration, the primary particle size, and the particle number concentration are compared with results of the simulation at different stages. A com-parison with the results of stochastic methods serves as further benchmark for the presented model. An excellent agreement with the experimental data is obtained, if essential growth mechanisms are considered. For the semi-industrial hot-wall reactor experiment, the BET surface and particle diameter, as well and the precursor consumption, are compared to the results of the new model and to a description with inception, coagulation, and sintering only. Due to the absence of crucial activated and non-activated surface reactions, the latter model predicts values that deviate more than one order of magnitude from the experimental data, while the new model shows an overall good agreement.
更多查看译文
AI 理解论文
溯源树
样例
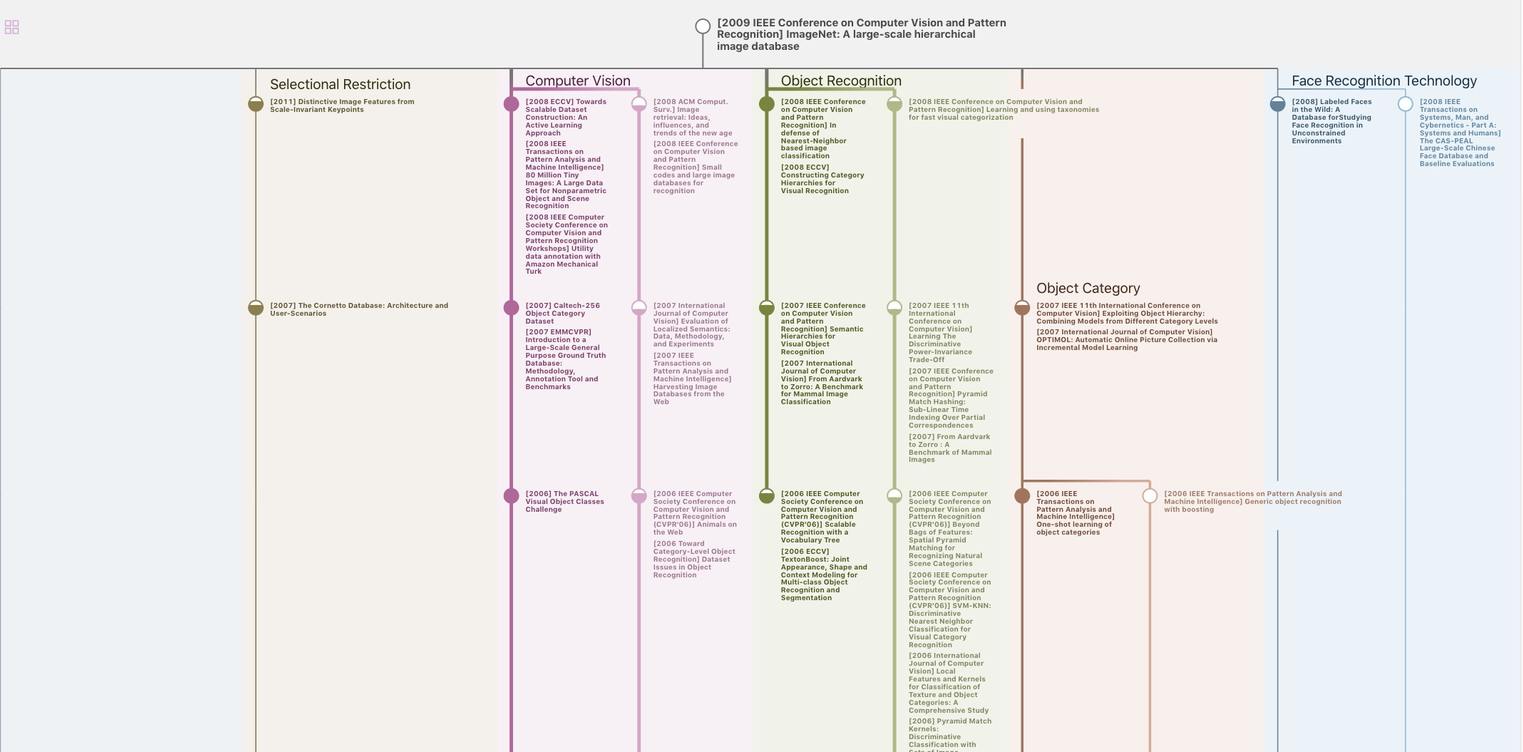
生成溯源树,研究论文发展脉络
Chat Paper
正在生成论文摘要