A Novel Click-Through Rate Prediction Model Based on Deep Feature Fusion Network
AATCC JOURNAL OF RESEARCH(2023)
摘要
Existing click-through rate prediction models employ both a shallow model and a deep neural model for better feature interaction. The former shallow model aims to extract explainable explicit features and the latter deep neural model aims to learn efficient implicit features. Deep neural network is a commonly used deep neural model, which can yield better performance with more neural layers. However, increasing the number of neural layers would lead to problems such as gradient vanishing, gradient explosion, and excessive parameters. In addition, the performance of a deep neural network will also decrease rapidly when it becomes too deep. In this article, we propose a novel click-through rate prediction model by improving the deep neural model part to alleviate the above problems of deep neural network-based models. This article proposes to utilize a dense deep neural network model to strengthen feature propagation, which takes the outputs of all previous layers as the input of the current layer, instead of only one previous layer being used in the deep neural network. In addition, we also utilize an advanced shallow model FmFM for better explicit features in this article, and explicit and implicit features are interacted in our model. Experiments on two data sets (Criteo and Avazu) show that the proposed click-through rate prediction model significantly outperforms existing classical models such as DeepFM, xDeepFM, and DeepLight models.
更多查看译文
关键词
Click-Through Rate Prediction,Deep Learning,Deep Neural Networks,Feature Fusion,FmFM
AI 理解论文
溯源树
样例
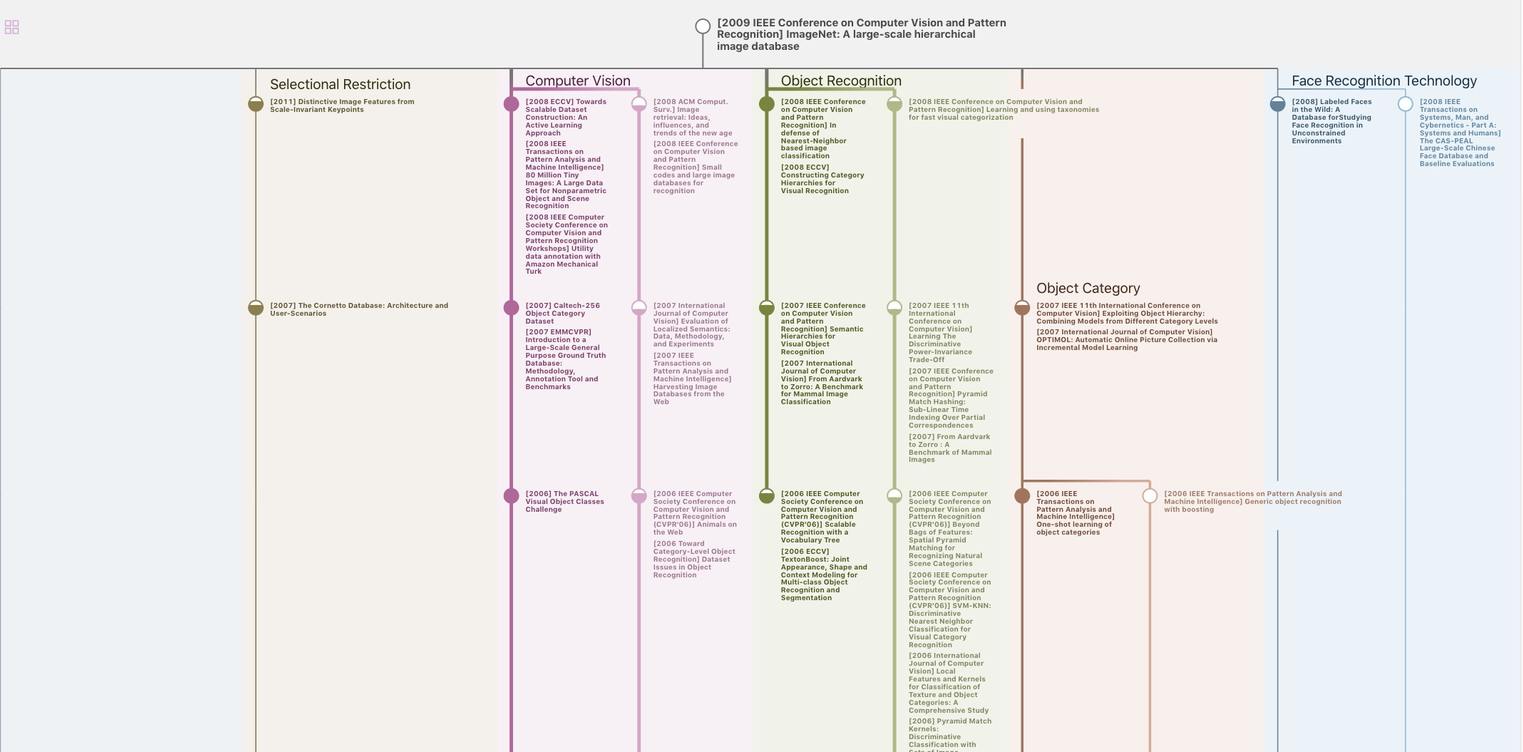
生成溯源树,研究论文发展脉络
Chat Paper
正在生成论文摘要