MDR-Net: Multiscale dense residual networks for liver image segmentation
IET IMAGE PROCESSING(2023)
摘要
Liver image segmentation is an attractive topic in the diagnosis and surgical planning of liver diseases. Although deep learning methods have significantly advanced liver segmentation, existing frameworks fail to clearly determine liver boundaries, especially in medical images where various organs have similar grey levels. In this paper, the authors design a multi-scale dense residual network (MDR-Net) for liver segmentation, which consists of two blocks: a liver segmentation network and an edge-aware network. In the segmentation network, the authors introduce a multi-scale residual pooling module combining channel attention (CA) mechanism and depth-wise separable convolution to accommodate liver scale variation. Furthermore, the authors employ an edge-aware loss network to refine edge information and enhance feature representation, which is beneficial to guide the network to iterate towards the ground truth. The authors' method achieves the best visualization results in qualitative evaluation. In addition, the authors' method achieves 96.189% on 3D-IRCADb and 96.889% on the CHAOS dataset in quantitative evaluation with respect to the dice index.
更多查看译文
关键词
biological organs,biological techniques,biological tissues,biomedical imaging,biomedical optical imaging,computer vision,feature extraction,feature selection
AI 理解论文
溯源树
样例
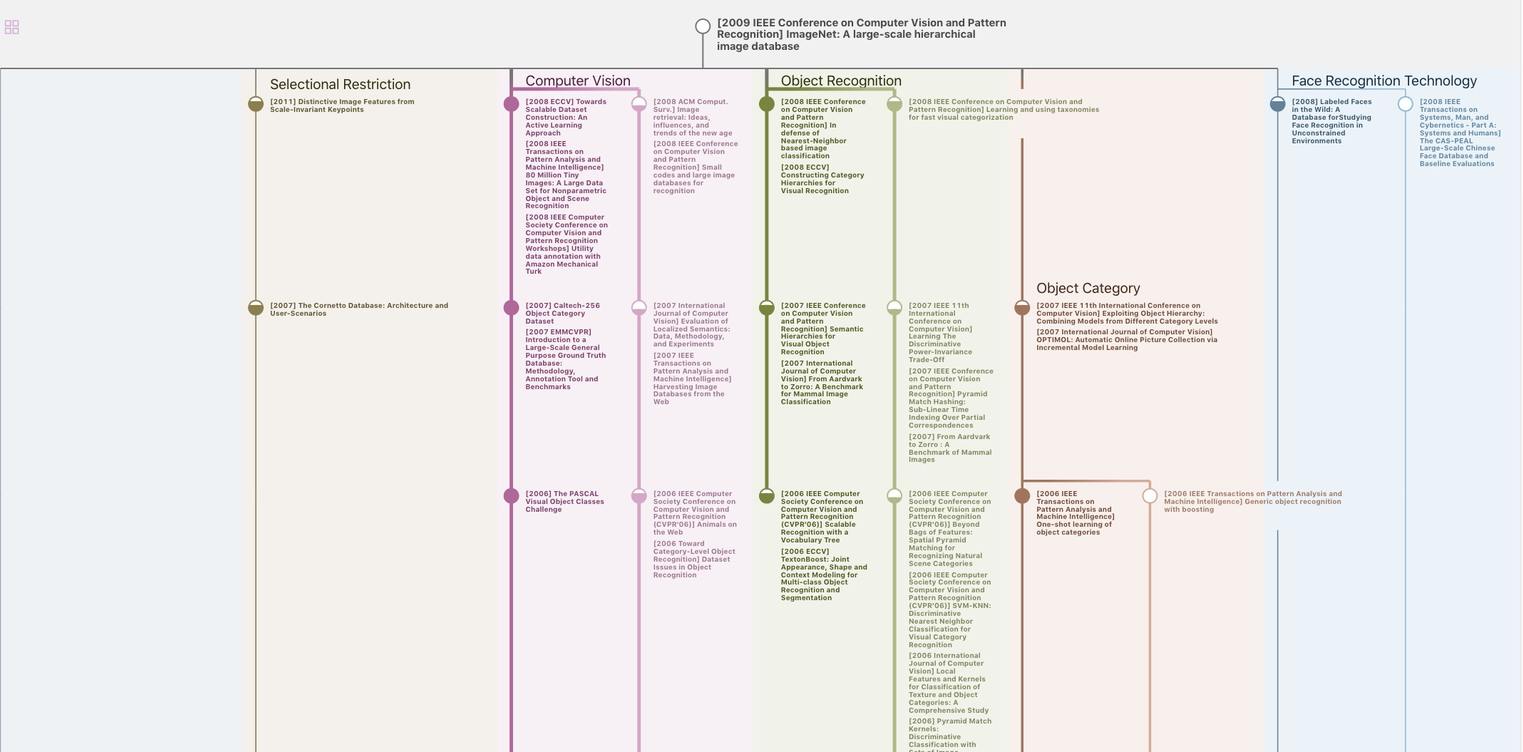
生成溯源树,研究论文发展脉络
Chat Paper
正在生成论文摘要