Wearable Sensor-Based Human Activity Recognition System Employing Bi-LSTM Algorithm
COMPUTER JOURNAL(2023)
摘要
Human activity recognition (HAR) systems employing wearable sensors are a promising area of research for tracking human activity. Recently, wearable devices such as smartwatches and sensors have been developed for activity recognition and monitoring. These systems aim to obtain the subject's state within his or her environment by exploiting heterogeneous sensors attached to the body. With the development of deep learning, new strategies have emerged to facilitate and solve the HAR problems. In this work, a deep multilayer bidirectional long-short memory (Bi-LSTM) architecture has been implemented to detect human activities. Instead of training a single model as in traditional LSTM methods, two models are presented in the Bi-LSTM scheme, one for learning the input data sequence and the other for learning the reverse sequence. Finally, a new novel postprocessing approach has been proposed based on windowing and voting in the last step to improve the average F1 score. Comprehensive investigations on the three publicly available datasets consisting of a different set of activities were used to evaluate the performance of the proposed framework. The empirical results of this paper on AReM, Mhealth and PAMAP2 datasets attained 95.46, 95.79 and 93.41% average F1 score, respectively. The results also revealed that selecting the window size and implementing the appropriate voting method has a significant effect on improving the average percentage of the F1 score.
更多查看译文
关键词
time series dataset,human activity recognition,Bi-LSTM algorithm,wearable sensors
AI 理解论文
溯源树
样例
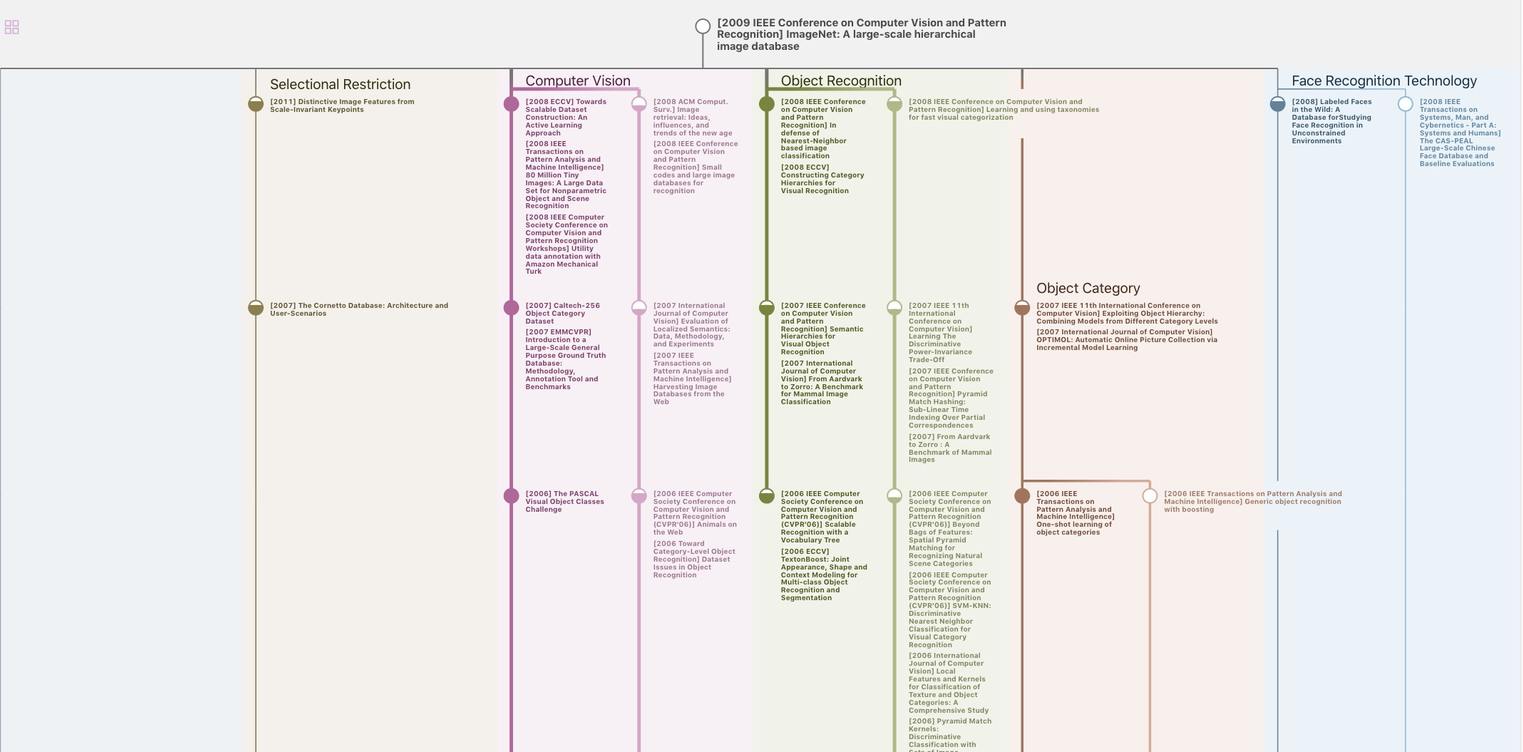
生成溯源树,研究论文发展脉络
Chat Paper
正在生成论文摘要