DM-Net: A Dual-Model Network for Automated Biomedical Image Diagnosis
Research in Computational Molecular Biology(2023)
摘要
Biomedical image segmentation is an essential task in the computer-aided diagnosis system. An encoder-decoder based on a shallow or deep convolutional neural network (DCNN) is an extensively used framework for biomedical image analysis. To study and rethink the effectiveness of compounding both the shallow and deep networks for the medical image segmentation task, we propose a dual-model CNN architecture, called DM-Net, for biomedical image segmentation. DM-Net is composed of a shallow CNN structure at its left, called L-Net and a deeper CNN structure at its right, named R-Net. The L-Net is proposed to encode low-level contextual information and the R-Net is presented to produce high-level semantic feature maps. Furthermore, a novel crossed-skip connection (CSC) strategy is proposed to transfer information between the two side networks mutually. Extensive experiments demonstrate that our method outperforms representative approaches on three public medical image datasets.
更多查看译文
关键词
Medical image segmentation,Crossed-skip c onnection,Contextual information encoding
AI 理解论文
溯源树
样例
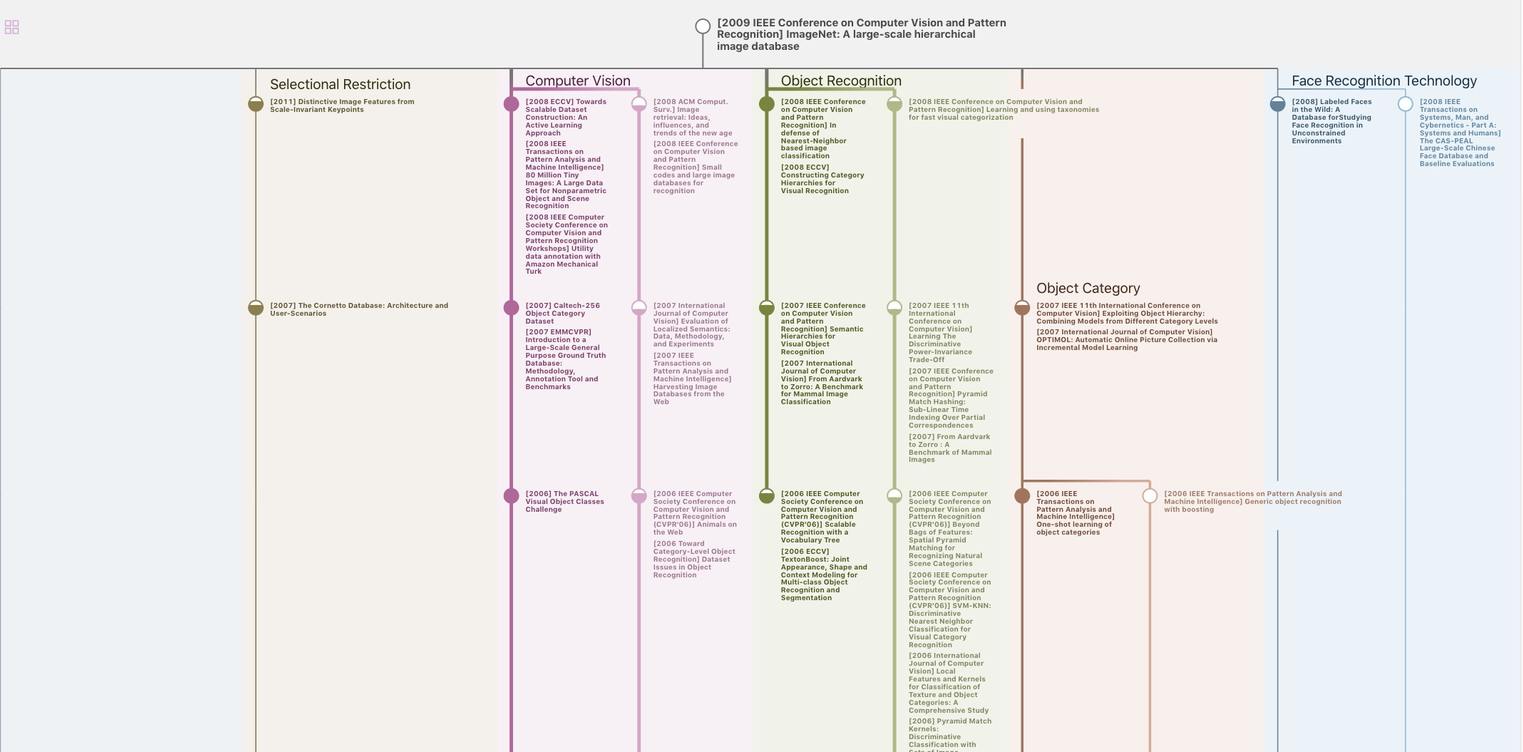
生成溯源树,研究论文发展脉络
Chat Paper
正在生成论文摘要