RNA-seq preprocessing and sample size considerations for gene network inference
bioRxiv (Cold Spring Harbor Laboratory)(2023)
摘要
Background Gene network inference (GNI) methods have the potential to reveal functional relationships between different genes and their products. Most GNI algorithms have been developed for microarray gene expression datasets and their application to RNA-seq data is relatively recent. As the characteristics of RNA-seq data are different from microarray data, it is an unanswered question what preprocessing methods for RNA-seq data should be applied prior to GNI to attain optimal performance, or what the required sample size for RNA-seq data is to obtain reliable GNI estimates.
Results We ran 9144 analysis of 7 different RNA-seq datasets to evaluate 300 different preprocessing combinations that include data transformations, normalizations and association estimators. We found that there was no single best performing preprocessing combination but that there were several good ones. The performance varied widely over various datasets, which emphasized the importance of choosing an appropriate preprocessing configuration before GNI. Two preprocessing combinations appeared promising in general: First, Log-2 TPM (transcript per million) with Variance-stabilizing transformation (VST) and Pearson Correlation Coefficient (PCC) association estimator. Second, raw RNA-seq count data with PCC. Along with these two, we also identified 18 other good preprocessing combinations. Any of these algorithms might perform best in different datasets. Therefore, the GNI performances of these approaches should be measured on any new dataset to select the best performing one for it. In terms of the required biological sample size of RNA-seq data, we found that between 30 to 85 samples were required to generate reliable GNI estimates.
Conclusions This study provides practical recommendations on default choices for data preprocessing prior to GNI analysis of RNA-seq data to obtain optimal performance results.
### Competing Interest Statement
The authors have declared no competing interest.
* BS
: B-spline
CPM
: counts per million
CS
: Chao-Shen
CT
: copula transformation
GNI
: Gene network inference
GRN
: Gene regulatory networks
GSEA
: Gene Set Enrichment Analysis ()
Log-2 or l2
: Logarithm with base 2
noCT
: no copula transformation
NoNorm or none
: No normalization (also denoted as nonnormalized )
QN or Q
: quantile normalization (QN)
PCC
: Pearson Correlation Coefficient
SCC
: Spearman Correlation Coefficient
PBG
: Pearson-based Gaussian
RLE
: relative log expression (RLE)
TMM
: The trimmed mean of M-values normalization
TPM
: transcript per million)
VST
: Variance-stabilizing transformation
更多查看译文
关键词
gene,sample size considerations,rna-seq
AI 理解论文
溯源树
样例
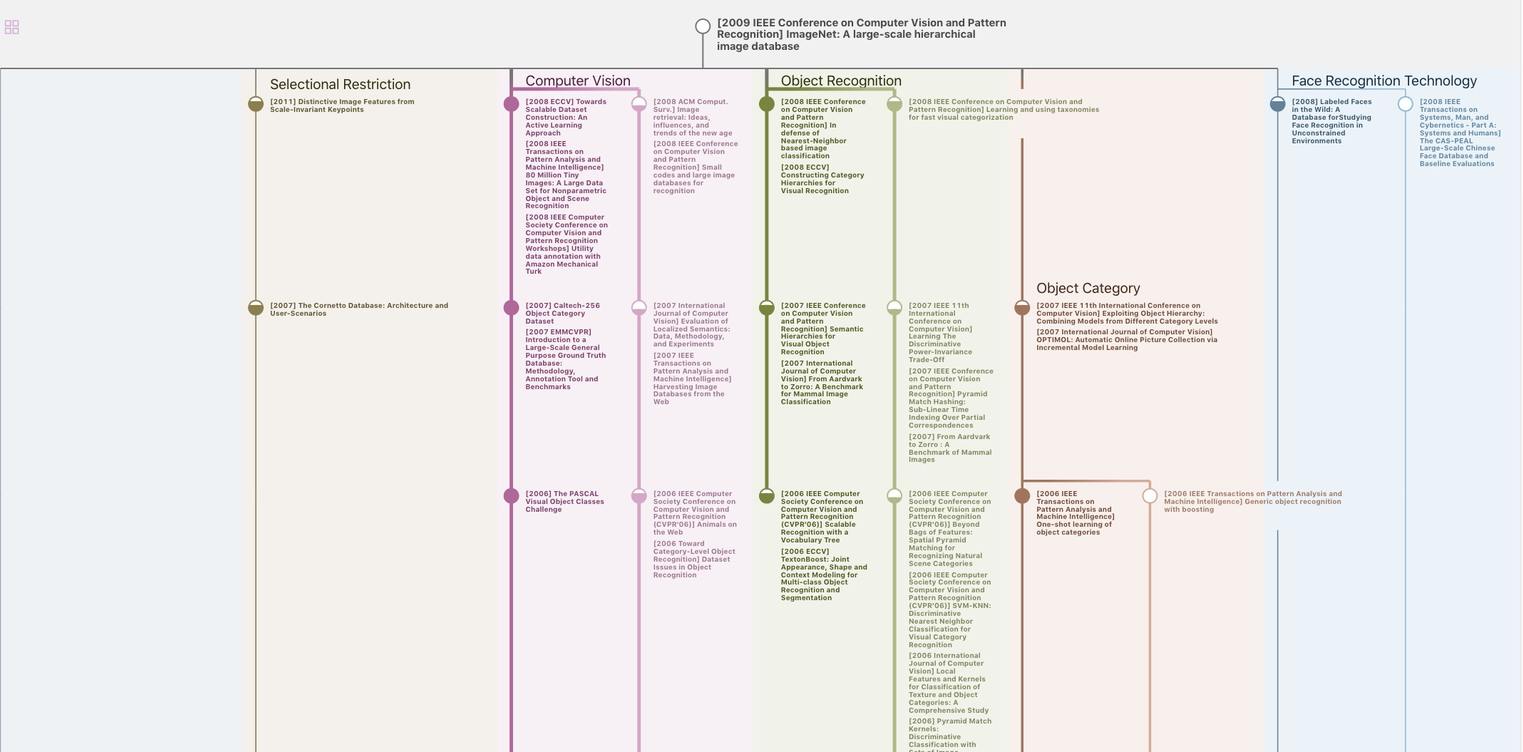
生成溯源树,研究论文发展脉络
Chat Paper
正在生成论文摘要