Machine learning boosting the discovery of porous metamaterials with an abnormal thermal transport property
APPLIED PHYSICS LETTERS(2023)
摘要
Normally, the introduction of porous structures into materials can tune their thermal conductivity, showing great applications in thermal management and thermoelectric energy harvesting. However, the ability of disorder changing the thermal conductivity of porous materials has seldom been explored. In this work, we show that an introduction of disorder into the macroscopic porous materials with a certain porosity can lead to a desired effective thermal conductivity over a large range, where an abnormal enhancement of similar to 7.9% and a normal reduction of similar to 44% at room temperature are predicted by the machine-learning-optimized algorithm. All of these theoretical calculation results are further verified by our experiments performed in the current work by using the steady-state thermal flux method. Moreover, when these periodic units are artificially connected, a structural anisotropy up to 40 is achieved, which can be further used to adjust the direction of the thermal flux in a well-controlled way. Our work provides an efficient and convenient approach for designing high-performance porous materials with specific thermal conductivity and high structural anisotropy for various applications in thermal management.
更多查看译文
关键词
porous metamaterials,learning
AI 理解论文
溯源树
样例
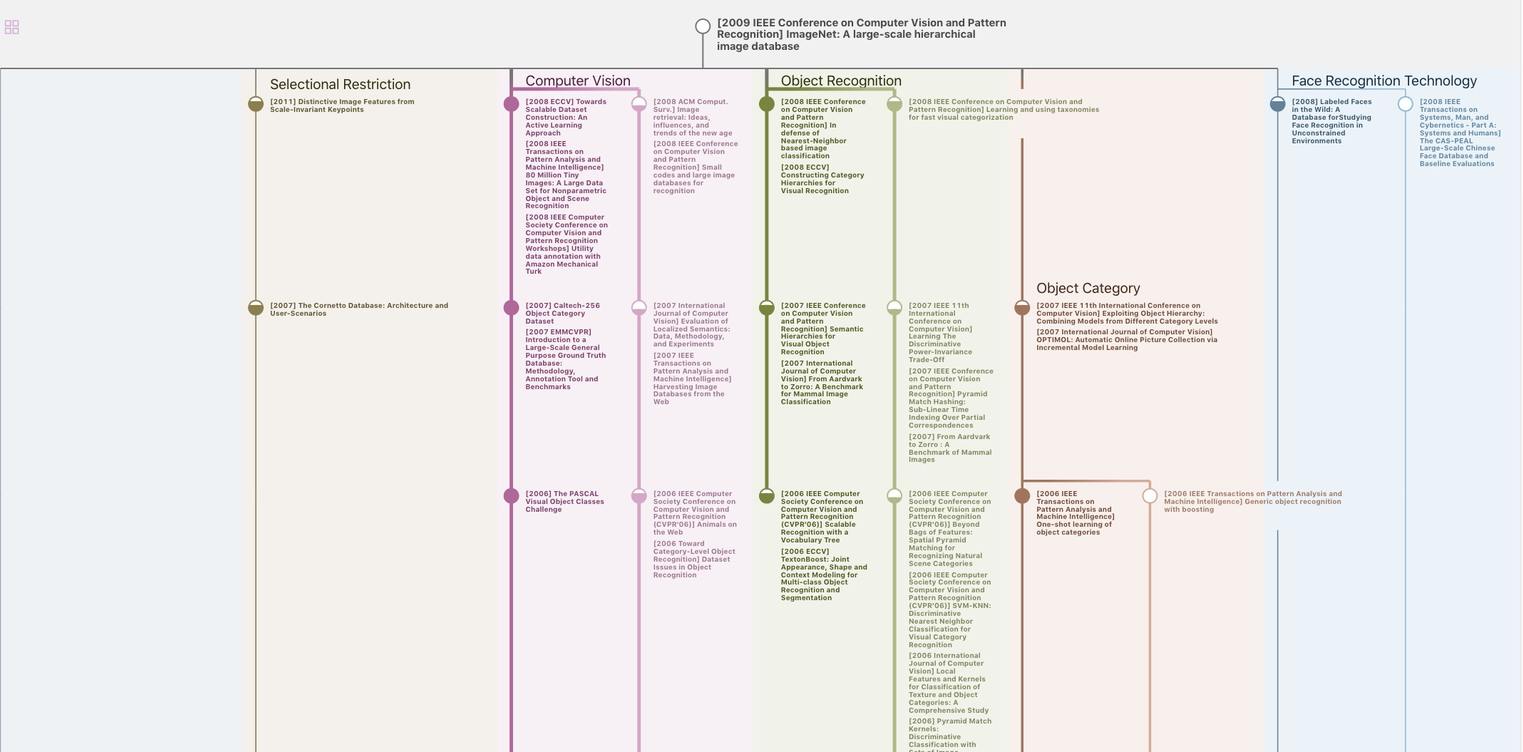
生成溯源树,研究论文发展脉络
Chat Paper
正在生成论文摘要