Data-based large-scale models provide a window into the organization of cortical computations
bioRxiv (Cold Spring Harbor Laboratory)(2023)
摘要
Understanding how the brain solves demanding computational tasks is one of the most exciting scientific challenges of our times. So far, recurrently connected artificial neural network models (RANNs) were primarily used to reverse-engineer brain computations. We show that it is now also feasible to reverse-engineer computations of detailed data-based large-scale models of cortical microcircuits. Furthermore, results of these analyses produce hypotheses that can readily be tested in biological experiments since they clarify from which neurons one needs to record and what type of information can be expected at specific time points during a trial. We apply this approach to a demanding visual processing task that has often been used in mouse experiments. Both the cortical microcircuit model and RANNs can solve this task as well as the mouse. But the resulting network dynamics matches only for the cortical microcircuit model experimental data on the sparseness of network activity and the impact of individual neurons on the network decision. Reverse-engineering of the computation in the cortical microcircuit model suggests that a particular subset of neurons causes a bifurcation of the network dynamics that triggers the network decision. Altogether, our results introduce a new type of neural network model for brain computations.
Teaser: Large-scale modeling on supercomputers provides new tools for understanding how the brain computes
### Competing Interest Statement
The authors have declared no competing interest.
更多查看译文
关键词
cortical,models,data-based,large-scale
AI 理解论文
溯源树
样例
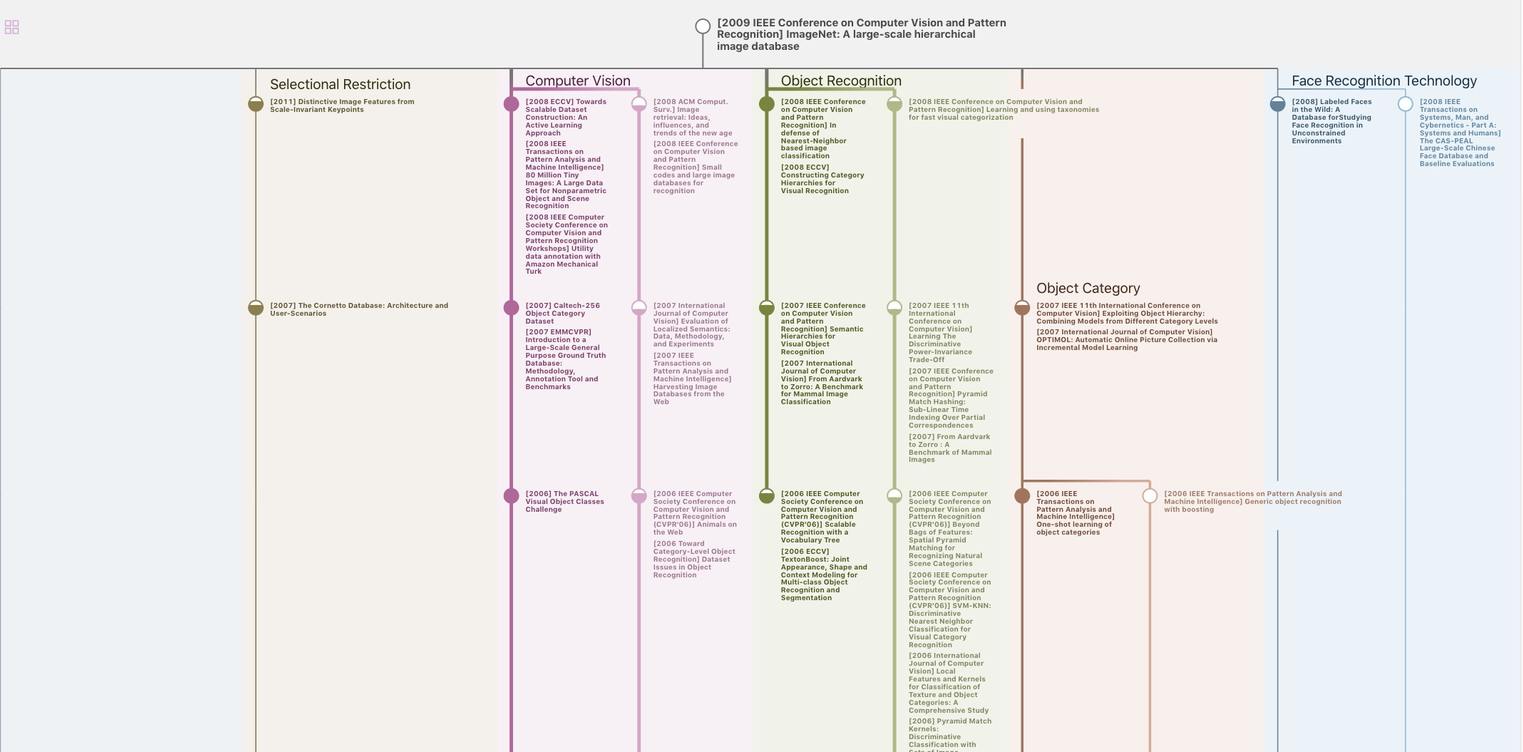
生成溯源树,研究论文发展脉络
Chat Paper
正在生成论文摘要