EEG channel selection-based binary particle swarm optimization with recurrent convolutional autoencoder for emotion recognition
BIOMEDICAL SIGNAL PROCESSING AND CONTROL(2023)
摘要
Electroencephalography (EEG) signals can demonstrate the activities of the human brain and recognize different emotional states. Emotion recognition based on full EEG channels leads to the use of redundant data and increases the hardware complexity. This paper presents a channel selection method based on a new Binary Many-Objective Particle Swarm Optimization with Cooperative Agents (BMaOPSO-CA). More specifically, we perform unsupervised feature learning with recurrent convolutional layers based on autoencoder architecture directly from clean EEG signals. Extensive validation on three public effective benchmarks, i.e. DASPS, DEAP, and SEED which are different in channel number used stimuli, and participant ratings, was carried out with subject independent scheme. As result, the experimental study highlights the optimal electrode locations related to emotions, leading to analyze the relationship between specific brain regions and emotions.
更多查看译文
关键词
Unsupervised EEG feature learning,Convolutional LSTM,Channel selection,Binary particle swarm optimization,Local learning strategy
AI 理解论文
溯源树
样例
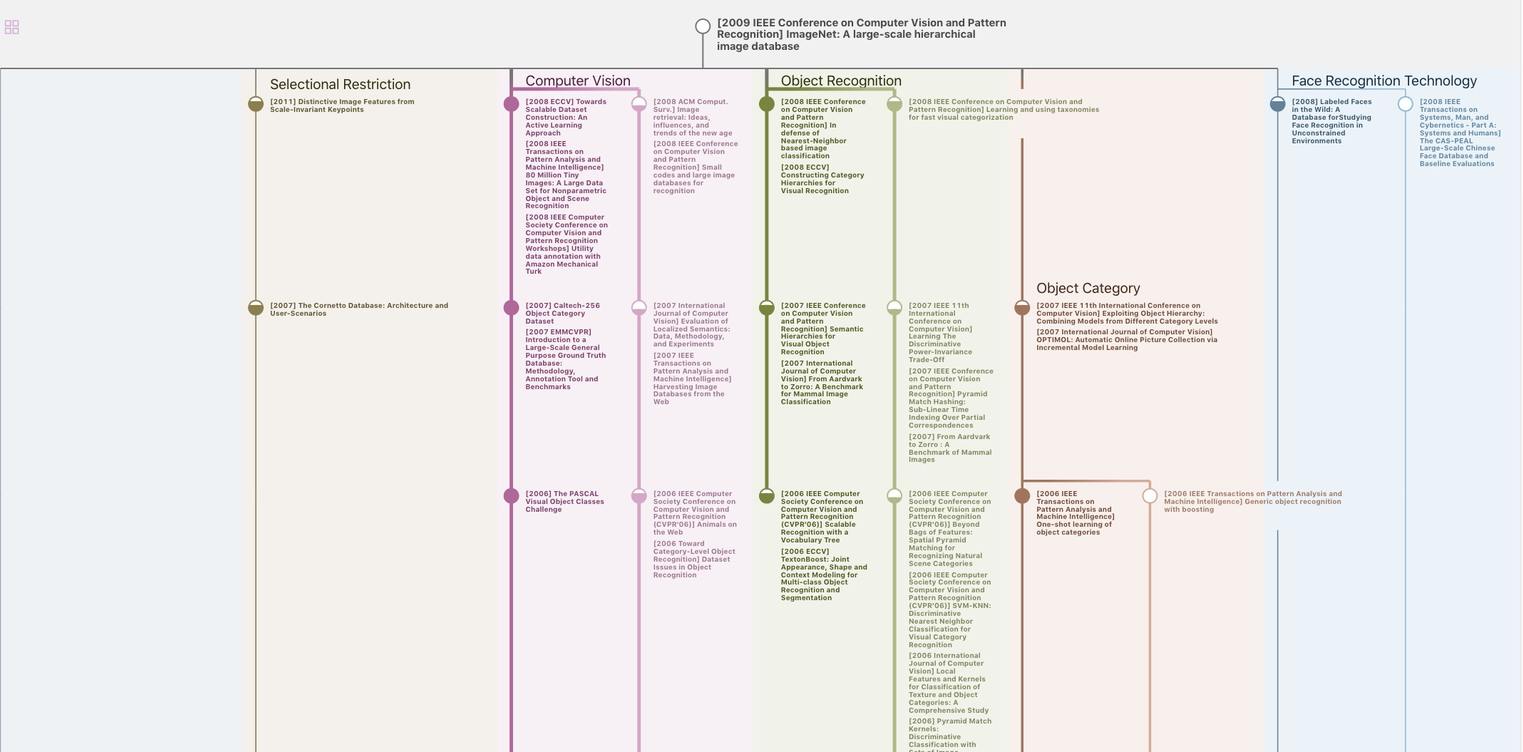
生成溯源树,研究论文发展脉络
Chat Paper
正在生成论文摘要