Spatial-temporal multi-feature fusion network for long short-term traffic prediction
EXPERT SYSTEMS WITH APPLICATIONS(2023)
摘要
Exploiting deep spatial-temporal features for traffic prediction has become growing widespread. Accurate traffic prediction is still challenging due to the complex spatial dependencies and time varying temporal dependencies, especially for long-term prediction tasks. Existing studies usually employ pre-defined spatial graphs or learned fixed adjacency graphs and design models to capture spatial and temporal features. However, the pre-defined or fixed graph cannot accurately model the complex hidden structure. In this paper, a novel deep learning framework called Spatial-Temporal Multi-Feature Fusion Network (STMFFN) is proposed to address these challenges. Specifically, a multi-scale attention module with temporal convolution is designed to capture the temporal dependencies from different scales. Then, a gated graph convolution module is proposed, which constructs adaptive adjacency matrices, and integrates graph convolution and graph aggregation modules to capture spatial dependencies from different ranges. Moreover, a multi-feature fusion layer is presented to fuse the extracted spatial and temporal dependencies by obtaining the attention vectors of temporal and spatial features. Experimental results on real-world datasets show a consistent improvement of 6%-9% over state-of-the-art baselines.
更多查看译文
关键词
Traffic prediction,Spatial-temporal data,Multi-feature fusion,Graph convolution network
AI 理解论文
溯源树
样例
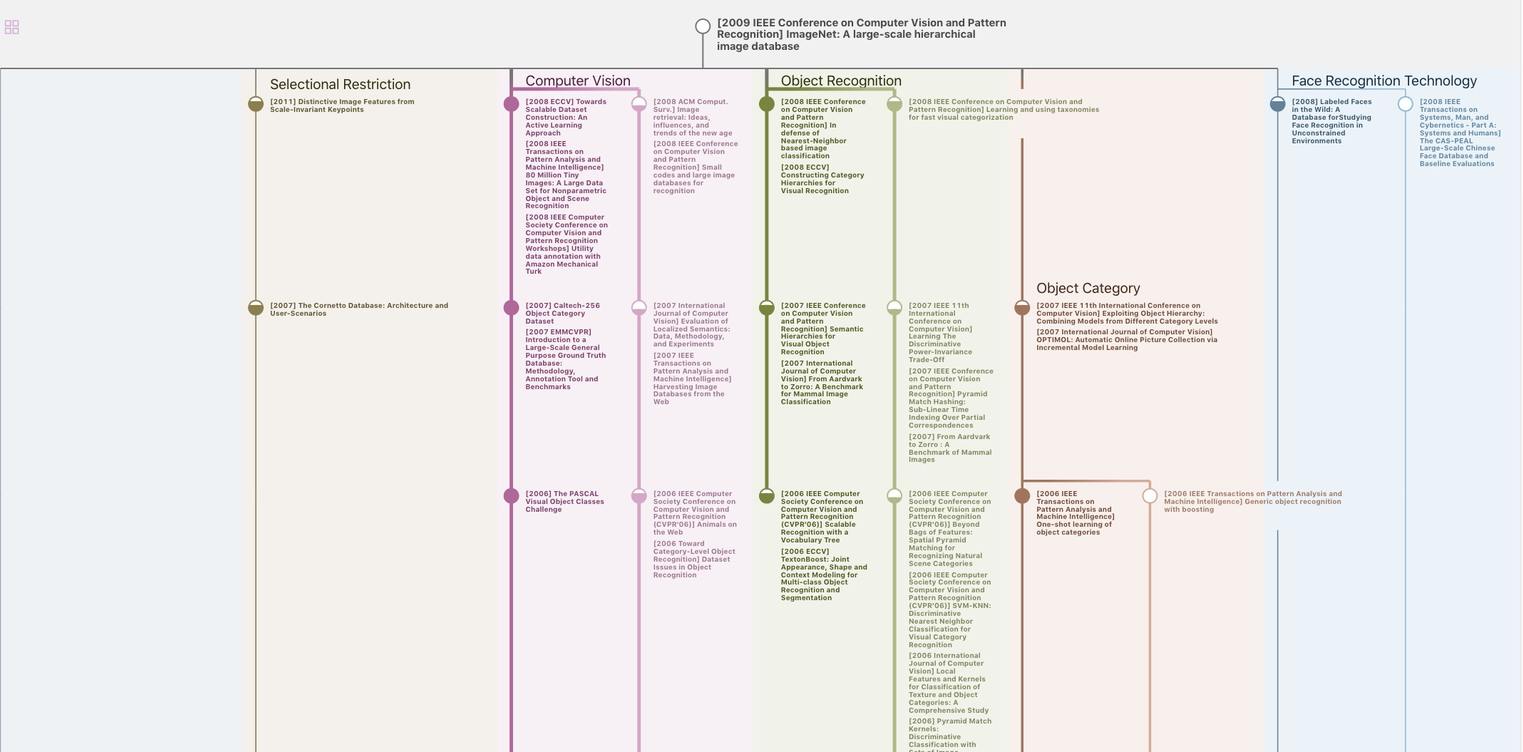
生成溯源树,研究论文发展脉络
Chat Paper
正在生成论文摘要