A Feedback-Enhanced Two-Stage Framework for judicial machine reading comprehension
ENGINEERING APPLICATIONS OF ARTIFICIAL INTELLIGENCE(2023)
摘要
Machine Reading Comprehension (MRC) is the task of teaching machines to understand and answer questions based on a given text. In the judicial domain, MRC has garnered attention as a novel strategy for addressing a variety of legal issues. Judicial MRC generally requires interpretability, meaning that the model should not only answer the question correctly but also provide supporting evidence sentences. A straightforward approach is to handle the two tasks separately, which ignores the relation between the two tasks and leads to the loss of annotated information. To make better use of the limited judicial MRC annotation data, we simulate the strategies used by humans in solving MRC problems and propose the Feedback-Enhanced Two-Stage Framework for Machine Reading Comprehension (FETSF-MRC). This framework consists of two cascaded modules: (a) a scanning module that identifies evidence sentences and (b) a detailed reading module that focuses on the evidence to identify candidate answers and provides feedback to the scanning module. Experiments on two Chinese judicial reading comprehension datasets (CJRC, CAIL2020) and an open-domain English dataset HotpotQA show that FETSF-MRC achieves superior performance compared to several baselines. FETSF-MRC outperforms the best baseline by 1.12% and 1.49% in joint F1 score on the CJRC and CAIL2020 datasets, respectively, and achieves 73.74% joint F1 score on the HotpotQA.
更多查看译文
关键词
Machine reading comprehension,Judicial question answering,Two stage training,Attention
AI 理解论文
溯源树
样例
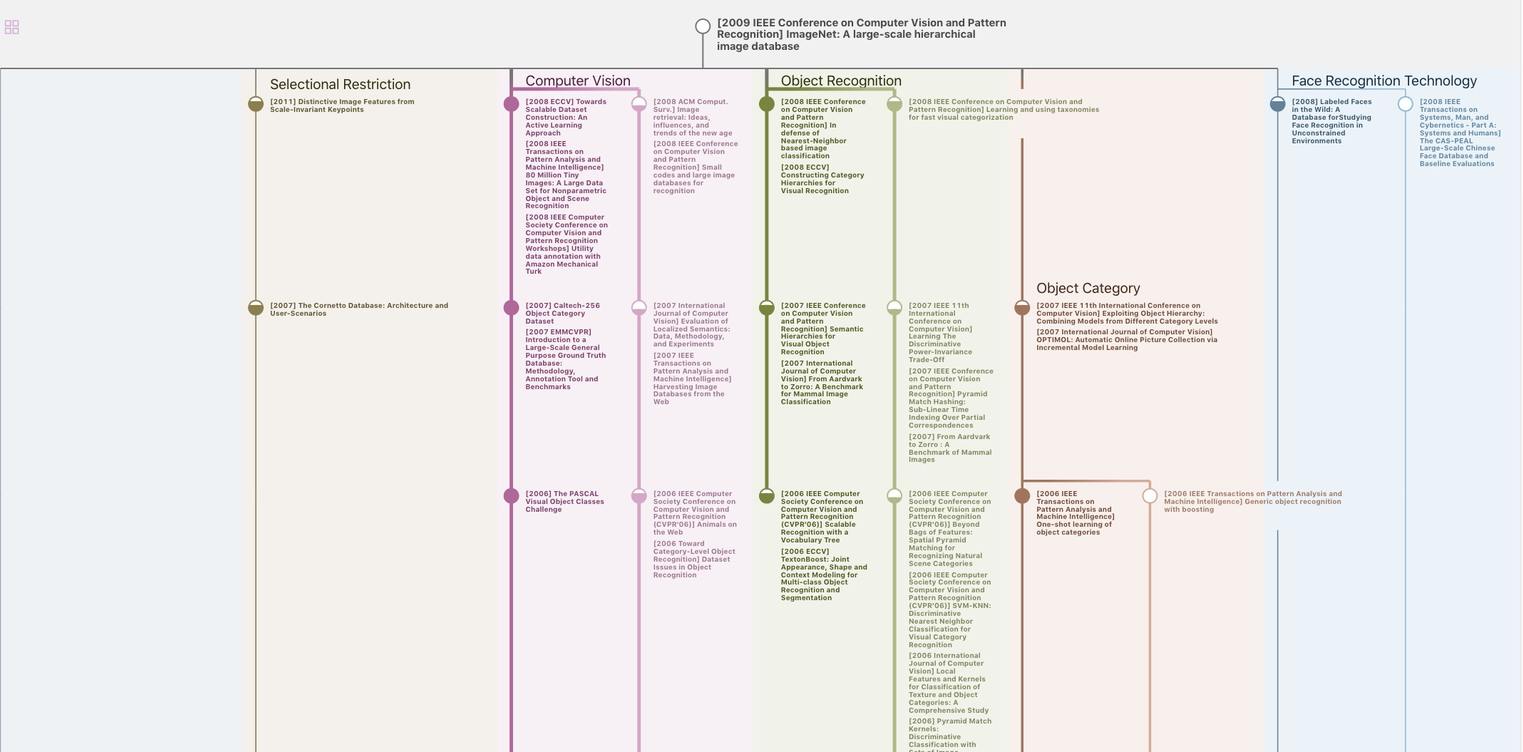
生成溯源树,研究论文发展脉络
Chat Paper
正在生成论文摘要